DOI: 10.31038/JCRM.2019252
Abstract
Objectives: Focal Segmental Glomerulosclerosis (FSGS) is a progressive glomerular disease. The pathogenesis of this disease, however, remains unclear. Here, we attempted to identify key candidate genes in FSGS through stringent bioinformatic analysis.
Methods: We systematically searched the Gene Expression Omnibus database for gene expression microarrays derived from human glomeruli tissues with FSGS. First, we identified differentially expressed genes (DEGs) by using the Limma package in R. Then, we subjected these DEGs to Gene Ontology (GO) analysis for further analysis. Finally, we constructed Protein–Protein Interaction networks (PPI) through four different methods and performed intersection analysis to further refine our results.
Results: A total of 627 DEGs were identified between the FSGS and control groups, among which 534 were up-regulated and 93 were down-regulated. GO analysis revealed that the DEGs were enriched in mRNA processing, cell adhesion molecule binding, and cadherin binding. Furthermore, via PPI, 7 DEGs overlapped in the four groups constructed through different analytical approaches. We also validated the overlapped 7 hub genes in in vitro experiments, including RBM5 and HNRNPF, with potentially important roles in the development of FSGS.
Conclusion: Our study provides a valuable resource for novel biomarkers and therapeutic targets for FSGS.
Keywords
Focal segmental glomerulosclerosis; Bioinformatic analysis; RBM5; HNRNPF
Introduction
Focal Segmental Glomerulosclerosis (FSGS) is a primary glomerular disease that manifests with heavy proteinuria [1]. It is the leading cause of the development of end-stage renal disease. Typically, FSGS lesions present a segmental manifestation that includes parietal cell migration, hyaline deposition, capillary collapse, and intracapillary thrombi. Recent studies suggest that podocyte injury may play a key role in FSGS lesions [2]. Injury and loss of podocytes result in foot process effacement and protein loss [3]. However, the pathogenesis of FSGS remains unclear, and the present diagnostic and therapeutic methods for this disease remain inadequate. Oxidative stress has been implicated in the development and progression of this FSGS [4,5]. Nuclear factor E2-related factor 2 (Nrf2) is a transcription factor that can potently induce the production of numerous antioxidants and prevent the generation of oxidative stress in renal fibrosis and inflammation [6–8]. Furthermore, apoptosis and the renin–angiotensin system are strongly involved in FSGS-related injury
[9–12]. Nevertheless, a considerable amount of important FSGS genes remain unidentified given the lack of global analysis.
With the development of bioinformatic analysis technology, gene expression profiling analysis has been increasingly used to explore molecular mechanisms and identify novel biomarkers in various diseases [13–16]. Bioinformatic analysis is mainly used to predict novel diagnostic biomarkers and therapeutic targets associated with tumors, such as bladder cancer [17], meningioma [18], and hepatocellular carcinoma [19]. The application of bioinformatic analysis in renal diseases, such as renal cell carcinoma [20], lupus nephritis [21], IgA nephropathy [22], and chronic kidney disease [23], has begun to develop gradually. However, up to now, no study has subjected FSGS to bioinformatic analysis. Thus, exploring the underlying crucial genes and effective therapeutic targets for FSGS through bioinformatic analysis is necessary.
In this study, we downloaded the gene expression profile datasets of FSGS from the Gene Expression Omnibus (GEO) database and investigated Differentially Expressed Genes (DEGs) between FSGS and control samples by using the Limma package in R. We performed Gene Ontology (GO) enrichment analysis for all DEGs. In addition, we constructed protein–protein interaction (PPI) networks, identified novel hub genes, and validated them in in vitro experiments. Our study aimed to predict novel diagnostic biomarkers and potential therapeutic targets for FSGS.
Materials and Methods
Data collection
Gene expression profiles were retrieved from NCBI’s GEO database (http://www.ncbi.nlm.nih.gov/geo/) by using the key words “focal segmental glomerulosclerosis” with the following criteria: 1) the study type is expression profiling by array, 2) the attribute name is tissue, 3) the organism of interest is Homo sapiens, and 4) the platform used is the Affymetrix Human Genome U133A Array. Ultimately, on the basis of the above criteria, we selected dataset GSE47185 of FSGS. Original CEL files were used for further bioinformatic analysis.
Data preprocessing
CEL files were normalized and converted to expression profiles by using the Affy package of R [24]. In brief, the original data were read using the Affy Bioconductor package and preprocessed for normalization through the robust multiarray analysis method, which includes background correction, normalization, expression calculation and batch effects removal. After obtaining the gene expression value, genes were annotated with the hgu133A.db and annotate software packages.
DEG analysis
The Limma package of R [25] was used to analyze DEGs after preprocessing. The linear fit method, Bayesian analysis, and t-test algorithm were used to calculate the P and FC values. DEGs were screened by setting a cut-off value of |log 2 Fold Change (FC)| > 1 and P < 0.05. The ggplot2 software package was used to visualize results. Moreover, to identify and visualize the DEGs between FSGS and normal samples, we generated a heat map of the top 10% DEGs by using the heatmap package (Version 1.0.8).
GO enrichment analysis for DEGs
The GO consortium includes three independent branches: Biological Process (BP), Cellular Component (CC), and Molecular Function (MF). In this study, we subjected the identified DEGs to GO enrichment analysis by using R and the clusterProfiler package [26]. P < 0.05 was used as the threshold for the identification of significant GO terms.
PPI Network Construction
To explore the relationships among the top 30% DEGs, we used the online tool STRING (Search Tool for the Retrieval of Interacting Genes/Proteins; http://string.embl.de/) database for the construction of PPI networks. The minimum required interaction score of 0.4 was used as the significant cut-off threshold. Then, the obtained PPI interaction networks were visualized by using Cytoscape software (version 6.3).
Hub-gene screening
On the basis of the STRING results, we introduced the four methods Degree, EPC, Maximal Clique Centrality (MCC), and DMNC [27] to rank the importance of nodes in the PPI networks and to further identify hub genes from the top 30% DEGs. Nodes with high centrality scores were considered hub genes. We applied the R package Venn diagram (version1.6.17, https://cran.r-project.org/web/packages/VennDiagram/) [28] to identify overlapping DEGs among these hub genes.
Degree (Deg(v) = |N(v)|) is a computing tool in Cytoscape software [29]. The default filter “in and out” was between 7 and 42 in the present study.
MCC is a topological analysis method in CytoHubba [27]. Given a node v, the MCC of v is defined as MCC(v) = ∑CÎS(v)(|C|−1)!.
Maximum Neighborhood Component (MNC) is another computing tool in cytoHubba. MNC(v) = |V(MC(v))|, where MC(v) is a maximum connected component of G[N(v)], and G[N(v)] is the induced subgraph of G by N(v). On the basis of MNC, Lin et al. proposed that DMNC(v) = |E(MC(v))|/ |V(MC(v))| ε , where ε = 1.7.
Cell culture
Conditionally immortalized human podocytes (LY893) were kindly provided by Dr. Lan Ni and Moin Saleem(Bristol, U.K.). Podocytes were cultured in RPMI 1640 medium(Gibco)supplemented with 10% Fetal Bovine Serum (Gibco) and 1% Insulin-Transferrin-Selenium(Invitrogen) at 33°C under 5% CO2 for propagation, then were thermo switched to 37°C under 5% CO2 when at 60% confluency for differentiation. The differentiated podocytes were incubated with adriamycin to construct an in vitro model for FSGS [30].
Quantitative Real-Time PCR
Total RNA was extracted using the RNeasy Plus Mini Kit (BioTeke RP1202) in accordance with the manufacturer’s instructions. The cDNA was obtained by reverse transcription, amplified and detected using a SYBR Green Supermix kit (Takara). Then, a BIO-RAD CFX-96 Real-Time PCR system (Bio-Rad) was used for PCR analysis under the following conditions: 95 °C for 3 min, followed by 40 cycles of 95 °C for 10 s and 51 °C for 30 s. The primer sequences used for PCR are listed in Table 1. Statistical differences were determined by Student’s t-test using R “ggpubr” package(version 0.1.8, https://CRAN.R-project.org/package=ggpubr).
Table 1. Primer sequences for RT-PCR.
Gene
|
Forward primer
|
Reverse primer
|
FUS
|
5’ GCAGGAGTTTGTGGAGTG 3’
|
5’ TGAGTACAGGCAGGATGTG 3’
|
DHX15
|
5’ CTTTACAAGCAGGGACTA 3’
|
5’ TCAAGAACAGTAGAGGGAT 3’
|
PRPF31
|
5’ TGTCGGGCTTCTCGTCTA 3’
|
5’ CACCTTCCCTTCTGTGCTCT 3’
|
PQBP1
|
5’ CAAGAAGGCAGTAAGCCGAAAG 3’
|
5’ TGTGGTGTCAGCGCCAGTC 3’
|
RBM5
|
5’ GGTGCGAAATGGAGATGA 3’
|
5’ AGAGTTGCTGGTGCCTGA 3’
|
HNRNPR
|
5’ AAGTCCCACAGAACCGAGAT 3’
|
5’ AACCCTGAGAAGAACTGAACAA 3’
|
TRA2B
|
5’ CACATACGCCAACACCAG 3’
|
5’ TCCTCCACCTCCTCCTCT 3’
|
GAPDH
|
5’ CTTTGGTATCGTGGAAGGACTC 3’
|
5’ GTAGAGGCAGGGATGATGTTCT 3’
|
Results
DEGs identification
This dataset GSE47185 contains the mRNA expression profiles of 13 FSGS samples and 14 control samples (normal tissue of renal tumor excision). Under the threshold of |log 2 fold change (FC)| > 1 and adj.P value < 0.05, 627 DEGs were identified between the FSGS and control groups. These DEGs included 534 up-regulated and 93 down-regulated DEGs. The results of expression-level analysis are presented as a volcano plot in Fig. 1A. As shown in Table 2, RPS4Y1, PLPP3, DDX3Y, SART3, TCF4, TROVE2, IQGAP1, MBP, CALD1, and RBFOX2 are the 10 most significantly up-regulated genes, whereas CYP4A11, FOSB, EGR1, G6PC, ALB, CTSZ, PPP3R1, XIST, PCK1, and HPGD are the 10 most significantly down-regulated genes. The more information of all DEGs is listed in supplementary Table 1.
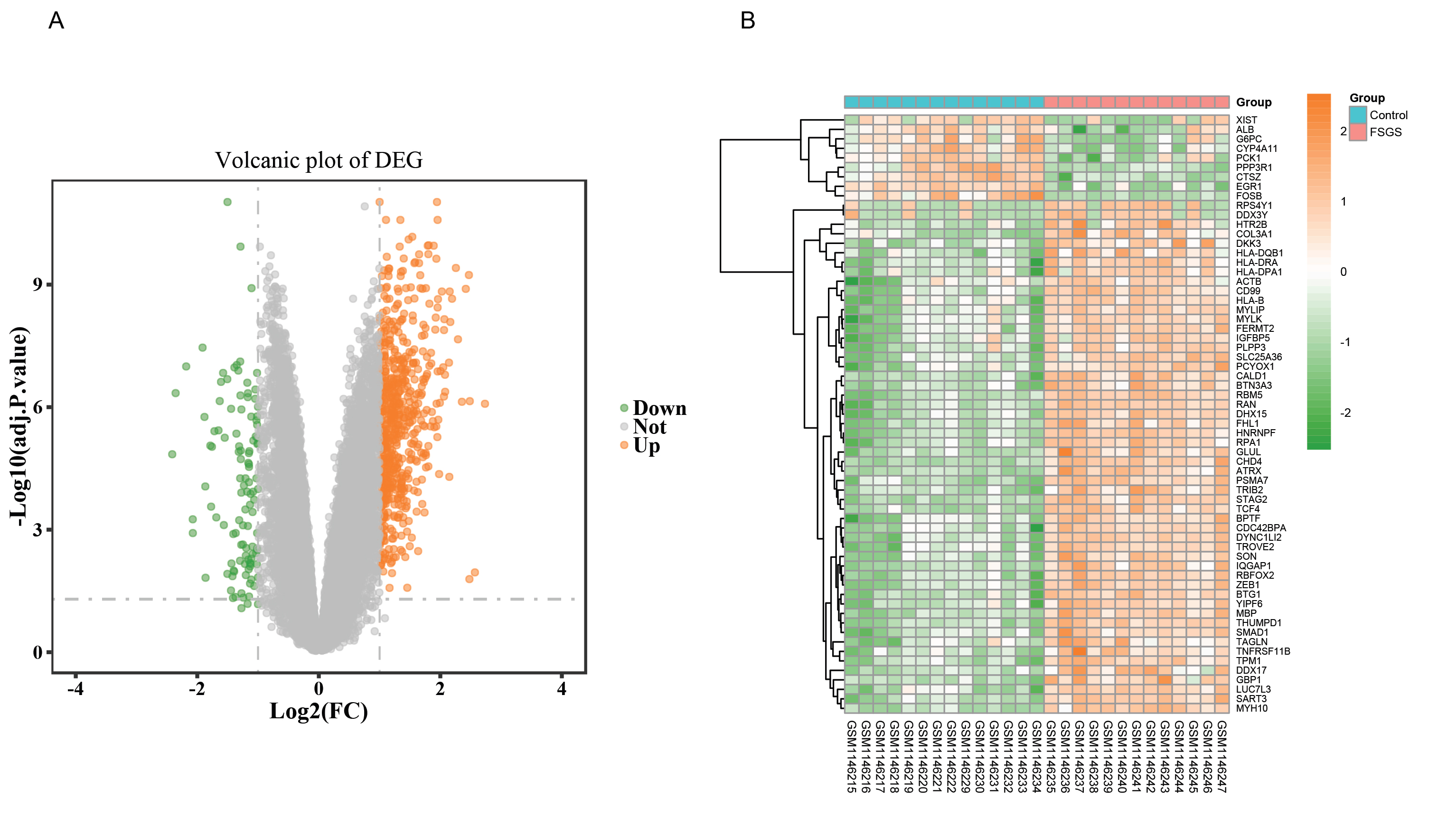
Figure 1.
Visualization of DEGs
A, The volcano plot of differentially expressed genes between FSGS and healthy tissues. The red plots represent up-regulated genes, green plots represent down-regulated genes, while grey plots represent non-significant genes. The volcano plot was constructed using the ggplot2 package of the R language; B, A heatmap of the top 10% DEGs. The horizontal axis denotes the different samples, and the vertical axis denotes different DEGs.blue, normal samples; red, FSGS samples; purple clusters represent up-regulated DEGs and green clusters represent downregulated DEGs. Color key represents the Z-score based on the Gene expression value.
Table 2. The Most Significant 10 Up-Regulated Genes And Down-Regulated Genes.
Gene Symbols
|
Log FC
|
Average Expression level
|
Adj.P. Value
|
RPS4Y1
|
2.569009
|
9.570289
|
1.11E-02
|
PLPP3
|
2.485089
|
9.251834
|
7.13E-07
|
DDX3Y
|
2.478844
|
7.003028
|
1.62E-02
|
SART3
|
2.470577
|
7.660357
|
5.82E-10
|
TCF4
|
2.41899
|
7.335133
|
1.27E-09
|
TROVE2
|
2.355365
|
9.457713
|
7.39E-07
|
IQGAP1
|
2.290646
|
8.145384
|
2.19E-08
|
MBP
|
2.255968
|
7.587945
|
3.91E-10
|
CALD1
|
2.192563
|
8.074506
|
2.21E-09
|
RBFOX2
|
2.164463
|
8.698168
|
8.22E-09
|
CYP4A11
|
-2.41227
|
9.128665
|
1.42E-05
|
FOSB
|
-2.35626
|
8.074382
|
4.53E-07
|
EGR1
|
-2.18278
|
10.28014
|
1.01E-07
|
G6PC
|
-2.07454
|
6.566343
|
5.57E-04
|
ALB
|
-2.0735
|
9.571928
|
1.21E-03
|
CTSZ
|
-1.91281
|
8.256097
|
3.49E-08
|
PPP3R1
|
-1.88153
|
7.627366
|
1.74E-06
|
XIST
|
-1.86386
|
7.615666
|
1.50E-02
|
PCK1
|
-1.7723
|
10.99439
|
2.73E-04
|
HPGD
|
-1.7174
|
10.07267
|
3.87E-06
|
The heatmaps of the top 10% DEGs are shown in Fig. 1B. The data are presented in a matrix format, in which rows represent individual genes, and columns represent individual samples. The purple and green colors indicate up-regulated and down-regulated genes, respectively. The hierarchy cluster analysis indicated that FSGS and control groups could be distinguished from each other on the basis of their different expression patterns.
Functional enrichment analysis
To reveal the biological functions of DEGs, we used the clusterProfiler package for GO analysis. We set adj.P value < 0.01 to identify significantly enriched GO terms. The top eight GO terms for the DEGs enriched in the BP, CC, and MF are shown in Figure 2. The DEGs were mainly involved in GO terms that included mRNA processing, regulation of mRNA metabolic process, antigen processing and presentation of exogenous antigen, focal adhesion, cell adhesion molecule binding, cadherin binding, and actin binding. Among these terms, the MFs related to focal adhesion (GO:0005925) [31,32], cell adhesion molecule (GO:0050839) [33,34], cadherin (GO:0045296) [35], and actin binding (GO:0003779) [36] were all confirmed to be involved in glomerulosclerosis. Detailed information on the top eight GO terms is shown in Table 3.
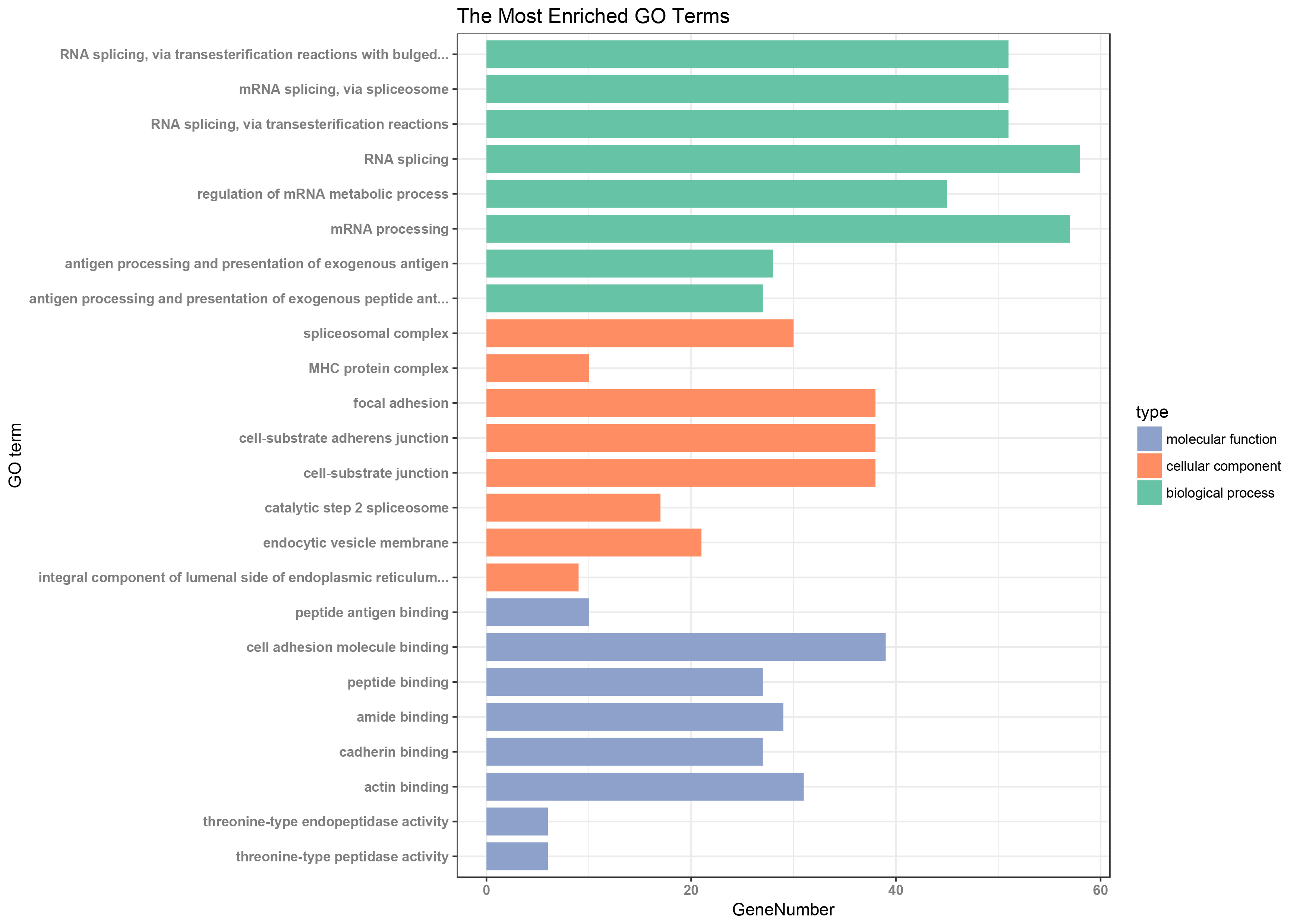
Figure 2. The 8 most significant enriched GO terms of DEGs
The adj.P value< 0.01 was used as the threshold for the identification of significant GO terms. The Gene ontology covers the biological process, cellular component, and molecular function.The horizontal axis represents the gene counts, the vertical axis represents GO terms.Green column graphs represent biological process(BP) GO term; orange column graphs represent cellular component(CC) GO term; and blue column graphs represent molecular function (MF) GO term.
Table 3. The Most Significantly Enriched GO Terms In BP ,CC and MF.
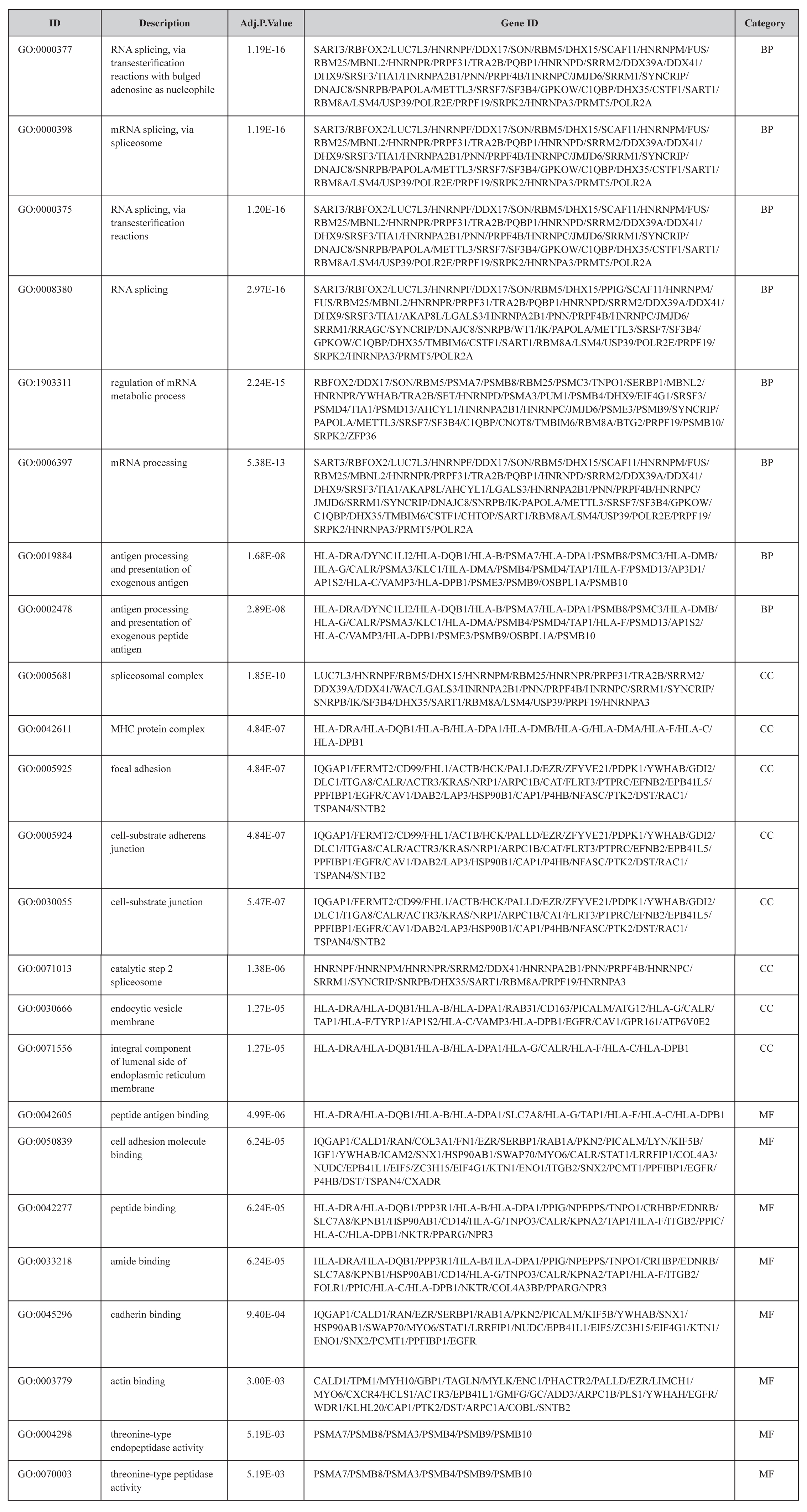
PPI Network Construction and Hub-Gene Screening
The major part of the constructed PPI network is presented in Figure 3A. To further reduce the scope for analysis, we analyzed the PPI network by using the four analysis methods in CytoHubba based on the R package Venn diagram. We used the top ranked 20 DEGs to identify seven overlapping hub genes screened through the four CytoHubba methods (Degree, EPC, MCC, and DMNC) in cytoscape software (Figure 3B–F). The seven overlapping hub genes included FUS, DHX15, PRPF31, PQBP1, RBM5, HNRNPR, and TRA2B. Strikingly, the identified hub DEGs in our study have never been reported in literature related to FSGS. In addition, these genes simultaneously ranked to the high position by the four different CytoHubba methods suggests they may play important roles in the development of FSGS.
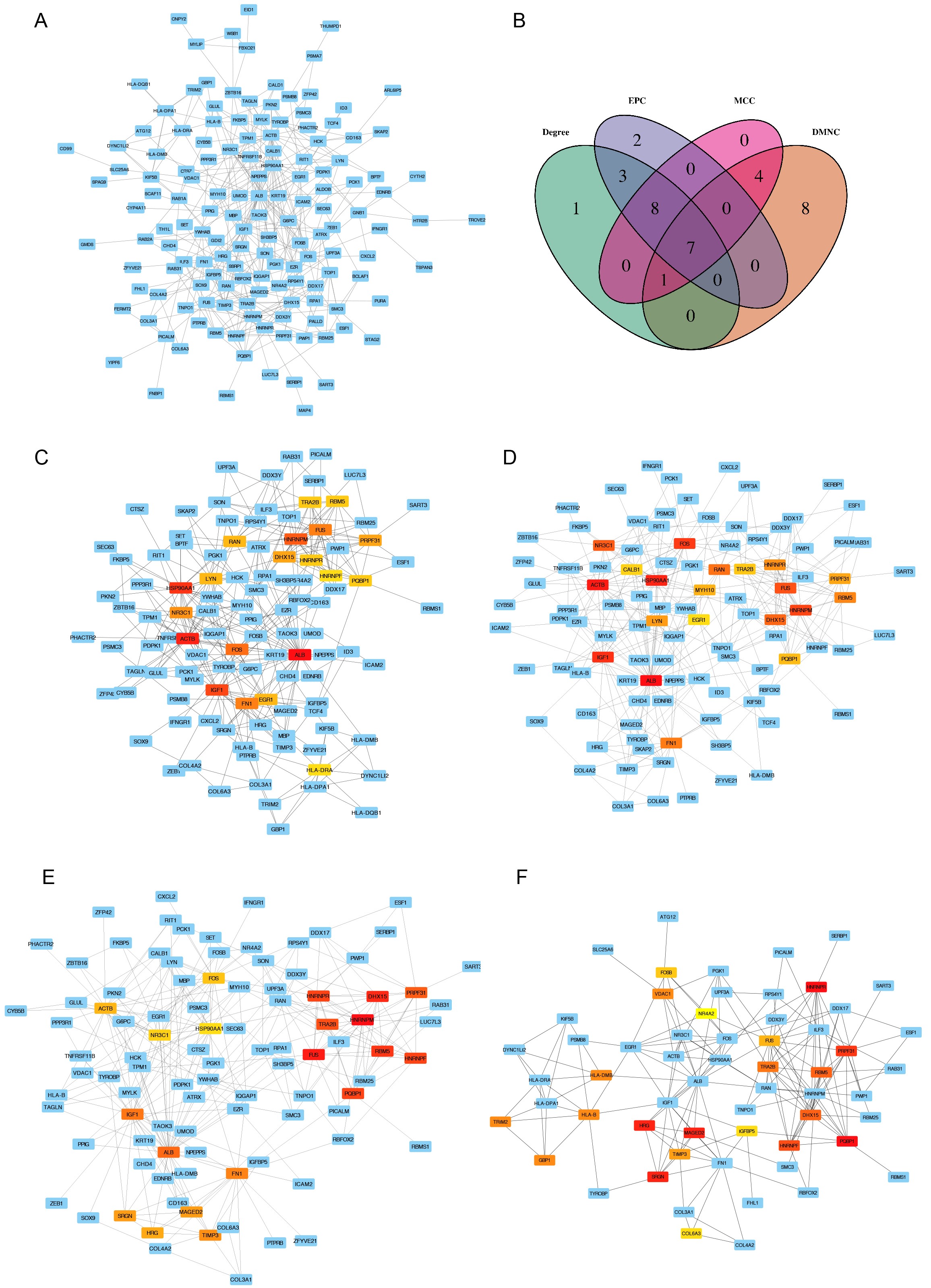
Figure 3: Protein-protein interaction (PPI) networks of DEGs and screening of hub genes
A: The major part of PPI network; B: Venn diagram of differentially expressed genes based on four screening methods including “Degree”, “EPC”, “MCC”, and “DMNC”; C: PPI of DEGs screened by the method “Degree” in Cytohubba; D: PPI of DEGs screened by the method “EPC” in Cytohubba; E: PPI of DEGs screened by the method “MCC” in Cytohubba; F: PPI of DEGs screened by the method “DMNC” in Cytohubba. The depth of red represents the rank of the hub genes.
Gene Expression Validation in In -Vitro Experiments
We validated the 7 top ranked hub genes expression in the FSGS model in vitro (Figure 4). Quantitative real-time PCR indicated that the mRNA levels of FUS, DHX15, PQBP1, RBM5, and HNRNPR were up-regulated after they were stimulated by adriamycin (ADR). The changes in PRPF31 and TRA2B mRNA were statistically insignificant. Except for PRPF31 and TRA2B, the changes in all of the other gene expression levels were consistent with the bioinformatic analysis results (Supplementary Table 1), with the accordance rate reaching 70% approximately.
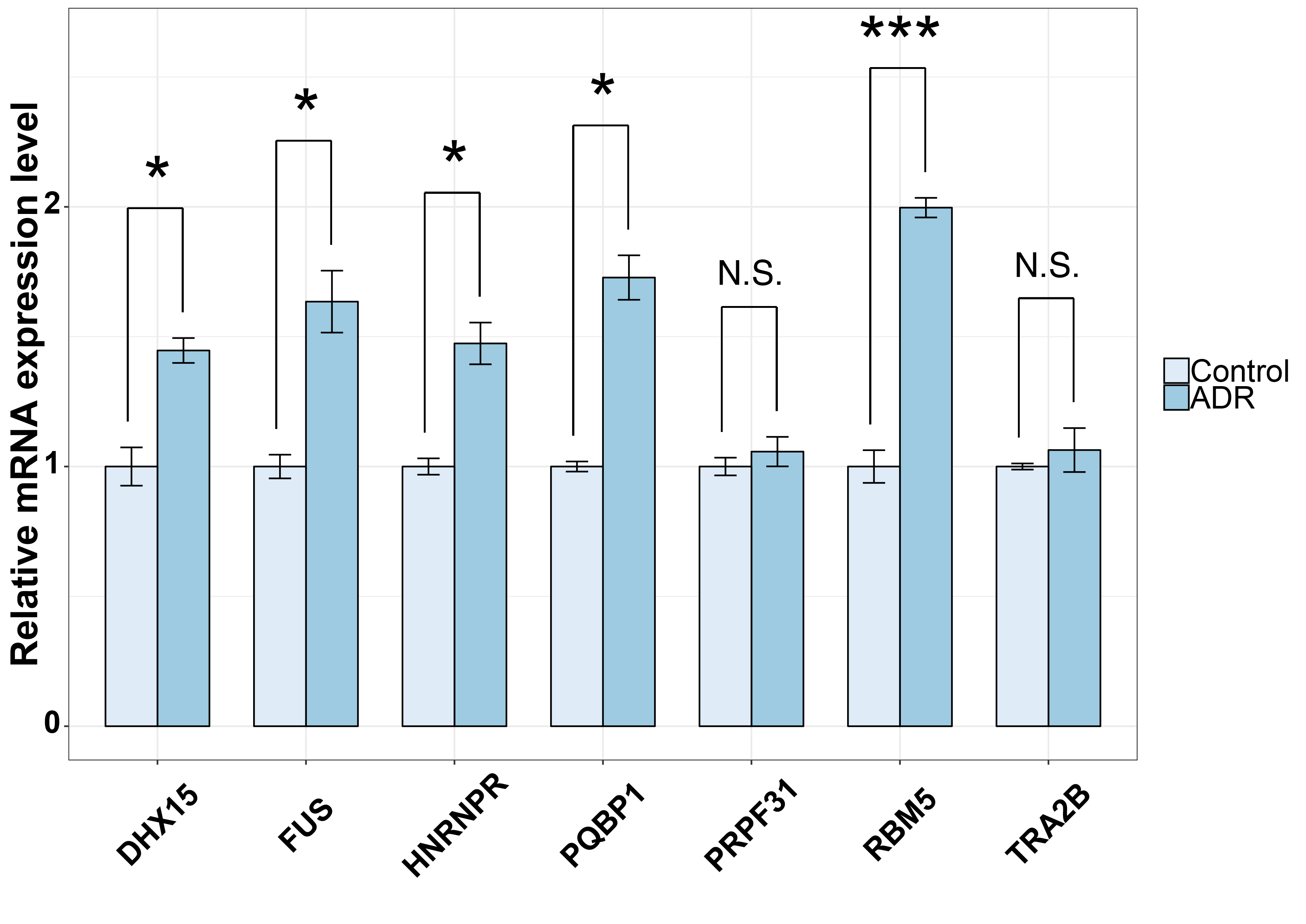
Figure 4. In vitro validation for the novel hub genes
Adriamycin (ADR) (0.125 ug/ml) was used to stimulate confluent conditionally immortalized human podocytes (LY893) for 0 (control) and 48 h. The mRNA expression levels of 7 novel top hub genes were measured by quantitative real-time PCR. The mRNA expression levels of the target genes were normalized to that of GAPDH. The data in three separate experiments were presented as mean ± SD (n=3). *Significantly changed expression levels in ADR-stimulated cells compared with the controls (P<0.05). N.S., no significant difference (P>0.05)
Extended information on Potential Hub Genes
On the basis of the above results, we used the abbreviations of the seven hub genes and FSGS as keywords to search the NCBI database for identifying the potential relationship between these hub genes and FSGS. Search results revealed that the seven hub genes have never been reported in literature related to FSGS. Then, we carefully collated information relevant to the biological functions and signaling pathways that involve these hub genes on Gene Cards website (https://www.genecards.org). The results indicate that heterogeneous nuclear ribonucleoprotein F (HNRNPF) is closely related to Nrf2 gene expression, renal angiotensinogen gene expression, the TGF-β1 signaling pathway, and oxidative stress. Moreover, RNA-binding motif protein 5 (RBM5) is involved in apoptosis induction in many tumors. Ultimately, in accordance with the accepted pathogenesis of FSGS, we selected HNRNPF and RBM5 as representative targets for further discussion. Extended information on HNRNPF and RBM5 are shown in Table 4.
Table 4. Extended Information of The Potential MN-Related Hub Genes.
Gene
|
Function
|
Disease/Cells
|
DOI
|
Authors
|
HNRNPF
|
Stimulates renal Ace-2 gene expression and prevents TGF-β1-induced kidney injury
|
Diabetes
|
10.1007/s00125-015-3700-y
|
Lo CS, Shi Y, Chang SY
|
|
Mediate renal angiotensinogen gene expression and prevention of hypertension and kidney injury
|
Diabetes
|
10.1007/s00125-013-2910-4
|
Abdo S, Lo CS, Chenier I
|
|
Inhibits Nrf2 Gene Expression
|
Diabetic mice
|
10.1210/en.2016-1576
|
Ghosh A, Abdo S, Zhao S
|
|
Against oxidative stress
|
Diabetic mice
|
10.2337/db16-1588
|
Lo CS, Shi Y, Chenier
|
|
Suppresses angiotensinogen gene expression
|
Diabetic mice
|
10.2337/db11-1349
|
Lo CS, Chang SY, Chenier I
|
|
Modulate the alternative splicing of the apoptotic mediator Bcl-x
|
Human HeLa cells
|
10.1074/jbc.M501070200
|
GarneauD,Revil T, Fisette JF
|
|
Modulates angiotensinogen gene expression
|
Diabetes
|
10.1681/ASN.2004080715
|
Wei CC, Guo DF, Zhang SL
|
RBM5
|
Inhibition of Wnt/β-catenin signaling and induction of apoptosis
|
Gliomas
|
10.1186/s12957-016-1084-1
|
Jiang Y, Sheng H, Meng L
|
|
Impacts cell proliferation and apoptosis
|
Lung cancer
|
10.1615/JEnvironPatholToxicolOncol.2017019366
|
Prabhu VV, Devaraj N
|
|
Regulates the activity of Wnt/β-catenin signaling
|
Alveolar epithelial injury
|
10.3892/or.2015.3828
|
Hao YQ, Su ZZ, Lv XJ
|
|
Promotes caspase activation
|
Human neuronal cells
|
10.1038/jcbfm.2014.242
|
Jackson TC, Du L,Janesko-Feldman K
|
|
Promotes neuronal apoptosis
|
Spinal cord injury
|
10.1016/j.biocel.2014.12.020
|
Zhang J, Cui Z, Feng G
|
|
Inhibits cell growth and induces apoptosis
|
Lung adenocarcinoma
|
10.1186/1477-7819-10-160
|
Shao C, Zhao L, Wang K
|
Discussion
Bioinformatics is a newly developed interdisciplinary subject that combines biological science and computer science. Over the past few years, a growing body of research has used gene expression profiles to explore key genes in the pathogenesis of numerous diseases [15,16,40,41]. To our knowledge, our study is the first work that subjected FSGS to bioinformatic analysis. We identified 627 DEGs between the FSGS and control groups. These DEGs included numerous DEGs that have not been previously reported to be involved in FSGS. Then, we predicted DEG functions on the basis of GO annotations. The GO terms we identified included focal adhesion [31,32], cell adhesion molecule [33,34], cadherin [35], and actin binding [36]. These processes are associated with glomerulosclerosis. For example, the genetic deletion of Epb41l5, a podocyte-specific focal adhesome component, results in podocyte detachment, severe proteinuria, and focal segmental glomerulosclerosis development [31]. In immortalized human podocytes, the overexpression of R431C mutant ANLN, an F-actin binding cell cycle gene, enhances podocyte motility [36]. Next, we identified seven overlapping hub genes by constructing PINs through four different analytical methods. Through an accurate search of the NCBI database, we identified HNRNPF and RBM5 as the representative targets for further elaboration.
HNRNPF is a protein-coding gene associated with gene expression. However, no research has been conducted on the role and mechanisms of HNRNPF in FSGS. In this work, we found that HNRNPF is an important DEG among the seven overlapping hub genes in the PPI networks. In addition, HNRNPF is deeply involved with Nrf2 [42], a renal angiotensinogen gene that is expressed in the kidney [43]. Furthermore, in diabetic mice, HNRNPF participates in the TGF-β1 signaling pathway [44] and oxidative stress [45]. These genes and pathways have been confirmed to play vital roles in the pathogenesis of FSGS [4,11,46]. One research suggested that osthole could improve FSGS by activating the Nrf2 antioxidant pathway [47]. TGF-β1 reduces WT1 expression in mouse podocytes and cultured human podocytes before overt glomerulosclerosis begins [46]. In addition, damage to podocytes stimulates TGF-β1 and TGF-βIIR expression in glomerular epithelial cells; this effect eventually leads to extracellular matrix overproduction [48]. On the basis of our analytical results, we conclude that HNRNPF likely participates in FSGS through oxidative stress-associated genes and pathways and is a potential biomarker for this disease.
RBM5 is a nuclear RNA-binding protein that is often genetically deleted in renal cancer49. Unfortunately, the role of RBM5 in FSGS remains unreported. In our study, we identified RBM5 as an up-regulated hub gene in FSGS. Moreover, RBM5 actively participates in apoptosis induction in tumors [50,51]. Apoptosis-induced podocyte damage is a key factor in the pathogenesis of FSGS [12,52]. On the basis of previous findings combined with our present bioinformatic analysis results, we speculate that RBM5 may participate in apoptosis promotion during FSGS progression.
Conclusion
Our study provides a fast, powerful, and effective strategy for the discovery of novel diagnostic biomarkers and therapeutic targets for FSGS. Our results suggest that HNRNPF and RBM5 are molecular candidates for the diagnosis and treatment of FSGS. However, our results are preliminary, and further work is needed to decipher these candidate genes.
Author contributions
Q.M and Z.H designed the research; Z.H analyzed the data and performed the research; D.Z wrote the manuscript. All authors read and approved the final manuscript.
Qianhong Ma and Dongmei Zhang contributed equally to this work and should be considered co-first authors.
Funding
This work was supported by grants from the National Natural Science Foundation of China (Grant No. 81200453).
References
- Pollak MR (2008) Focal segmental glomerulosclerosis: recent advancesyper. Curr Opin Nephrol H tens 17: 138–142.
- Wiggins RC (2007) The spectrum of podocytopathies: a unifying view of glomerular diseases. Kidney Int 71: 1205–1214.
- Kriz W, Lemley KV (2015) A potential role for mechanical forces in the detachment of podocytes and the progression of CKD. J Am Soc Nephrol 26: 258–269.
- Lin EY, Bayarsengee U, Wang CC, Chiang YH, Cheng CW (2018) The natural compound 2,3,5,4’-tetrahydroxystilbene-2-O-beta-d glucoside protects against adriamycin-induced nephropathy through activating the Nrf2-Keap1 antioxidant pathway. Environ Toxicol 33: 72–82.
- Raij L, Tian R, Wong JS, He JC, Campbell KN, et al. (2016) Podocyte injury: the role of proteinuria, urinary plasminogen, and oxidative stress. Am J Physiol Renal Physiol 311: 1308–1317.
- Yang SM, Ka SM, Hua KF, Wu TF, Chuang YP, et al. (2013) Antroquinonol mitigates an accelerated and progressive IgA nephropathy model in mice by activating the Nrf2 pathway and inhibiting T cells and NLRP3 inflammasome. Free Radic Biol Med 61: 285–297.
- Oh CJ, Kim JY, Choi YK, Lee IK, Kim HJ, et al. (2012) Dimethylfumarate attenuates renal fibrosis via NF-E2-related factor 2-mediated inhibition of transforming growth factor-beta/Smad signaling. PloS one 7: 45870.
- Tsai PY, Ka SM, Chao TK, Chang JM, Lin SH, et al. (2011) Antroquinonol reduces oxidative stress by enhancing the Nrf2 signaling pathway and inhibits inflammation and sclerosis in focal segmental glomerulosclerosis mice. Free Radic Biol Med 50: 1503–1516.
- Schiffer M, Bitzer M, Roberts IS, Kopp JB, Mundel P, et al. (2001) Apoptosis in podocytes induced by TGF-beta and Smad7. J Clin Invest 108: 807–816.
- Schachter AD, Strehlau J, Zurakowski D, Kim YS, Strom TB, et al. (2000) Increased nuclear factor-kappaB and angiotensinogen gene expression in posttransplant recurrent focal segmental glomerulosclerosis. Transplantation 70: 1107–1110.
- Luther Y, Bantis C, Ivens K, Fehsel K, Kolb-Bachhofen V, et al. (2003) Effects of the genetic polymorphisms of the renin-angiotensin system on focal segmental glomerulosclerosis. Kidney Blood Press Res 26: 333–337.
- Liu L, Lin W, Zhang Q, Cao W, Liu Z (2016) TGF-beta induces miR-30d down-regulation and podocyte injury through Smad2/3 and HDAC3-associated transcriptional repression. J Mol Med (Berl) 94: 291–300.
- Xue Y, Yin P, Li G, Zhong D (2018) Genome-wide Integration Study of Circulating miRNAs and Peripheral Whole-Blood mRNAs of Male Acute Ischemic Stroke Patients. Neuroscience 380: 27–37.
- Li H, Zhen Y, Geng Y, Feng J, Wang J (2018) Exploring the Molecular Mechanism of Thoracic Aortic Aneurysm via Bioinformatics Analysis. Med Sci Monit 24: 1533–1539.
- Dong B, Wang G, Yao J, Yuan P, Kang W, et al. (2017) Predicting novel genes and pathways associated with osteosarcoma by using bioinformatics analysis. Gene 628: 32–37.
- Luo Y, Zhang C, Tang F, Zhao J, Shen C, et al. (2015) Bioinformatics identification of potentially involved microRNAs in Tibetan with gastric cancer based on microRNA profiling. Cancer Cell Int 15: 115.
- Wang JP, Leng JY, Zhang RK, Zhang L, Zang B, et al. (2018) Functional analysis of gene expression profiling-based prediction in bladder cancer. Oncol Lett 15: 8417–8423.
- Dai J, Ma Y, Chu S, Le N, Cao J, et al. (2018) Identification of key genes and pathways in meningioma by bioinformatics analysis. Oncol Lett 15: 8245–8252.
- Lin P, Wen DY, Dang YW, He Y, Yang H, et al. (2018) Comprehensive and Integrative Analysis Reveals the Diagnostic, Clinicopathological and Prognostic Significance of Polo-Like Kinase 1 in Hepatocellular Carcinoma. Cell Physiol Biochem 47: 925–947.
- Yao T, Wang Q, Zhang W, Bian A, Zhang J (2016) Identification of genes associated with renal cell carcinoma using gene expression profiling analysis. Oncol Lett 12: 73–78.
- Shu B, Fang Y, He W, Yang J, Dai C (2018) Identification of macrophage-related candidate genes in lupus nephritis using bioinformatics analysis. Cell Signal 46: 43–51.
- Cui Y, Liu S, Cui W, Gao D, Zhou W, et al. (2017) Identification of potential biomarkers and therapeutic targets for human IgA nephropathy and hypertensive nephropathy by bioinformatics analysis. Molecular medicine reports 16: 3087–3094.
- Zhou LT, Qiu S, Lv LL, Li ZL, Liu H, et al. (2018) Integrative Bioinformatics Analysis Provides Insight into the Molecular Mechanisms of Chronic Kidney Disease. Kidney Blood Press Res 43: 568–581.
- Gautier L, Cope L, Bolstad BM, Irizarry RA (2004) affy–analysis of Affymetrix GeneChip data at the probe level. Bioinformatics 20: 307–315.
- Ritchie ME, Phipson B, Wu D, Hu Y, Shi, et al. (2015) limma powers differential expression analyses for RNA-sequencing and microarray studies. Nucleic Acids Res 43: 47.
- Yu G, Wang LG, Han Y, He QY (2012) Cluster Profiler: an R package for comparing biological themes among gene clusters. OMICS 16: 284–287.
- Chin CH, Chen SH, Wu HH, Ho CW, Ko MT, et al. (2014) cytoHubba: identifying hub objects and sub-networks from complex interactome. BMC Syst Biol 8: 11.
- Chen H, Boutros PC (2011) VennDiagram: a package for the generation of highly-customizable Venn and Euler diagrams in R. BMC Bioinformatics 12: 35.
- Shannon P, Markiel A, Ozier O, Wang JT, Amin N, et al. (2003) Cytoscape: a software environment for integrated models of biomolecular interaction networks. Genome Res 13: 2498–2504.
- Kachurina N, Chung CF, Benderoff E, Babayeva S, Bitzan M, et al. (2016) Novel unbiased assay for circulating podocyte-toxic factors associated with recurrent focal segmental glomerulosclerosis. Am J Physiol Renal Physiol 310: 1148–1156.
- Schell C, Rogg M, Suhm M, Helmstadter M, Sellung D, et al. (2017) The FERM protein EPB41L5 regulates actomyosin contractility and focal adhesion formation to maintain the kidney filtration barrier. Proc Natl Acad Sci U S A 114: 4621–4630.
- Sever S, Schiffer M (2018) Actin dynamics at focal adhesions: a common endpoint and putative therapeutic target for proteinuric kidney diseases. Kidney Int 93: 1298–1307
- Gong W, Chen C, Xiong F, Yang Z, Wang Y, et al. (2016) CKIP-1 ameliorates high glucose-induced expression of fibronectin and intercellular cell adhesion molecule-1 by activating the Nrf2/ARE pathway in glomerular mesangial cells. Biochem Pharmacol 116: 140–152.
- Huang J, Filipe A, Rahuel C, Bonnin P, Mesnard L, et al. ( 2014) Lutheran/basal cell adhesion molecule accelerates progression of crescentic glomerulonephritis in mice. Kidney Int 85: 1123–1136.
- Naves MA, Requiao-Moura LR, Soares MF, Silva-Junior JA, Mastroianni-Kirsztajn G, et al. (2012) Podocyte Wnt/ss-catenin pathway is activated by integrin-linked kinase in clinical and experimental focal segmental glomerulosclerosis. Journal of nephrology 25: 401–409.
- Gbadegesin RA, Hall G, Adeyemo A, Hanke N, Tossidou I, et al. (2014) Mutations in the gene that encodes the F-actin binding protein anillin cause FSGS. Journal of the American Society of Nephrology: JASN 25: 1991–2002.
- Papazachariou L, Papagregoriou G, Hadjipanagi D, Dwmosthenous P, Voskarides K, et al. (2017) Frequent COL4 mutations in familial microhematuria accompanied by later-onset Alport nephropathy due to focal segmental glomerulosclerosis. Clin Genet 92: 517–527.
- Johnstone DB, Zhang J, George B, Leon C, Gachet C, et al. (2011) Podocyte-specific deletion of Myh9 encoding nonmuscle myosin heavy chain 2A predisposes mice to glomerulopathy. Mol Cell Biol 31: 2162–2170.
- Boyer O, Benoit G, Gribouval O, Nevo F, Tete MJ, et al. (2011) Mutations in INF2 are a major cause of autosomal dominant focal segmental glomerulosclerosis. J Am Soc Nephrol 22: 239–245.
- Li WX, He K, Tang L, Dai SX, Li GH, et al. (2017) Comprehensive tissue-specific gene set enrichment analysis and transcription factor analysis of breast cancer by integrating 14 gene expression datasets. Oncotarget 8: 6775–6786.
- Cui H, Zhang Y, Zhang Q, Chen W, Zhao H, et al. (2017) A comprehensive genome-wide analysis of long noncoding RNA expression profile in hepatocellular carcinoma. Cancer Med 6: 2932–2941.
- Ghosh A, Abdo S, Zhao S, Wu CH, Shi Y, et al. (2017) Insulin Inhibits Nrf2 Gene Expression via Heterogeneous Nuclear Ribonucleoprotein F/K in Diabetic Mice. Endocrinology 158: 903–919.
- Wei CC, Guo DF, Zhang SL, Ingelfinger JR, Chan JS (2005) Heterogenous nuclear ribonucleoprotein F modulates angiotensinogen gene expression in rat kidney proximal tubular cells. J Am Soc Nephrol 16: 616–628.
- Lo CS, Shi Y, Chang SY, Abdo S, Chenier I, et al. (2015) Overexpression of heterogeneous nuclear ribonucleoprotein F stimulates renal Ace-2 gene expression and prevents TGF-beta1-induced kidney injury in a mouse model of diabetes. Diabetologia 58: 2443–2454.
- Lo CS, Shi Y, Chenier I, Ghosh A, Wu CH, et al. (2017) Heterogeneous Nuclear Ribonucleoprotein F Stimulates Sirtuin-1 Gene Expression and Attenuates Nephropathy Progression in Diabetic Mice. Diabetes 66: 1964–1978.
- Sakairi T, Abe Y, Kopp JB (2011) TGF-beta1 reduces Wilms’ tumor suppressor gene expression in podocytes. Nephrol Dial Transplant 26: 2746–2752.
- Yang SM, Chan YL, Hua KF, Chang JM, Chen HL, et al. (2014) Osthole improves an accelerated focal segmental glomerulosclerosis model in the early stage by activating the Nrf2 antioxidant pathway and subsequently inhibiting NF-kappaB-mediated COX-2 expression and apoptosis. Free Radic Biol Med 73: 260–269.
- Kim JH, Kim BK, Moon KC, Hong HK, Lee HS (2003) Activation of the TGF-beta/Smad signaling pathway in focal segmental glomerulosclerosis. Kidney Int 64: 1715–1721.
- Bechara EG, Sebestyen E, Bernardis I, Eyras E, Valcarcel J (2013) RBM5, 6, and 10 differentially regulate NUMB alternative splicing to control cancer cell proliferation. Mol Cell 52: 720–733.
- Jiang Y, Sheng H, Meng L, Yue H, Li B, et al. (2017) RBM5 inhibits tumorigenesis of gliomas through inhibition of Wnt/beta-catenin signaling and induction of apoptosis. World J Surg Oncol 15: 9.
- Prabhu VV, Devaraj N (2017) Regulating RNA Binding Motif 5 Gene Expression- A Novel Therapeutic Target for Lung Cancer. J Environ Pathol Toxicol Oncol 36: 99–105.
- Nafar M, Kalantari S, Samavat S, Rezaei-Tavirani M, Rutishuser D, et al. (2014) The novel diagnostic biomarkers for focal segmental glomerulosclerosis. Int J Nephrol 2014: 574261.
Supplementary Table 1
gene.symbols
|
logFC
|
AveExpr
|
ts
|
P.Value
|
adj.P.Val
|
B
|
RPS4Y1
|
2.569009422
|
9.570289361
|
3.101358611
|
0.004371381
|
0.011115768
|
-2.711721859
|
PLPP3
|
2.485089122
|
9.251833831
|
7.62668606
|
2.66E-08
|
7.13E-07
|
9.068379301
|
DDX3Y
|
2.478844353
|
7.003027727
|
2.920309189
|
0.006843408
|
0.016208722
|
-3.132862184
|
SART3
|
2.470577198
|
7.660356798
|
12.25209245
|
9.40E-13
|
5.82E-10
|
19.16340462
|
TCF4
|
2.418989646
|
7.335132517
|
11.59356528
|
3.44E-12
|
1.27E-09
|
17.89787797
|
CYP4A11
|
-2.412274749
|
9.12866545
|
-6.123580669
|
1.33E-06
|
1.42E-05
|
5.192258061
|
FOSB
|
-2.356258176
|
8.074381933
|
-7.877904555
|
1.42E-08
|
4.53E-07
|
9.690625188
|
TROVE2
|
2.355365338
|
9.457713493
|
7.605950945
|
2.80E-08
|
7.39E-07
|
9.016659035
|
IQGAP1
|
2.290645788
|
8.14538406
|
9.69432624
|
1.94E-10
|
2.19E-08
|
13.93671972
|
MBP
|
2.255967535
|
7.587944516
|
12.62989302
|
4.56E-13
|
3.91E-10
|
19.86587003
|
CALD1
|
2.192562666
|
8.074505734
|
11.18804096
|
7.85E-12
|
2.21E-09
|
17.09171526
|
EGR1
|
-2.182777112
|
10.2801361
|
-8.747798502
|
1.72E-09
|
1.01E-07
|
11.78050827
|
RBFOX2
|
2.164462681
|
8.69816763
|
10.33705244
|
4.72E-11
|
8.22E-09
|
15.33081151
|
IGFBP5
|
2.148130254
|
9.995294632
|
5.537086859
|
6.45E-06
|
5.09E-05
|
3.626766637
|
CDC42BPA
|
2.079395261
|
9.770721553
|
7.925115034
|
1.26E-08
|
4.20E-07
|
9.806647308
|
SMAD1
|
2.076607382
|
7.949965987
|
8.465377555
|
3.38E-09
|
1.61E-07
|
11.11324049
|
G6PC
|
-2.074536589
|
6.566342687
|
-4.459033616
|
0.000121983
|
0.000557225
|
0.736400459
|
ALB
|
-2.073497949
|
9.571928342
|
-4.114808392
|
0.000309678
|
0.001209773
|
-0.171567409
|
ZEB1
|
2.066318697
|
8.614493251
|
10.45925661
|
3.63E-11
|
7.03E-09
|
15.5895635
|
TPM1
|
2.060897076
|
9.232448331
|
10.04753441
|
8.88E-11
|
1.30E-08
|
14.70977983
|
FERMT2
|
2.05930113
|
7.350506265
|
7.771402732
|
1.85E-08
|
5.49E-07
|
9.427823748
|
LUC7L3
|
2.047718235
|
8.759118544
|
8.718232863
|
1.85E-09
|
1.06E-07
|
11.71116708
|
RAN
|
2.025548667
|
10.29196832
|
11.46235083
|
4.49E-12
|
1.47E-09
|
17.63930778
|
SLC25A36
|
2.003665861
|
8.269066322
|
8.586494779
|
2.53E-09
|
1.31E-07
|
11.40073865
|
HLA-DRA
|
1.992802428
|
11.12961293
|
7.574703865
|
3.03E-08
|
7.84E-07
|
8.938615772
|
HTR2B
|
1.980142562
|
6.49870054
|
5.606952454
|
5.34E-06
|
4.35E-05
|
3.814138409
|
PCYOX1
|
1.966411418
|
7.433879894
|
7.068593108
|
1.10E-07
|
2.03E-06
|
7.65787493
|
ATRX
|
1.961414528
|
6.588331245
|
11.16381872
|
8.25E-12
|
2.27E-09
|
17.04289981
|
DYNC1LI2
|
1.955508721
|
9.605304116
|
10.85559765
|
1.57E-11
|
3.64E-09
|
16.41511633
|
CD99
|
1.9554319
|
9.927197771
|
8.57318363
|
2.61E-09
|
1.33E-07
|
11.36923982
|
CHD4
|
1.94490416
|
8.814477542
|
16.59170115
|
5.35E-16
|
9.51E-12
|
26.31762235
|
MYLIP
|
1.938154662
|
9.419703765
|
7.548038454
|
3.24E-08
|
8.23E-07
|
8.871918527
|
HNRNPF
|
1.934376435
|
8.42993207
|
13.0216741
|
2.19E-13
|
2.32E-10
|
20.57678872
|
HLA-DQB1
|
1.933559554
|
9.283173095
|
6.877515473
|
1.81E-07
|
2.96E-06
|
7.16657257
|
FHL1
|
1.916965148
|
7.704294928
|
10.06888924
|
8.47E-11
|
1.27E-08
|
14.75597408
|
CTSZ
|
-1.912814074
|
8.256096924
|
-9.422794947
|
3.59E-10
|
3.49E-08
|
13.3308037
|
DDX17
|
1.900816911
|
7.156016292
|
6.849768897
|
1.94E-07
|
3.11E-06
|
7.094896981
|
THUMPD1
|
1.891011353
|
7.141971356
|
13.73428793
|
6.01E-14
|
1.12E-10
|
21.82576576
|
PPP3R1
|
-1.881530742
|
7.627366273
|
-7.148865697
|
8.96E-08
|
1.74E-06
|
7.863044486
|
TNFRSF11B
|
1.87836792
|
7.853323079
|
7.02736233
|
1.23E-07
|
2.20E-06
|
7.552206366
|
XIST
|
-1.863860797
|
7.615665673
|
-2.956875261
|
0.006256155
|
0.015025427
|
-3.048847061
|
SON
|
1.851258175
|
7.997946847
|
8.840334385
|
1.39E-09
|
8.53E-08
|
11.99675806
|
GLUL
|
1.846739708
|
7.937765075
|
9.235752198
|
5.51E-10
|
4.67E-08
|
12.90751377
|
DKK3
|
1.838529383
|
7.235094143
|
6.88002716
|
1.80E-07
|
2.95E-06
|
7.173056725
|
BTG1
|
1.820054032
|
7.907833291
|
11.00485807
|
1.15E-11
|
2.94E-09
|
16.72066783
|
TRIB2
|
1.81921404
|
8.182950746
|
8.768912824
|
1.64E-09
|
9.74E-08
|
11.82995473
|
RBM5
|
1.808667325
|
8.306181873
|
13.85226391
|
4.87E-14
|
1.09E-10
|
22.02721576
|
HLA-B
|
1.803285216
|
12.34984324
|
6.340923899
|
7.42E-07
|
8.94E-06
|
5.766672368
|
MYH10
|
1.802983311
|
6.632652763
|
13.31027341
|
1.29E-13
|
1.80E-10
|
21.08934934
|
STAG2
|
1.793115173
|
5.860191501
|
13.76494033
|
5.69E-14
|
1.12E-10
|
21.87824888
|
GBP1
|
1.783205635
|
6.97780633
|
8.494851598
|
3.15E-09
|
1.53E-07
|
11.18338801
|
RPA1
|
1.779726763
|
9.056001662
|
9.084532378
|
7.82E-10
|
5.91E-08
|
12.56176241
|
BPTF
|
1.776426474
|
7.907083176
|
7.356751223
|
5.27E-08
|
1.17E-06
|
8.390867161
|
PCK1
|
-1.772301574
|
10.99438749
|
-4.775458625
|
5.15E-05
|
0.000272763
|
1.580947098
|
BTN3A3
|
1.772184439
|
6.173764331
|
8.425121851
|
3.73E-09
|
1.74E-07
|
11.01724161
|
TAGLN
|
1.764332395
|
9.007016683
|
5.779780851
|
3.34E-06
|
3.00E-05
|
4.276795382
|
PSMA7
|
1.763068956
|
6.889044422
|
11.69687487
|
2.80E-12
|
1.22E-09
|
18.09994223
|
HLA-DPA1
|
1.762375082
|
10.82315093
|
6.098738113
|
1.42E-06
|
1.50E-05
|
5.126369472
|
MYLK
|
1.75861114
|
10.39524956
|
6.152944852
|
1.23E-06
|
1.34E-05
|
5.270080435
|
YIPF6
|
1.747988074
|
9.294461389
|
8.337256865
|
4.61E-09
|
2.03E-07
|
10.80694239
|
COL3A1
|
1.741079028
|
10.03488916
|
6.880350494
|
1.80E-07
|
2.95E-06
|
7.173891393
|
ACTB
|
1.739818254
|
10.62946301
|
4.630671948
|
7.64E-05
|
0.000379292
|
1.193646749
|
DHX15
|
1.737775319
|
10.02003843
|
9.85519139
|
1.36E-10
|
1.72E-08
|
14.29091385
|
PPIG
|
1.731145889
|
7.407036172
|
7.251707604
|
6.89E-08
|
1.43E-06
|
8.124803038
|
SCAF11
|
1.721893208
|
9.371324456
|
10.2275776
|
5.99E-11
|
9.60E-09
|
15.09731143
|
HPGD
|
-1.717401682
|
10.07266901
|
-6.740627536
|
2.59E-07
|
3.87E-06
|
6.812168581
|
DPP8
|
1.715249149
|
9.638588643
|
8.318534824
|
4.82E-09
|
2.09E-07
|
10.76199707
|
WSB1
|
1.714938423
|
8.596432117
|
6.91877743
|
1.62E-07
|
2.75E-06
|
7.273007517
|
ENC1
|
1.714016384
|
6.351234133
|
9.286980973
|
4.90E-10
|
4.30E-08
|
13.02392792
|
FN1
|
1.713084538
|
9.852710944
|
6.048869862
|
1.62E-06
|
1.68E-05
|
4.993973035
|
UPF3A
|
1.706676789
|
8.950115438
|
11.59802708
|
3.41E-12
|
1.27E-09
|
17.90663237
|
KRT19
|
1.703164941
|
8.133804492
|
4.840602977
|
4.31E-05
|
0.000235133
|
1.755562232
|
BCLAF1
|
1.697469525
|
8.139217001
|
9.830297038
|
1.44E-10
|
1.80E-08
|
14.23633277
|
FBXO21
|
1.697287783
|
9.28818289
|
5.462538041
|
7.90E-06
|
5.94E-05
|
3.426663378
|
IFNGR1
|
1.695558168
|
9.158556204
|
8.094628782
|
8.32E-09
|
3.11E-07
|
10.22081389
|
HCK
|
1.695111511
|
7.690905947
|
7.857688804
|
1.49E-08
|
4.67E-07
|
9.640854784
|
CXCL2
|
-1.686811585
|
6.477878156
|
-4.50798237
|
0.000106777
|
0.000500487
|
0.866554554
|
PHACTR2
|
1.679845963
|
7.782456361
|
7.807529456
|
1.69E-08
|
5.14E-07
|
9.517134367
|
HNRNPM
|
1.673764753
|
11.0821542
|
11.69753948
|
2.80E-12
|
1.22E-09
|
18.10123784
|
CAMK2N1
|
-1.651602298
|
10.19208306
|
-6.765897326
|
2.42E-07
|
3.70E-06
|
6.877740372
|
FUS
|
1.650968802
|
9.619854977
|
13.27029455
|
1.39E-13
|
1.82E-10
|
21.01889984
|
PLPBP
|
1.650135185
|
5.59234436
|
12.81694912
|
3.21E-13
|
2.98E-10
|
20.20749556
|
PSMB8
|
1.646543823
|
10.456135
|
7.886039857
|
1.39E-08
|
4.48E-07
|
9.71063892
|
SEC63
|
1.640469887
|
7.954060099
|
6.382735607
|
6.64E-07
|
8.19E-06
|
5.876729688
|
PALLD
|
1.635448714
|
8.943061698
|
7.556440719
|
3.17E-08
|
8.09E-07
|
8.892944454
|
ZBTB16
|
1.635125895
|
7.66230392
|
3.653696968
|
0.001057166
|
0.003348994
|
-1.358707431
|
RBM25
|
1.63291277
|
6.324655258
|
8.650201845
|
2.17E-09
|
1.18E-07
|
11.5511557
|
TYROBP
|
1.630051539
|
7.893243583
|
5.141218255
|
1.90E-05
|
0.00012023
|
2.563038692
|
DACH1
|
1.623091259
|
7.101855154
|
6.801703043
|
2.20E-07
|
3.44E-06
|
6.970537686
|
PSMC3
|
1.612543512
|
9.366600505
|
5.93353245
|
2.21E-06
|
2.14E-05
|
4.68711502
|
RBMS1
|
1.6103609
|
8.650805194
|
4.65105936
|
7.23E-05
|
0.000362333
|
1.248105923
|
PGK1
|
1.607870056
|
10.36413941
|
5.403307466
|
9.28E-06
|
6.73E-05
|
3.267574125
|
NPEPPS
|
1.606521068
|
8.421329051
|
7.835158094
|
1.58E-08
|
4.88E-07
|
9.585322216
|
PTPRB
|
1.604906513
|
9.444331687
|
7.330988837
|
5.62E-08
|
1.23E-06
|
8.325736785
|
TNPO1
|
1.599343907
|
9.775968964
|
8.87594367
|
1.27E-09
|
8.10E-08
|
12.07966014
|
EZR
|
1.591497713
|
8.757810465
|
5.97334531
|
1.98E-06
|
1.97E-05
|
4.793136085
|
MAGED2
|
1.586605561
|
10.30790428
|
12.89378949
|
2.78E-13
|
2.81E-10
|
20.3466641
|
ANKRD12
|
1.580638115
|
7.392354398
|
6.693843176
|
2.92E-07
|
4.26E-06
|
6.690596121
|
FNBP1
|
1.575997647
|
8.157390697
|
8.31499427
|
4.86E-09
|
2.10E-07
|
10.75349206
|
NR4A2
|
-1.57481871
|
6.24079609
|
-8.527861329
|
2.91E-09
|
1.45E-07
|
11.26180965
|
SERBP1
|
1.573844316
|
8.951883468
|
11.22792569
|
7.23E-12
|
2.19E-09
|
17.17193154
|
SPAG9
|
1.573771613
|
5.928584131
|
12.82512531
|
3.16E-13
|
2.98E-10
|
20.22233593
|
SCAMP1
|
1.572326984
|
7.135740239
|
7.680340099
|
2.32E-08
|
6.50E-07
|
9.201956772
|
RAB1A
|
1.571530153
|
8.448144883
|
6.477980274
|
5.16E-07
|
6.71E-06
|
6.126856918
|
TAOK3
|
1.570396428
|
7.085559648
|
9.345798665
|
4.28E-10
|
3.85E-08
|
13.15714066
|
SRGN
|
1.568368396
|
9.423138514
|
5.18175814
|
1.70E-05
|
0.000109899
|
2.672037194
|
ZFYVE21
|
1.5676445
|
6.846082627
|
7.262978403
|
6.69E-08
|
1.40E-06
|
8.153414092
|
HRG
|
-1.562922635
|
8.320562127
|
-4.315974661
|
0.000179859
|
0.000770138
|
0.357348521
|
PWP1
|
1.561834997
|
8.446947472
|
8.857117309
|
1.33E-09
|
8.24E-08
|
12.03585221
|
SKAP2
|
1.561530224
|
9.277742191
|
7.33501641
|
5.57E-08
|
1.22E-06
|
8.335924267
|
UBXN4
|
1.551349919
|
9.105993907
|
6.390043816
|
6.51E-07
|
8.06E-06
|
5.895950838
|
PECAM1
|
1.550870521
|
9.054536986
|
6.663178499
|
3.17E-07
|
4.53E-06
|
6.610791348
|
MBNL2
|
1.550572397
|
7.066502239
|
10.39994319
|
4.12E-11
|
7.62E-09
|
15.46422432
|
SOX9
|
-1.548564432
|
8.004071342
|
-6.562764085
|
4.13E-07
|
5.60E-06
|
6.348811078
|
TRIM2
|
1.546572067
|
8.578770031
|
8.831695492
|
1.41E-09
|
8.68E-08
|
11.97661948
|
HNRNPR
|
1.545721471
|
9.080452193
|
10.58685737
|
2.76E-11
|
5.70E-09
|
15.85761598
|
MYOF
|
1.54258444
|
8.617870178
|
7.043673577
|
1.18E-07
|
2.13E-06
|
7.594032778
|
NR3C1
|
1.537314952
|
8.658438842
|
7.183782173
|
8.20E-08
|
1.62E-06
|
7.95205499
|
TFPI2
|
1.530489929
|
8.411366859
|
3.999919431
|
0.000421606
|
0.001565513
|
-0.471022596
|
TMEM47
|
1.524753951
|
8.263583054
|
6.004266035
|
1.83E-06
|
1.84E-05
|
4.875407299
|
PURA
|
1.522929493
|
7.624254878
|
6.128405127
|
1.31E-06
|
1.41E-05
|
5.20504847
|
RAB31
|
1.519313807
|
8.597342752
|
6.411935462
|
6.15E-07
|
7.72E-06
|
5.95349937
|
CYB5B
|
1.515075529
|
7.815221603
|
11.7624269
|
2.46E-12
|
1.16E-09
|
18.22746762
|
RIT1
|
1.514871553
|
7.235874533
|
7.407198303
|
4.63E-08
|
1.07E-06
|
8.518169631
|
PRPF31
|
1.51015233
|
6.970023854
|
7.948526057
|
1.19E-08
|
4.05E-07
|
9.864072564
|
ALDOB
|
-1.504568555
|
8.472159452
|
-3.061872507
|
0.004824275
|
0.012090824
|
-2.80464753
|
MAP4
|
1.503555867
|
8.031894594
|
5.975611845
|
1.97E-06
|
1.96E-05
|
4.799168802
|
RAB2A
|
-1.502751043
|
8.315889782
|
-16.2138848
|
9.61E-16
|
9.51E-12
|
25.7672033
|
BTN3A2
|
1.502705438
|
7.877942556
|
6.982291597
|
1.38E-07
|
2.40E-06
|
7.436477371
|
GMDS
|
1.497770885
|
9.599429406
|
7.605970724
|
2.80E-08
|
7.39E-07
|
9.016708396
|
CD163
|
1.496518651
|
7.363641477
|
3.780358703
|
0.000756817
|
0.002538255
|
-1.036824644
|
PKN2
|
1.492252244
|
7.396561746
|
9.26254208
|
5.18E-10
|
4.46E-08
|
12.96843728
|
PICALM
|
1.48883338
|
7.452309182
|
7.882640103
|
1.40E-08
|
4.50E-07
|
9.702276208
|
LYN
|
1.48458898
|
7.748906756
|
6.995137981
|
1.33E-07
|
2.35E-06
|
7.469486452
|
ARL6IP5
|
1.481819394
|
9.42435093
|
6.181264534
|
1.14E-06
|
1.26E-05
|
5.345073096
|
SH3BP5
|
1.480757339
|
9.948617496
|
7.175018224
|
8.38E-08
|
1.65E-06
|
7.929726958
|
CYTH2
|
1.478569815
|
8.695293012
|
14.0977443
|
3.17E-14
|
7.84E-11
|
22.44167418
|
HSP90AA1
|
1.475101446
|
10.7822491
|
5.488461362
|
7.36E-06
|
5.62E-05
|
3.49626449
|
PDPK1
|
1.474624621
|
8.188917333
|
5.243240876
|
1.44E-05
|
9.60E-05
|
2.837346192
|
SMC3
|
1.470872089
|
7.133595994
|
11.12401077
|
8.96E-12
|
2.41E-09
|
16.96251038
|
MBTPS1
|
1.465393655
|
8.496997806
|
7.766899015
|
1.87E-08
|
5.53E-07
|
9.416678054
|
TIMP3
|
1.463150322
|
9.290336056
|
7.335248955
|
5.56E-08
|
1.22E-06
|
8.336512413
|
SSRP1
|
1.462015221
|
7.615804716
|
9.043612741
|
8.60E-10
|
6.37E-08
|
12.46766016
|
NAA35
|
1.458486577
|
6.726259229
|
8.092465915
|
8.37E-09
|
3.11E-07
|
10.21555345
|
KIF5B
|
1.454182588
|
8.394875765
|
5.616323045
|
5.20E-06
|
4.27E-05
|
3.839255868
|
DHRS7
|
1.454116053
|
7.744616405
|
9.572938924
|
2.56E-10
|
2.65E-08
|
13.66709981
|
CNPY2
|
1.453764841
|
8.534477403
|
8.634059418
|
2.26E-09
|
1.20E-07
|
11.51309488
|
FKBP5
|
1.452723718
|
7.291698701
|
4.149986734
|
0.000281682
|
0.001116854
|
-0.079470163
|
COL6A3
|
1.447501816
|
7.827000817
|
4.905985588
|
3.61E-05
|
0.000203504
|
1.930987853
|
COL4A2
|
1.446665268
|
7.456526747
|
6.876736022
|
1.81E-07
|
2.96E-06
|
7.164560203
|
CALB1
|
-1.44533189
|
9.757638848
|
-2.605850418
|
0.01452899
|
0.030801016
|
-3.831208449
|
IGF1
|
-1.437115619
|
9.406113438
|
-3.336824653
|
0.002407335
|
0.006723822
|
-2.146238464
|
NELFCD
|
1.434489749
|
9.91051469
|
11.67139035
|
2.95E-12
|
1.22E-09
|
18.0502204
|
YWHAB
|
1.431615777
|
8.640142735
|
5.544355542
|
6.32E-06
|
5.00E-05
|
3.646268215
|
RGCC
|
1.429769752
|
7.679603868
|
4.732902335
|
5.78E-05
|
0.000299666
|
1.466986783
|
SLC25A6
|
1.428984166
|
11.61631244
|
9.101576376
|
7.52E-10
|
5.75E-08
|
12.60089001
|
ID3
|
1.428233521
|
10.14589451
|
8.266105018
|
5.47E-09
|
2.29E-07
|
10.63587854
|
TRA2B
|
1.424948669
|
9.497698712
|
8.624014607
|
2.31E-09
|
1.22E-07
|
11.48939306
|
GDI2
|
1.424531564
|
9.161869349
|
6.615965347
|
3.59E-07
|
4.97E-06
|
6.48773575
|
CRHBP
|
1.423223574
|
10.85750131
|
3.501852173
|
0.001572242
|
0.004679346
|
-1.739506163
|
EID1
|
1.422600712
|
9.21370437
|
7.376173329
|
5.01E-08
|
1.13E-06
|
8.439915301
|
SET
|
1.420718447
|
10.19887727
|
8.55531067
|
2.73E-09
|
1.37E-07
|
11.32690802
|
FOS
|
-1.420440832
|
9.273302043
|
-3.000916158
|
0.005612259
|
0.013756238
|
-2.946942453
|
VDAC1
|
1.415009019
|
8.172894871
|
7.118018567
|
9.71E-08
|
1.85E-06
|
7.784289799
|
WASHC4
|
1.412990786
|
7.331446343
|
8.01490027
|
1.01E-08
|
3.56E-07
|
10.02649009
|
UMOD
|
-1.412526572
|
11.37016849
|
-2.38566243
|
0.024069305
|
0.047104894
|
-4.291378203
|
SERTAD2
|
1.411604795
|
8.175798374
|
8.513812449
|
3.01E-09
|
1.48E-07
|
11.22845173
|
PQBP1
|
1.411256434
|
7.748400335
|
6.769612323
|
2.40E-07
|
3.68E-06
|
6.887374717
|
CEP350
|
1.409862497
|
6.337432566
|
7.135793044
|
9.27E-08
|
1.79E-06
|
7.829682626
|
GLYAT
|
-1.407015938
|
9.579743485
|
-4.089844356
|
0.000331191
|
0.001276585
|
-0.236812106
|
ICAM2
|
1.406910331
|
9.771163793
|
5.247150209
|
1.42E-05
|
9.52E-05
|
2.84785686
|
GNB1
|
1.402945544
|
9.130430125
|
10.649444
|
2.42E-11
|
5.13E-09
|
15.98830239
|
HLA-DMB
|
1.401194865
|
10.17298113
|
8.848947598
|
1.36E-09
|
8.38E-08
|
12.01682652
|
EDNRB
|
1.398466757
|
8.373823696
|
4.94196731
|
3.27E-05
|
0.000188026
|
2.02759004
|
ESF1
|
1.398047621
|
7.471743775
|
13.05457765
|
2.06E-13
|
2.30E-10
|
20.63569798
|
AIDA
|
1.397041007
|
7.692499594
|
8.873300307
|
1.28E-09
|
8.11E-08
|
12.07351212
|
TOP1
|
1.396150489
|
8.310821776
|
7.122657614
|
9.59E-08
|
1.83E-06
|
7.796140615
|
SNX1
|
1.393315129
|
7.77005298
|
9.617845685
|
2.31E-10
|
2.46E-08
|
13.76708049
|
CLDND1
|
1.389278478
|
8.356235731
|
7.353059136
|
5.32E-08
|
1.18E-06
|
8.381538064
|
TSPAN3
|
1.38922393
|
9.719295375
|
4.512799487
|
0.000105387
|
0.000495607
|
0.879374553
|
SLC7A8
|
1.388681022
|
9.335582412
|
4.354999431
|
0.000161804
|
0.000706402
|
0.460535324
|
ARHGAP5
|
-1.388500976
|
8.373041869
|
-8.710601093
|
1.88E-09
|
1.07E-07
|
11.69324854
|
ILF3
|
1.386329503
|
6.940492403
|
8.230768777
|
5.97E-09
|
2.45E-07
|
10.55066888
|
ATG12
|
1.384776561
|
7.242819619
|
7.607340868
|
2.79E-08
|
7.39E-07
|
9.020127669
|
ZNF148
|
1.382618168
|
8.099582442
|
12.45022932
|
6.42E-13
|
4.62E-10
|
19.53391159
|
DLC1
|
1.379754481
|
9.408529743
|
8.406044121
|
3.90E-09
|
1.80E-07
|
10.97166939
|
MYDGF
|
1.379051924
|
8.854924998
|
9.767015768
|
1.65E-10
|
1.97E-08
|
14.09720669
|
PSD3
|
1.379024578
|
6.766600545
|
5.210698818
|
1.57E-05
|
0.000103008
|
2.749850991
|
CDC37
|
1.377078992
|
9.468182931
|
10.45472383
|
3.67E-11
|
7.04E-09
|
15.58000157
|
KPNB1
|
1.376929789
|
9.458847188
|
9.024827414
|
8.99E-10
|
6.56E-08
|
12.4243824
|
FOXN3
|
1.375967793
|
6.83901797
|
8.165674647
|
6.99E-09
|
2.76E-07
|
10.39326148
|
LIMCH1
|
1.375409137
|
9.097684708
|
5.56555133
|
5.97E-06
|
4.77E-05
|
3.703125677
|
MLEC
|
1.374524107
|
9.243909884
|
6.009168559
|
1.80E-06
|
1.82E-05
|
4.888445732
|
FABP5
|
1.370563815
|
7.473351905
|
3.638781888
|
0.001099405
|
0.003461152
|
-1.396367326
|
SCNN1A
|
-1.367945371
|
9.167955994
|
-4.114464255
|
0.000309965
|
0.001210427
|
-0.172467464
|
TPR
|
1.367879082
|
8.216314258
|
4.77899159
|
5.10E-05
|
0.000270724
|
1.590412014
|
HSP90AB1
|
1.36640902
|
9.19132412
|
5.419142441
|
8.89E-06
|
6.51E-05
|
3.310113519
|
ABCF2
|
1.364898619
|
7.12749747
|
8.687031396
|
1.99E-09
|
1.11E-07
|
11.63785903
|
HNRNPD
|
1.36405605
|
9.094619756
|
8.738208165
|
1.76E-09
|
1.02E-07
|
11.75802893
|
TGFBR2
|
1.362070619
|
7.153715484
|
5.381838143
|
9.84E-06
|
7.03E-05
|
3.209890462
|
CD14
|
1.361767811
|
8.618764289
|
5.636868061
|
4.92E-06
|
4.09E-05
|
3.894313936
|
FRZB
|
1.361170602
|
9.126704666
|
5.082735903
|
2.22E-05
|
0.000137296
|
2.405816869
|
BBS4
|
1.360150632
|
7.202996413
|
8.971308938
|
1.02E-09
|
7.08E-08
|
12.30081903
|
HLA-G
|
1.359808391
|
10.72098347
|
5.244182836
|
1.43E-05
|
9.58E-05
|
2.839878762
|
SWAP70
|
1.359427598
|
9.113593244
|
5.306026158
|
1.21E-05
|
8.33E-05
|
3.006138059
|
TNPO3
|
1.358231729
|
7.693616767
|
10.42872351
|
3.88E-11
|
7.26E-09
|
15.52510066
|
LACTB2
|
-1.355852383
|
7.530616
|
-6.671617676
|
3.10E-07
|
4.45E-06
|
6.632763722
|
CEP57
|
1.352993373
|
5.052119046
|
12.37324173
|
7.44E-13
|
4.88E-10
|
19.39050197
|
MYO6
|
1.352421261
|
9.176047271
|
5.255177235
|
1.39E-05
|
9.36E-05
|
2.869438127
|
EPRS
|
1.351852256
|
8.607022753
|
8.649814499
|
2.17E-09
|
1.18E-07
|
11.55024283
|
LRBA
|
-1.349799955
|
8.811192382
|
-8.795602403
|
1.54E-09
|
9.30E-08
|
11.8923698
|
CXCR4
|
1.347649061
|
8.222517485
|
3.684201964
|
0.000975644
|
0.003135087
|
-1.281519314
|
SRRM2
|
1.347568684
|
8.460042382
|
5.001654871
|
2.78E-05
|
0.000165066
|
2.187913815
|
RPLP0
|
1.34749233
|
11.59881981
|
12.16742107
|
1.11E-12
|
6.17E-10
|
19.00364445
|
ITGA8
|
1.343209501
|
8.187995584
|
5.506346749
|
7.01E-06
|
5.43E-05
|
3.544273858
|
DDX39A
|
1.342371076
|
8.373749992
|
14.91487814
|
7.84E-15
|
2.61E-11
|
23.77683811
|
SSBP2
|
1.341694376
|
8.032258834
|
10.36379012
|
4.46E-11
|
8.01E-09
|
15.38759602
|
HMGCR
|
1.340708611
|
7.991412194
|
7.949010066
|
1.19E-08
|
4.05E-07
|
9.865259037
|
STX11
|
1.338253755
|
6.131744045
|
8.963225837
|
1.04E-09
|
7.12E-08
|
12.28212241
|
TTF1
|
1.335503511
|
7.454129952
|
7.565990872
|
3.10E-08
|
7.95E-07
|
8.916832104
|
AZIN1
|
1.331515063
|
6.892036517
|
13.60307467
|
7.59E-14
|
1.17E-10
|
21.59996308
|
OAS1
|
1.328744906
|
7.180037476
|
4.581369291
|
8.74E-05
|
0.000424389
|
1.062067683
|
PLEKHB1
|
-1.325016546
|
7.570574431
|
-8.601436826
|
2.44E-09
|
1.27E-07
|
11.43606785
|
TMEM230
|
1.322637972
|
8.978392691
|
6.664841119
|
3.15E-07
|
4.52E-06
|
6.615120736
|
BMPR2
|
1.321446254
|
6.459157792
|
7.530440342
|
3.39E-08
|
8.48E-07
|
8.827852131
|
ZNF207
|
1.320509797
|
9.748881671
|
7.924554461
|
1.27E-08
|
4.20E-07
|
9.805271384
|
RAD21
|
1.320165778
|
7.728846175
|
7.217996731
|
7.51E-08
|
1.53E-06
|
8.039137581
|
HCLS1
|
1.318686529
|
9.088478783
|
7.557331729
|
3.17E-08
|
8.09E-07
|
8.895173607
|
COG7
|
1.318315987
|
7.957007308
|
6.947663903
|
1.51E-07
|
2.60E-06
|
7.34740937
|
TSPAN5
|
1.317168741
|
7.574432985
|
7.172334408
|
8.44E-08
|
1.66E-06
|
7.922887565
|
CALR
|
-1.316887257
|
9.46952319
|
-6.187797543
|
1.12E-06
|
1.24E-05
|
5.362364282
|
CASP1
|
1.315406814
|
7.280061706
|
6.917554285
|
1.63E-07
|
2.75E-06
|
7.269855086
|
CD53
|
1.314626339
|
9.310209635
|
4.82374315
|
4.51E-05
|
0.000244358
|
1.710352793
|
STAT1
|
1.311254404
|
7.989702392
|
8.164628307
|
7.01E-09
|
2.76E-07
|
10.39072663
|
NASP
|
1.311023485
|
8.658422087
|
8.195333579
|
6.50E-09
|
2.59E-07
|
10.46505182
|
ATF3
|
-1.309777911
|
9.32422844
|
-4.436888056
|
0.000129551
|
0.000586505
|
0.677587657
|
LRRFIP1
|
1.308595239
|
9.842768068
|
6.02460185
|
1.73E-06
|
1.77E-05
|
4.929480606
|
BLVRA
|
1.307890164
|
7.463070636
|
7.883905699
|
1.40E-08
|
4.49E-07
|
9.705389497
|
TRAM2
|
1.307573251
|
8.603172154
|
6.048923045
|
1.62E-06
|
1.68E-05
|
4.994114324
|
SPTLC1
|
1.306960571
|
7.365444445
|
8.208877143
|
6.29E-09
|
2.54E-07
|
10.49779515
|
COL4A3
|
1.303589773
|
10.63769098
|
5.004570724
|
2.75E-05
|
0.000164118
|
2.195748058
|
DDX41
|
1.303536766
|
9.473571199
|
11.00669283
|
1.14E-11
|
2.94E-09
|
16.72440575
|
ACTR3
|
1.302268329
|
10.75060111
|
7.097878642
|
1.02E-07
|
1.93E-06
|
7.732811998
|
ACLY
|
1.302204054
|
8.910503391
|
7.337433307
|
5.53E-08
|
1.22E-06
|
8.342036709
|
FNTA
|
1.301806985
|
9.235960273
|
8.985880851
|
9.85E-10
|
6.90E-08
|
12.33450181
|
FCN1
|
1.301737217
|
7.825729707
|
4.507676388
|
0.000106866
|
0.000500799
|
0.865740298
|
GPM6B
|
1.30102056
|
7.554873441
|
5.23789948
|
1.46E-05
|
9.70E-05
|
2.822985149
|
NUDC
|
1.299825767
|
10.1129032
|
9.124297995
|
7.13E-10
|
5.57E-08
|
12.65298921
|
PHB
|
1.299280352
|
9.036698753
|
8.34467426
|
4.52E-09
|
2.00E-07
|
10.82473593
|
USP1
|
1.299122208
|
5.712328518
|
6.903628133
|
1.69E-07
|
2.81E-06
|
7.233951504
|
UGCG
|
1.299100428
|
7.637255196
|
6.564098659
|
4.11E-07
|
5.59E-06
|
6.352299401
|
NUP88
|
1.298049189
|
7.778731314
|
11.63242519
|
3.19E-12
|
1.26E-09
|
17.97404068
|
LEPROT
|
1.297113978
|
11.60207857
|
4.961828441
|
3.10E-05
|
0.000180137
|
2.080928206
|
SORD
|
-1.296373291
|
9.712182519
|
-3.408042698
|
0.002004522
|
0.00576643
|
-1.971684138
|
RNASEH1
|
1.296211957
|
8.062974187
|
7.743815272
|
1.98E-08
|
5.73E-07
|
9.359509858
|
BGN
|
-1.293749031
|
10.83426956
|
-5.497336355
|
7.19E-06
|
5.53E-05
|
3.520088593
|
EPB41L1
|
-1.293565904
|
8.131970301
|
-8.910275853
|
1.18E-09
|
7.65E-08
|
12.15942346
|
NT5DC2
|
1.290854598
|
7.006657669
|
5.49509333
|
7.23E-06
|
5.55E-05
|
3.514067608
|
EIF5
|
1.290509517
|
9.992535565
|
5.777169499
|
3.37E-06
|
3.01E-05
|
4.26981525
|
JUND
|
-1.288819693
|
11.17357526
|
-13.61921949
|
7.38E-14
|
1.17E-10
|
21.62784642
|
PLK2
|
1.287415552
|
7.339410911
|
5.759288171
|
3.53E-06
|
3.14E-05
|
4.222009283
|
M6PR
|
1.286522924
|
7.51082701
|
7.771500991
|
1.85E-08
|
5.49E-07
|
9.428066888
|
DHRS7B
|
1.286003609
|
9.123234779
|
8.514469828
|
3.01E-09
|
1.48E-07
|
11.23001323
|
TCF7L1
|
-1.284417857
|
9.471134171
|
-6.21840816
|
1.03E-06
|
1.16E-05
|
5.443338468
|
TMEM140
|
1.284344829
|
9.254354193
|
10.94233816
|
1.31E-11
|
3.20E-09
|
16.59303621
|
CYP27B1
|
-1.28385958
|
8.032205684
|
-5.997251595
|
1.86E-06
|
1.87E-05
|
4.856749376
|
KRAS
|
1.281495706
|
8.377790663
|
6.362344392
|
7.01E-07
|
8.55E-06
|
5.823074564
|
GMFG
|
1.279945687
|
9.288317601
|
7.459463711
|
4.06E-08
|
9.68E-07
|
8.64973168
|
PSMA3
|
1.279565875
|
10.16490647
|
5.83933803
|
2.85E-06
|
2.64E-05
|
4.435894253
|
CNOT2
|
1.279300816
|
8.782442522
|
6.434254514
|
5.79E-07
|
7.35E-06
|
6.01212757
|
KLC1
|
1.277054189
|
9.104980272
|
7.524681574
|
3.44E-08
|
8.56E-07
|
8.813423521
|
PLPPR1
|
-1.276165848
|
8.240622771
|
-3.816088742
|
0.000688405
|
0.002344447
|
-0.945391009
|
ABCG1
|
1.275777921
|
7.186008684
|
6.811441439
|
2.15E-07
|
3.37E-06
|
6.995753313
|
DAZAP2
|
1.274142925
|
9.739018005
|
7.637559175
|
2.59E-08
|
7.01E-07
|
9.095478672
|
ERLIN2
|
-1.273846883
|
9.305200067
|
-7.370850264
|
5.08E-08
|
1.14E-06
|
8.426477123
|
GC
|
-1.272445074
|
6.332951466
|
-2.524545429
|
0.017546408
|
0.036095513
|
-4.004098064
|
RNF13
|
1.268534955
|
10.26760703
|
5.355043954
|
1.06E-05
|
7.48E-05
|
3.137888261
|
COL1A2
|
1.266974585
|
7.179573515
|
3.501641194
|
0.001573104
|
0.004681133
|
-1.740031084
|
ZC3H15
|
1.266150743
|
9.172149178
|
5.200299793
|
1.61E-05
|
0.000105414
|
2.721890801
|
KMT5B
|
1.265913904
|
8.649285188
|
7.46494521
|
4.00E-08
|
9.61E-07
|
8.663510146
|
PUM1
|
1.263073454
|
8.134411951
|
6.955641137
|
1.48E-07
|
2.55E-06
|
7.367939869
|
ADD3
|
1.258422615
|
8.997988364
|
5.947988367
|
2.12E-06
|
2.08E-05
|
4.725622462
|
KPNA2
|
1.255126625
|
8.907075287
|
6.720095976
|
2.73E-07
|
4.03E-06
|
6.758843409
|
HLA-DMA
|
1.254424027
|
10.37397051
|
5.785073702
|
3.29E-06
|
2.96E-05
|
4.290942076
|
THEMIS2
|
1.253798071
|
7.019422688
|
6.789113515
|
2.28E-07
|
3.53E-06
|
6.937924834
|
GNL3L
|
1.252325393
|
7.124510447
|
10.81838227
|
1.69E-11
|
3.89E-09
|
16.33847971
|
PSMB4
|
1.249957625
|
10.72159802
|
9.265970538
|
5.14E-10
|
4.44E-08
|
12.97622687
|
AGMAT
|
-1.248270761
|
10.8536785
|
-3.824270959
|
0.000673611
|
0.002304987
|
-0.924415444
|
GIPC2
|
-1.247317538
|
7.723359098
|
-4.309932572
|
0.000182827
|
0.000781047
|
0.341387838
|
DHX9
|
1.246849962
|
6.351974134
|
7.128339548
|
9.45E-08
|
1.81E-06
|
7.810652181
|
EWSR1
|
1.246648172
|
8.535866582
|
10.08327007
|
8.21E-11
|
1.24E-08
|
14.78704752
|
SETD5
|
1.245898909
|
7.482287092
|
7.89652774
|
1.36E-08
|
4.40E-07
|
9.736427478
|
EIF4G1
|
1.244109972
|
7.486954023
|
8.936556557
|
1.10E-09
|
7.39E-08
|
12.22037107
|
SRSF3
|
1.243986139
|
9.803111657
|
7.286475154
|
6.30E-08
|
1.34E-06
|
8.21301214
|
ILF2
|
1.243442066
|
9.552958146
|
6.68055437
|
3.03E-07
|
4.37E-06
|
6.656023645
|
CYP26B1
|
-1.242450416
|
8.634347541
|
-2.397548309
|
0.02343442
|
0.046015965
|
-4.26720407
|
SERPINE1
|
1.240736407
|
6.725458286
|
4.521128924
|
0.000103025
|
0.000486376
|
0.901546668
|
PSMD4
|
1.240423225
|
10.38557871
|
10.02544818
|
9.32E-11
|
1.33E-08
|
14.66193831
|
PDLIM3
|
1.239425245
|
7.796445864
|
8.153301921
|
7.21E-09
|
2.81E-07
|
10.36327807
|
TMEM87A
|
1.238174918
|
8.636838914
|
8.683893986
|
2.01E-09
|
1.12E-07
|
11.63048026
|
PRRC2C
|
1.238003417
|
7.756011399
|
7.200125227
|
7.86E-08
|
1.58E-06
|
7.993668362
|
SNX4
|
1.237495753
|
7.321680798
|
7.530106662
|
3.39E-08
|
8.48E-07
|
8.827016208
|
NRP1
|
1.236313178
|
9.129569229
|
5.883209216
|
2.53E-06
|
2.39E-05
|
4.552965336
|
WAC
|
1.235001654
|
7.03036538
|
7.197894999
|
7.90E-08
|
1.58E-06
|
7.98799151
|
SASH1
|
1.234739955
|
8.294239034
|
7.261823684
|
6.71E-08
|
1.40E-06
|
8.15048352
|
TAP1
|
1.234734308
|
9.251835212
|
8.342764658
|
4.55E-09
|
2.01E-07
|
10.82015571
|
CHMP2A
|
1.234514701
|
10.38085677
|
5.726260811
|
3.86E-06
|
3.38E-05
|
4.133668805
|
ARPC1B
|
1.23312388
|
9.847594921
|
6.629826451
|
3.46E-07
|
4.86E-06
|
6.523885973
|
C1QA
|
1.232874166
|
7.267276992
|
3.49242546
|
0.001611225
|
0.004776231
|
-1.762948256
|
ZFPM2
|
-1.231930712
|
8.037949772
|
-5.308203625
|
1.20E-05
|
8.29E-05
|
3.011991311
|
APLP2
|
1.231910612
|
10.32603877
|
7.606109272
|
2.80E-08
|
7.39E-07
|
9.017054163
|
UTP14A
|
1.231347458
|
7.661719061
|
7.121605911
|
9.62E-08
|
1.84E-06
|
7.793454173
|
CAT
|
1.229093195
|
8.87973752
|
6.872999304
|
1.83E-07
|
2.99E-06
|
7.15491194
|
TIA1
|
1.227747542
|
7.247584903
|
9.010951208
|
9.29E-10
|
6.61E-08
|
12.39238301
|
NAGK
|
1.22754899
|
9.926020105
|
10.36793433
|
4.42E-11
|
8.00E-09
|
15.39638877
|
TM9SF1
|
1.227465595
|
8.623773028
|
8.253859905
|
5.64E-09
|
2.34E-07
|
10.60636978
|
GLG1
|
1.226621678
|
9.826971817
|
5.632513248
|
4.98E-06
|
4.12E-05
|
3.882644978
|
XPNPEP1
|
1.225389014
|
8.466825777
|
7.86771117
|
1.46E-08
|
4.60E-07
|
9.665536137
|
HYAL2
|
1.22355501
|
9.281267332
|
6.500981316
|
4.86E-07
|
6.40E-06
|
6.18713754
|
ASF1A
|
1.223294692
|
7.739507727
|
7.51950823
|
3.48E-08
|
8.66E-07
|
8.80045816
|
WARS
|
1.221822477
|
9.608411649
|
7.31487298
|
5.86E-08
|
1.27E-06
|
8.284953181
|
TMCO3
|
1.22011721
|
9.102616418
|
4.979257633
|
2.95E-05
|
0.000173536
|
2.127743623
|
KTN1
|
1.218156632
|
6.898784102
|
5.40406927
|
9.26E-06
|
6.72E-05
|
3.269620769
|
NNT
|
1.215568091
|
6.952880722
|
5.386246015
|
9.72E-06
|
6.96E-05
|
3.221734225
|
XPNPEP2
|
-1.215559549
|
8.851730714
|
-3.325159474
|
0.002480356
|
0.006893212
|
-2.174682385
|
FLRT3
|
1.210792751
|
10.14839181
|
4.168211768
|
0.000268176
|
0.001070866
|
-0.031686858
|
FADS3
|
1.209980177
|
7.375563699
|
8.103163063
|
8.15E-09
|
3.06E-07
|
10.24156454
|
ENO1
|
1.209953356
|
7.883385651
|
6.223548255
|
1.01E-06
|
1.15E-05
|
5.45692833
|
HLA-F
|
1.209909743
|
11.83477086
|
8.124091232
|
7.74E-09
|
2.96E-07
|
10.29240912
|
PTPRC
|
1.208351307
|
5.832030673
|
8.047207456
|
9.35E-09
|
3.38E-07
|
10.10533478
|
SYT1
|
-1.208255658
|
7.30889895
|
-4.705882758
|
6.23E-05
|
0.000319624
|
1.394681992
|
COMT
|
1.206977299
|
9.312304131
|
7.752820425
|
1.94E-08
|
5.66E-07
|
9.381819803
|
PLS1
|
-1.206303455
|
8.261543055
|
-5.496977729
|
7.19E-06
|
5.53E-05
|
3.519125937
|
ITGB2
|
1.205909695
|
7.91890843
|
5.254326121
|
1.39E-05
|
9.37E-05
|
2.867149862
|
SMARCE1
|
1.205666484
|
8.972747998
|
7.373668132
|
5.04E-08
|
1.13E-06
|
8.433591315
|
SEC14L1
|
1.205033056
|
7.939442936
|
6.08339441
|
1.48E-06
|
1.55E-05
|
5.085651874
|
POSTN
|
1.201856865
|
10.82658185
|
3.379886179
|
0.00215532
|
0.006130585
|
-2.040877409
|
DEFB1
|
-1.200644494
|
10.99447884
|
-3.02989907
|
0.005223435
|
0.012932645
|
-2.879463619
|
TYRP1
|
-1.200351272
|
6.207994431
|
-3.539545898
|
0.001425307
|
0.004302374
|
-1.645531253
|
IMP3
|
1.199878035
|
9.403506224
|
8.37029393
|
4.25E-09
|
1.92E-07
|
10.88613742
|
SMARCA2
|
1.199515299
|
6.947329006
|
7.267526219
|
6.61E-08
|
1.38E-06
|
8.164954491
|
AKAP8L
|
1.199316813
|
6.916908369
|
7.807966077
|
1.69E-08
|
5.14E-07
|
9.518212724
|
PSMD13
|
1.198610121
|
8.052040062
|
10.25115059
|
5.69E-11
|
9.46E-09
|
15.14772671
|
COPS8
|
1.1970076
|
9.204275007
|
7.044000749
|
1.17E-07
|
2.13E-06
|
7.594871427
|
AKAP13
|
1.19666266
|
7.792140231
|
6.987365814
|
1.36E-07
|
2.38E-06
|
7.449517889
|
RRAD
|
1.196066763
|
6.953293655
|
7.077275531
|
1.08E-07
|
2.01E-06
|
7.680102108
|
PLEKHO1
|
1.195459638
|
7.723639306
|
7.159751859
|
8.72E-08
|
1.70E-06
|
7.89081119
|
FOLR1
|
-1.193436488
|
6.956916716
|
-3.734922458
|
0.000853477
|
0.002814152
|
-1.152704059
|
PPIC
|
1.192607632
|
8.477886659
|
6.960597292
|
1.46E-07
|
2.53E-06
|
7.380691668
|
SNX2
|
1.192127734
|
8.610500524
|
6.157301546
|
1.21E-06
|
1.33E-05
|
5.281621255
|
MBD4
|
1.185770843
|
8.009202546
|
7.069175367
|
1.10E-07
|
2.03E-06
|
7.659365794
|
AP3D1
|
1.18500723
|
8.6135603
|
7.725903422
|
2.07E-08
|
5.94E-07
|
9.315102851
|
HBB
|
1.184947119
|
11.93377932
|
3.343143234
|
0.002368653
|
0.006631573
|
-2.130813886
|
RNF114
|
1.183206283
|
9.30418479
|
9.465288756
|
3.26E-10
|
3.26E-08
|
13.42629734
|
ABHD17A
|
1.181106471
|
9.170196843
|
8.329715797
|
4.69E-09
|
2.06E-07
|
10.78884453
|
LRRC42
|
1.180209467
|
7.573503367
|
8.941836312
|
1.09E-09
|
7.34E-08
|
12.23260386
|
DDAH1
|
-1.180008114
|
11.46433716
|
-5.151767722
|
1.84E-05
|
0.000117466
|
2.591402158
|
TSC22D3
|
1.179816234
|
8.262843013
|
4.224652868
|
0.000230275
|
0.000942027
|
0.116582968
|
GYG1
|
1.177529499
|
9.834468492
|
6.016373152
|
1.77E-06
|
1.80E-05
|
4.907603688
|
UQCRC2
|
1.176924599
|
9.899057439
|
7.23706376
|
7.15E-08
|
1.47E-06
|
8.087606913
|
FKBP11
|
1.176919235
|
8.727123226
|
6.266120256
|
9.06E-07
|
1.04E-05
|
5.569402926
|
TM4SF1
|
1.176815697
|
8.331708401
|
5.239153764
|
1.45E-05
|
9.68E-05
|
2.826357468
|
AHCYL1
|
1.176691914
|
8.745386211
|
5.330391797
|
1.13E-05
|
7.91E-05
|
3.071632149
|
SLC28A1
|
-1.176464801
|
6.772070338
|
-8.212773139
|
6.23E-09
|
2.52E-07
|
10.50720966
|
LGALS3
|
1.176104581
|
9.941755013
|
7.549802161
|
3.23E-08
|
8.21E-07
|
8.876332784
|
CAVIN2
|
1.174754505
|
6.982038785
|
5.495846891
|
7.22E-06
|
5.54E-05
|
3.516090419
|
SERPINH1
|
1.174275681
|
9.648430496
|
6.407521754
|
6.22E-07
|
7.79E-06
|
5.941900078
|
ACTA2
|
1.173648029
|
10.38204081
|
5.932733912
|
2.21E-06
|
2.15E-05
|
4.684987509
|
HNRNPA2B1
|
1.173592582
|
11.06475641
|
9.665793594
|
2.07E-10
|
2.28E-08
|
13.87352619
|
AP1S2
|
1.172535127
|
8.523906337
|
5.252624432
|
1.40E-05
|
9.41E-05
|
2.862574763
|
PNN
|
1.17087381
|
7.970795555
|
7.747188801
|
1.97E-08
|
5.70E-07
|
9.367868879
|
HLA-C
|
1.169664398
|
10.16761106
|
7.595608208
|
2.88E-08
|
7.54E-07
|
8.990840477
|
PRPF4B
|
1.169551009
|
6.935475927
|
5.67669604
|
4.42E-06
|
3.75E-05
|
4.000998961
|
USP48
|
-1.168630772
|
9.038096545
|
-7.747244509
|
1.97E-08
|
5.70E-07
|
9.368006903
|
MCM7
|
1.168505141
|
8.351995021
|
8.050385686
|
9.28E-09
|
3.36E-07
|
10.11308366
|
TUG1
|
1.168335892
|
9.165666062
|
6.054292701
|
1.60E-06
|
1.66E-05
|
5.008378733
|
CASP4
|
1.167446728
|
7.679803702
|
10.35532363
|
4.54E-11
|
8.03E-09
|
15.36962558
|
FOXO3
|
1.167411973
|
8.567526997
|
5.725633219
|
3.87E-06
|
3.38E-05
|
4.131989636
|
VAMP2
|
-1.166211897
|
7.442364851
|
-7.873871112
|
1.43E-08
|
4.56E-07
|
9.680699271
|
PDIA6
|
1.165260403
|
10.67248004
|
5.899377478
|
2.42E-06
|
2.31E-05
|
4.596082594
|
DDOST
|
1.164865434
|
10.9394614
|
6.470185623
|
5.27E-07
|
6.83E-06
|
6.10641779
|
PYCARD
|
1.163566225
|
7.46929959
|
5.864762597
|
2.66E-06
|
2.50E-05
|
4.503753551
|
ZNF24
|
1.162861739
|
7.210438195
|
7.357297992
|
5.26E-08
|
1.17E-06
|
8.392248586
|
TYRO3
|
1.161757961
|
8.189575049
|
5.5953718
|
5.51E-06
|
4.46E-05
|
3.783092394
|
ADH6
|
-1.16166635
|
8.207644366
|
-3.436207702
|
0.001863907
|
0.005412229
|
-1.902237878
|
ACSL3
|
1.16124775
|
8.408959315
|
3.546281515
|
0.001400494
|
0.004237233
|
-1.628698861
|
PARP2
|
1.161143625
|
8.581427075
|
11.19875359
|
7.68E-12
|
2.21E-09
|
17.11328056
|
MCCC2
|
-1.160816294
|
10.45719644
|
-5.897428416
|
2.43E-06
|
2.32E-05
|
4.590885687
|
ZNF22
|
1.159131186
|
7.261181636
|
12.5812987
|
5.00E-13
|
4.05E-10
|
19.77645675
|
RALBP1
|
1.158724753
|
8.274230454
|
7.859219723
|
1.49E-08
|
4.66E-07
|
9.644625716
|
NUP85
|
1.156176785
|
8.422135666
|
9.538080515
|
2.77E-10
|
2.81E-08
|
13.5892997
|
CPD
|
1.156112532
|
6.819716927
|
8.154881627
|
7.18E-09
|
2.81E-07
|
10.36710738
|
HCP5
|
1.155541743
|
8.967916149
|
5.892526241
|
2.47E-06
|
2.34E-05
|
4.577813709
|
AGTR1
|
1.155149645
|
6.968562585
|
5.121576314
|
2.00E-05
|
0.000125603
|
2.510230811
|
LIN37
|
1.155105526
|
6.577595527
|
9.667828073
|
2.06E-10
|
2.28E-08
|
13.87803581
|
CCT6A
|
1.154913313
|
9.14834035
|
7.235166054
|
7.18E-08
|
1.48E-06
|
8.08278478
|
HNRNPC
|
1.154717025
|
8.873047157
|
6.813932366
|
2.13E-07
|
3.35E-06
|
7.002201462
|
SLC13A1
|
-1.15376563
|
7.5476979
|
-3.18681201
|
0.003526296
|
0.009294589
|
-2.508681404
|
VAMP3
|
1.152730555
|
7.405947549
|
4.957816762
|
3.13E-05
|
0.000181512
|
2.070153776
|
MAP4K3
|
-1.150486671
|
7.339441604
|
-6.152728191
|
1.23E-06
|
1.34E-05
|
5.269506464
|
JMJD6
|
1.150291091
|
7.191119689
|
12.55420458
|
5.27E-13
|
4.05E-10
|
19.726484
|
HLA-DPB1
|
1.150218342
|
11.36426215
|
5.525706581
|
6.65E-06
|
5.21E-05
|
3.596230432
|
SRRM1
|
-1.149363305
|
9.137180405
|
-5.391189648
|
9.59E-06
|
6.88E-05
|
3.235017125
|
RRAGC
|
1.149352733
|
8.837854366
|
6.475336686
|
5.20E-07
|
6.75E-06
|
6.119925528
|
ZNF804A
|
-1.149172983
|
8.504688414
|
-5.863390141
|
2.67E-06
|
2.50E-05
|
4.500091338
|
PSME3
|
-1.148471143
|
9.262437167
|
-5.79437545
|
3.21E-06
|
2.90E-05
|
4.315800219
|
RBP4
|
-1.148117964
|
8.285701455
|
-2.430483745
|
0.021754392
|
0.043227525
|
-4.199808903
|
NMD3
|
1.147678273
|
7.096738478
|
5.993365837
|
1.88E-06
|
1.89E-05
|
4.846412134
|
PSMB9
|
1.144615141
|
10.39353049
|
6.638465727
|
3.38E-07
|
4.76E-06
|
6.546407914
|
SYNCRIP
|
1.144133474
|
7.533673599
|
5.254346869
|
1.39E-05
|
9.37E-05
|
2.867205645
|
YWHAH
|
1.142268582
|
8.609758108
|
3.448365406
|
0.001806203
|
0.005267323
|
-1.872190252
|
DNAJC8
|
1.141702145
|
9.890908337
|
12.41268851
|
6.90E-13
|
4.67E-10
|
19.46406954
|
ARF4
|
1.141135381
|
9.861513946
|
4.629461092
|
7.67E-05
|
0.000380207
|
1.190413168
|
CYP1B1
|
1.139251767
|
8.983971841
|
6.038927934
|
1.66E-06
|
1.71E-05
|
4.967557162
|
FGL2
|
1.139176467
|
10.00389177
|
5.396769049
|
9.45E-06
|
6.81E-05
|
3.250007688
|
TAX1BP1
|
1.137965
|
10.06609394
|
7.996955328
|
1.06E-08
|
3.69E-07
|
9.982636271
|
DNAJC7
|
1.136922569
|
8.975904344
|
5.350564296
|
1.07E-05
|
7.55E-05
|
3.125849226
|
SNRPB
|
1.13575687
|
10.70918168
|
8.092302578
|
8.37E-09
|
3.11E-07
|
10.21515617
|
WT1
|
1.132916354
|
9.05457011
|
4.948629513
|
3.21E-05
|
0.000185305
|
2.045480572
|
SLC13A3
|
-1.132662093
|
10.63519462
|
-3.356064493
|
0.002291407
|
0.006445268
|
-2.099233019
|
EFNB2
|
1.132430918
|
8.078269009
|
5.104816376
|
2.09E-05
|
0.000130601
|
2.465173592
|
GATM
|
-1.132347483
|
9.378659276
|
-4.820073926
|
4.56E-05
|
0.000246052
|
1.700515384
|
CNIH4
|
1.131683601
|
8.479595575
|
5.273080806
|
1.32E-05
|
8.97E-05
|
2.917571743
|
EPHX1
|
-1.131399427
|
8.499935775
|
-2.800395449
|
0.00915647
|
0.020779471
|
-3.404434478
|
SLC12A1
|
-1.131269099
|
9.982695474
|
-3.262062692
|
0.002913818
|
0.007915227
|
-2.327789665
|
DDX18
|
1.12754023
|
7.44385403
|
6.968940574
|
1.43E-07
|
2.48E-06
|
7.402152136
|
ALAS1
|
1.125667398
|
9.259205057
|
10.4312198
|
3.86E-11
|
7.26E-09
|
15.5303756
|
PIPOX
|
-1.123197227
|
9.810383723
|
-3.323828884
|
0.002488819
|
0.006915008
|
-2.177924155
|
TMEM204
|
1.123075986
|
10.94367823
|
5.734623996
|
3.78E-06
|
3.30E-05
|
4.156043341
|
IFI16
|
1.121716104
|
6.377629496
|
6.221519431
|
1.02E-06
|
1.15E-05
|
5.451564585
|
FDPS
|
1.121519611
|
9.476641456
|
5.462769647
|
7.89E-06
|
5.94E-05
|
3.427285292
|
ADSL
|
1.120968528
|
9.354870139
|
11.7975847
|
2.29E-12
|
1.13E-09
|
18.29564463
|
C21orf59
|
1.120241495
|
9.349721825
|
8.079662341
|
8.63E-09
|
3.19E-07
|
10.18440035
|
PLXDC2
|
1.119961822
|
6.674408432
|
6.694948461
|
2.91E-07
|
4.25E-06
|
6.693470854
|
IK
|
1.119516839
|
11.08344752
|
11.17279646
|
8.10E-12
|
2.26E-09
|
17.06100157
|
EPB41L5
|
1.119432184
|
8.719882514
|
5.112636291
|
2.05E-05
|
0.000128284
|
2.486196259
|
PAPOLA
|
1.11940591
|
9.203346945
|
6.556215488
|
4.20E-07
|
5.67E-06
|
6.331691821
|
BABAM1
|
1.118621088
|
9.799168898
|
13.13465146
|
1.78E-13
|
2.09E-10
|
20.77854969
|
EIF3A
|
1.118546997
|
8.851059563
|
7.373548512
|
5.05E-08
|
1.13E-06
|
8.433289332
|
10-Sep
|
1.11477609
|
7.162778757
|
5.1308438
|
1.95E-05
|
0.000123054
|
2.535146373
|
METTL3
|
1.114576495
|
9.512185519
|
10.75712554
|
1.93E-11
|
4.32E-09
|
16.21193997
|
RYBP
|
1.114362488
|
8.074681305
|
5.558649075
|
6.08E-06
|
4.84E-05
|
3.684612117
|
SRSF7
|
1.114068517
|
7.812810523
|
6.78434274
|
2.31E-07
|
3.57E-06
|
6.925561917
|
SPCS2
|
1.113642182
|
10.67916436
|
10.02665475
|
9.29E-11
|
1.33E-08
|
14.66455361
|
IL6ST
|
1.112439922
|
7.249378474
|
5.2191725
|
1.53E-05
|
0.000101131
|
2.772634323
|
CASP3
|
1.112233366
|
7.060212477
|
6.02808801
|
1.71E-06
|
1.75E-05
|
4.938747575
|
SLC22A8
|
-1.111645351
|
8.172587127
|
-2.906495713
|
0.007078547
|
0.016675257
|
-3.164457325
|
SLC34A1
|
-1.111436435
|
8.827172212
|
-3.462040019
|
0.001743365
|
0.005105454
|
-1.838343558
|
SLCO3A1
|
1.110078679
|
7.643092094
|
5.674934307
|
4.44E-06
|
3.76E-05
|
3.996281323
|
H2AFZ
|
1.109787786
|
10.36972324
|
7.309395678
|
5.94E-08
|
1.29E-06
|
8.271084918
|
TRAM1
|
1.109674164
|
9.330891829
|
5.475848882
|
7.62E-06
|
5.78E-05
|
3.462403688
|
CPM
|
1.108350817
|
6.344952054
|
4.511146811
|
0.000105862
|
0.000497192
|
0.874975993
|
SF3B4
|
-1.106855958
|
8.447412686
|
-11.66922686
|
2.96E-12
|
1.22E-09
|
18.04599558
|
GPKOW
|
1.105821203
|
9.402386711
|
15.05894645
|
6.17E-15
|
2.61E-11
|
24.00537577
|
COMMD4
|
1.105582864
|
8.633374558
|
9.573721267
|
2.55E-10
|
2.65E-08
|
13.66884399
|
NKTR
|
1.105103578
|
7.436472493
|
6.31627032
|
7.93E-07
|
9.41E-06
|
5.701708637
|
GZMB
|
1.104615964
|
6.213304274
|
4.943201366
|
3.26E-05
|
0.000187539
|
2.030903852
|
PRKAR1A
|
1.103349619
|
9.085496498
|
6.930113803
|
1.58E-07
|
2.69E-06
|
7.302217123
|
GAR1
|
1.103322681
|
8.867905942
|
11.69566488
|
2.81E-12
|
1.22E-09
|
18.09758328
|
PCMT1
|
1.10293129
|
9.090122013
|
8.57858399
|
2.58E-09
|
1.32E-07
|
11.38202189
|
OSBPL1A
|
1.101591227
|
7.294578344
|
6.029552935
|
1.71E-06
|
1.75E-05
|
4.942641418
|
CSNK1A1
|
1.101429726
|
9.136865726
|
8.271107725
|
5.41E-09
|
2.27E-07
|
10.64792844
|
FRY
|
1.101325533
|
7.416761028
|
4.840073415
|
4.32E-05
|
0.000235359
|
1.75414204
|
ETS2
|
1.10079836
|
9.955899787
|
6.80950633
|
2.16E-07
|
3.38E-06
|
6.990743531
|
C1QBP
|
1.099436575
|
9.443174021
|
7.90432057
|
1.33E-08
|
4.35E-07
|
9.755579866
|
CAMK1
|
1.098714967
|
7.277320139
|
8.659802769
|
2.12E-09
|
1.16E-07
|
11.57377593
|
TIGAR
|
1.098198376
|
7.2916035
|
5.49588839
|
7.21E-06
|
5.54E-05
|
3.516201816
|
ELF1
|
1.097652061
|
9.011134077
|
8.423523203
|
3.74E-09
|
1.74E-07
|
11.01342471
|
PPFIBP1
|
1.096172641
|
6.181551302
|
6.404621461
|
6.27E-07
|
7.83E-06
|
5.934277118
|
TCF25
|
1.096054149
|
8.091775108
|
6.227690486
|
1.00E-06
|
1.13E-05
|
5.467878422
|
CDC27
|
1.095817747
|
8.505153326
|
4.91940844
|
3.48E-05
|
0.000197882
|
1.967020368
|
SLA
|
1.094863831
|
8.203268999
|
6.487175988
|
5.04E-07
|
6.58E-06
|
6.150962717
|
NEU1
|
1.093178847
|
8.947170302
|
7.96855995
|
1.13E-08
|
3.91E-07
|
9.913156724
|
MTDH
|
1.092673248
|
9.333952516
|
4.191473779
|
0.000251861
|
0.001016156
|
0.029369908
|
RALYL
|
-1.091884713
|
8.179522241
|
-3.770225925
|
0.0007774
|
0.002597903
|
-1.062705647
|
EGR2
|
-1.090102058
|
6.469861827
|
-4.641895549
|
7.41E-05
|
0.000369589
|
1.223623989
|
SELENOW
|
1.089407334
|
10.83413383
|
8.564446583
|
2.67E-09
|
1.35E-07
|
11.34855174
|
DHX35
|
1.088913326
|
7.875257997
|
8.366012273
|
4.30E-09
|
1.94E-07
|
10.87588196
|
H2AFY
|
1.088300954
|
8.945648275
|
7.534738013
|
3.35E-08
|
8.41E-07
|
8.838617254
|
FEZ2
|
1.088132355
|
9.829802339
|
6.601202262
|
3.73E-07
|
5.13E-06
|
6.449212407
|
CXCL1
|
-1.08797836
|
7.890182363
|
-3.583978395
|
0.001269183
|
0.003906784
|
-1.534276712
|
TUBG1
|
1.086695059
|
8.745692451
|
9.151344631
|
6.70E-10
|
5.33E-08
|
12.71491244
|
EGFR
|
1.086214902
|
6.754578363
|
6.745928219
|
2.55E-07
|
3.84E-06
|
6.82592863
|
DERL1
|
1.086001096
|
6.89012709
|
6.279613636
|
8.74E-07
|
1.01E-05
|
5.605021567
|
CAV1
|
1.084584181
|
7.441861966
|
7.66312002
|
2.43E-08
|
6.70E-07
|
9.159125496
|
IDH3G
|
1.082946039
|
9.572242915
|
4.996077021
|
2.82E-05
|
0.000166977
|
2.172927883
|
DDIT4
|
1.082694616
|
9.431563963
|
4.287981287
|
0.000194025
|
0.000818704
|
0.283437599
|
MT1G
|
-1.082597887
|
12.96743712
|
-5.150025681
|
1.85E-05
|
0.000117877
|
2.586718443
|
TUBB3
|
1.081470203
|
10.81289795
|
9.271887653
|
5.07E-10
|
4.40E-08
|
12.98966696
|
EFHC1
|
1.08144924
|
8.498565098
|
9.503313964
|
2.99E-10
|
3.03E-08
|
13.51153807
|
MPHOSPH8
|
1.081120204
|
8.222430403
|
6.647821917
|
3.30E-07
|
4.67E-06
|
6.570790457
|
SLC39A6
|
1.080520811
|
8.612275262
|
5.321066886
|
1.16E-05
|
8.09E-05
|
3.046567941
|
GMPR2
|
1.080419808
|
10.1835269
|
9.982494926
|
1.02E-10
|
1.40E-08
|
14.56870652
|
PLPP1
|
1.079549675
|
12.22298887
|
4.588629924
|
8.57E-05
|
0.000417251
|
1.081433867
|
C3AR1
|
1.079533021
|
7.464575983
|
4.520362847
|
0.00010324
|
0.000487288
|
0.899507206
|
PKP4
|
1.078988705
|
7.219341697
|
4.508110069
|
0.00010674
|
0.000500419
|
0.86689438
|
SCG5
|
1.078692914
|
6.420091104
|
3.471874341
|
0.001699488
|
0.00500128
|
-1.81396971
|
CNOT8
|
1.078685586
|
8.779292263
|
6.264621562
|
9.09E-07
|
1.05E-05
|
5.565445885
|
MKNK2
|
1.07867287
|
9.762189026
|
11.32712576
|
5.91E-12
|
1.88E-09
|
17.37056083
|
ZNF721
|
1.077745797
|
8.69368259
|
4.543383862
|
9.70E-05
|
0.000462503
|
0.960815239
|
STK32B
|
-1.076764142
|
8.278102207
|
-6.487474074
|
5.03E-07
|
6.58E-06
|
6.151743993
|
DAB2
|
1.076679738
|
9.010118077
|
5.160270103
|
1.80E-05
|
0.000115318
|
2.614262168
|
WDR1
|
1.075060578
|
10.70569774
|
12.48277575
|
6.04E-13
|
4.48E-10
|
19.59432742
|
DDX50
|
1.072849739
|
10.42516752
|
5.249666541
|
1.41E-05
|
9.46E-05
|
2.854622248
|
CLDN3
|
1.07236717
|
6.08859028
|
4.843722356
|
4.27E-05
|
0.000233435
|
1.763928086
|
ZBTB20
|
1.071588517
|
9.17400408
|
4.031253406
|
0.000387637
|
0.001460553
|
-0.389561913
|
ASNA1
|
1.071351132
|
9.062987359
|
4.634014168
|
7.58E-05
|
0.000376438
|
1.202572636
|
COL4A3BP
|
1.069228532
|
7.496292481
|
6.771068462
|
2.39E-07
|
3.67E-06
|
6.891150626
|
DBT
|
1.068894976
|
10.81364504
|
6.479610391
|
5.14E-07
|
6.70E-06
|
6.131130702
|
KLF6
|
1.068595042
|
8.694058607
|
3.923383883
|
0.000517366
|
0.001851367
|
-0.669284058
|
KLHL20
|
1.068504768
|
7.038412418
|
10.21355215
|
6.17E-11
|
9.83E-09
|
15.06727982
|
EED
|
1.06808641
|
8.464217814
|
7.919227461
|
1.28E-08
|
4.24E-07
|
9.792194251
|
MTF2
|
1.067468895
|
7.289425208
|
9.731404613
|
1.79E-10
|
2.08E-08
|
14.01867335
|
USE1
|
1.067373964
|
8.080264229
|
8.78600915
|
1.57E-09
|
9.41E-08
|
11.86994673
|
DIP2C
|
-1.064411186
|
8.287020133
|
-4.950915171
|
3.19E-05
|
0.000184439
|
2.051618708
|
GUCY1A3
|
1.064369955
|
8.391717113
|
7.722954073
|
2.09E-08
|
5.98E-07
|
9.307786878
|
FABP1
|
-1.063475899
|
8.139323754
|
-2.732116105
|
0.010784021
|
0.023903519
|
-3.556239515
|
NMI
|
1.063418627
|
8.913174785
|
6.061674729
|
1.56E-06
|
1.63E-05
|
5.027985704
|
FNDC3B
|
1.062680278
|
7.298362048
|
6.320892845
|
7.83E-07
|
9.33E-06
|
5.713893204
|
C21orf33
|
1.062481266
|
7.988234956
|
3.425838621
|
0.001914528
|
0.005546208
|
-1.92783153
|
LAP3
|
1.062459662
|
10.15323608
|
5.869684864
|
2.62E-06
|
2.47E-05
|
4.516887066
|
HSP90B1
|
1.06186157
|
8.503549506
|
5.777879179
|
3.36E-06
|
3.01E-05
|
4.271712257
|
ING3
|
1.061456364
|
7.638573932
|
4.825058581
|
4.50E-05
|
0.000243541
|
1.71387968
|
C1QB
|
1.061188708
|
6.688390333
|
3.127770653
|
0.004091421
|
0.010523969
|
-2.649244412
|
TMBIM6
|
1.060676668
|
12.20049452
|
4.027508929
|
0.00039155
|
0.001473553
|
-0.39930522
|
GPR161
|
1.059734032
|
6.501724924
|
6.01425427
|
1.78E-06
|
1.81E-05
|
4.901969665
|
NAA15
|
1.057880748
|
6.432962831
|
6.629466788
|
3.46E-07
|
4.86E-06
|
6.522948198
|
SULF1
|
1.05757773
|
9.843063851
|
6.231652537
|
9.93E-07
|
1.12E-05
|
5.478350927
|
WIPI1
|
1.056510973
|
8.27825346
|
6.246765604
|
9.54E-07
|
1.09E-05
|
5.518286309
|
ZBTB38
|
1.056169824
|
7.302859849
|
7.766411617
|
1.87E-08
|
5.53E-07
|
9.415471696
|
CSTF1
|
1.055434663
|
7.337081595
|
10.68718219
|
2.23E-11
|
4.83E-09
|
16.06685271
|
CX3CR1
|
1.055309203
|
8.117188378
|
3.623156632
|
0.001145417
|
0.003583227
|
-1.435762734
|
NFYC
|
1.054756655
|
6.448423523
|
8.20428046
|
6.36E-09
|
2.56E-07
|
10.48668483
|
APOL3
|
1.054037856
|
10.02998628
|
7.311646662
|
5.91E-08
|
1.28E-06
|
8.276784737
|
PALMD
|
1.053740224
|
7.735488403
|
6.012628847
|
1.78E-06
|
1.81E-05
|
4.897647525
|
CHTOP
|
1.052486772
|
7.220378721
|
6.679260814
|
3.04E-07
|
4.38E-06
|
6.652657347
|
SEC11A
|
1.051884002
|
10.29217746
|
4.251936424
|
0.000213903
|
0.000885124
|
0.188406866
|
VAMP5
|
1.050721926
|
10.52172068
|
4.438313791
|
0.00012905
|
0.000584474
|
0.681372633
|
ANKLE2
|
1.05042763
|
7.196105217
|
8.876060405
|
1.27E-09
|
8.10E-08
|
12.07993162
|
CAP1
|
1.050024945
|
10.95712377
|
7.506067683
|
3.61E-08
|
8.86E-07
|
8.766758041
|
P4HB
|
1.049010802
|
10.47300414
|
4.458270788
|
0.000122236
|
0.000558178
|
0.734373822
|
PLEKHA1
|
1.048977766
|
7.19454498
|
7.575881532
|
3.02E-08
|
7.84E-07
|
8.941559368
|
SART1
|
1.047328783
|
8.1137404
|
8.376187797
|
4.19E-09
|
1.90E-07
|
10.90025038
|
ERBB4
|
-1.046679912
|
10.46327143
|
-7.702647823
|
2.20E-08
|
6.23E-07
|
9.257386132
|
CPQ
|
1.046010336
|
10.54802267
|
5.912742818
|
2.33E-06
|
2.24E-05
|
4.631713331
|
FXR1
|
1.045839377
|
7.714915221
|
6.751506073
|
2.51E-07
|
3.80E-06
|
6.840405057
|
NFASC
|
-1.043772901
|
11.00079661
|
-7.995409742
|
1.06E-08
|
3.70E-07
|
9.978857179
|
RBM8A
|
1.043747898
|
7.545132469
|
4.96501949
|
3.07E-05
|
0.00017886
|
2.089498913
|
AATF
|
1.043262199
|
9.350762714
|
7.721186391
|
2.10E-08
|
6.00E-07
|
9.303401537
|
RNF146
|
1.042920236
|
7.254295397
|
5.404368353
|
9.25E-06
|
6.72E-05
|
3.270424275
|
PTK2
|
1.042474572
|
7.441602272
|
7.416573273
|
4.52E-08
|
1.05E-06
|
8.541792951
|
NSFL1C
|
1.042373942
|
7.852911437
|
6.47816252
|
5.16E-07
|
6.71E-06
|
6.127334735
|
FBXW7
|
1.041338605
|
7.484615862
|
5.840734365
|
2.83E-06
|
2.63E-05
|
4.439622073
|
NSDHL
|
1.040858898
|
7.542287566
|
9.071986684
|
8.06E-10
|
6.00E-08
|
12.53293586
|
LSM4
|
1.040785139
|
7.644681813
|
7.310557642
|
5.92E-08
|
1.28E-06
|
8.274027254
|
FBXO9
|
1.038692099
|
7.796898194
|
5.341521258
|
1.10E-05
|
7.71E-05
|
3.101545225
|
PPARG
|
1.037896309
|
6.88201037
|
7.302296857
|
6.05E-08
|
1.30E-06
|
8.253105673
|
GLT8D1
|
1.037533949
|
8.106135801
|
7.178182333
|
8.31E-08
|
1.64E-06
|
7.937789238
|
HMGN4
|
-1.03747284
|
9.403636016
|
-6.593807899
|
3.80E-07
|
5.22E-06
|
6.429909286
|
PTGER4
|
1.037231193
|
6.884584114
|
5.215070337
|
1.55E-05
|
0.000102088
|
2.761604788
|
MED6
|
1.035682324
|
5.366963402
|
7.930582401
|
1.25E-08
|
4.18E-07
|
9.820064745
|
DST
|
1.034952212
|
8.875164537
|
5.651911373
|
4.72E-06
|
3.95E-05
|
3.934617402
|
IFT27
|
1.034430229
|
8.164343121
|
9.736350061
|
1.77E-10
|
2.08E-08
|
14.02958994
|
MTUS1
|
1.033651385
|
7.914456679
|
6.513891394
|
4.69E-07
|
6.21E-06
|
6.220950438
|
ACO2
|
1.033548604
|
10.80339959
|
5.484032163
|
7.45E-06
|
5.68E-05
|
3.484373888
|
SP3
|
-1.031245916
|
9.087202589
|
-3.605371867
|
0.001200071
|
0.003731848
|
-1.480530029
|
VDAC3
|
1.030703765
|
8.940345624
|
6.468148347
|
5.30E-07
|
6.84E-06
|
6.101074729
|
USP39
|
1.029451601
|
8.677413981
|
9.982258621
|
1.02E-10
|
1.40E-08
|
14.56819292
|
CMTM6
|
1.02805724
|
9.525157136
|
4.774766756
|
5.16E-05
|
0.000273084
|
1.579093631
|
XYLT1
|
-1.027723777
|
8.518221821
|
-5.498716221
|
7.16E-06
|
5.52E-05
|
3.523792515
|
ARHGAP19
|
1.02763613
|
9.56426488
|
7.028513894
|
1.22E-07
|
2.19E-06
|
7.55516027
|
POLR2E
|
1.027421342
|
7.924388244
|
3.308300088
|
0.002589674
|
0.00715863
|
-2.215716541
|
BTG2
|
-1.026399219
|
10.23337218
|
-7.052038998
|
1.15E-07
|
2.10E-06
|
7.615472284
|
PRPF19
|
1.026183615
|
8.826847728
|
8.122731764
|
7.77E-09
|
2.96E-07
|
10.2891081
|
ANKRD11
|
1.025729915
|
7.978900966
|
7.642598687
|
2.56E-08
|
6.94E-07
|
9.108033698
|
PSMB10
|
1.024330336
|
9.421279003
|
3.339953151
|
0.002388107
|
0.006680164
|
-2.138602883
|
SRPK2
|
1.024123484
|
9.338732967
|
5.693785151
|
4.22E-06
|
3.62E-05
|
4.04675383
|
COL4A1
|
1.024118647
|
8.992245418
|
5.104326235
|
2.10E-05
|
0.000130711
|
2.46385594
|
NRN1
|
1.024086017
|
9.095218377
|
4.759145776
|
5.38E-05
|
0.000282366
|
1.537252436
|
RSRC2
|
1.023758956
|
8.613657235
|
6.01119626
|
1.79E-06
|
1.82E-05
|
4.893838006
|
RAC1
|
1.0232867
|
11.63257747
|
4.154851659
|
0.000278012
|
0.001103875
|
-0.066719657
|
HADHA
|
1.022601138
|
10.74473959
|
6.326166993
|
7.72E-07
|
9.21E-06
|
5.727793185
|
AUP1
|
1.021101769
|
9.246258609
|
11.719411
|
2.68E-12
|
1.22E-09
|
18.14384431
|
TXNIP
|
1.02026765
|
11.46235662
|
5.062303643
|
2.35E-05
|
0.000143623
|
2.350896021
|
TUBB
|
1.019856466
|
11.1030305
|
5.384868531
|
9.76E-06
|
6.98E-05
|
3.218033023
|
LPL
|
-1.019304987
|
9.954464295
|
-3.318786443
|
0.002521146
|
0.006987401
|
-2.190204228
|
NPR3
|
1.019147185
|
7.765826952
|
6.380158117
|
6.69E-07
|
8.24E-06
|
5.869949575
|
GNA11
|
1.018301382
|
7.135455751
|
3.567078203
|
0.001326501
|
0.00405132
|
-1.576653085
|
ARPC1A
|
1.018110026
|
10.05733879
|
5.427722254
|
8.68E-06
|
6.39E-05
|
3.333160252
|
TSPAN4
|
1.017903455
|
9.153133398
|
3.286599685
|
0.002737228
|
0.007501373
|
-2.268400912
|
HNRNPA3
|
1.017675658
|
7.627507212
|
5.584619489
|
5.67E-06
|
4.57E-05
|
3.75426261
|
JUN
|
-1.01630629
|
10.14683512
|
-8.526608216
|
2.92E-09
|
1.45E-07
|
11.25883533
|
COBL
|
-1.016074994
|
9.359404055
|
-7.163224111
|
8.64E-08
|
1.68E-06
|
7.899664761
|
EIF3B
|
1.015917612
|
7.916407277
|
7.295506558
|
6.16E-08
|
1.32E-06
|
8.235902161
|
COPS6
|
1.01571967
|
10.73984087
|
6.871235397
|
1.84E-07
|
3.00E-06
|
7.150356983
|
PTPRO
|
-1.015461858
|
12.12826016
|
-4.113981359
|
0.000310368
|
0.001211407
|
-0.173730401
|
ZFP36
|
-1.015055803
|
9.582243452
|
-6.92601257
|
1.59E-07
|
2.71E-06
|
7.2916514
|
BUD23
|
1.014932236
|
9.485752254
|
6.221938523
|
1.02E-06
|
1.15E-05
|
5.452672596
|
PBLD
|
-1.014740962
|
10.58094791
|
-3.724016801
|
0.000878415
|
0.002881032
|
-1.180450248
|
SNTB2
|
1.014417654
|
7.961457348
|
6.282527181
|
8.67E-07
|
1.01E-05
|
5.612710524
|
CTDSPL
|
1.01439347
|
10.43635804
|
4.266936434
|
0.000205397
|
0.000857252
|
0.22793438
|
RNF7
|
1.01409318
|
9.590065207
|
9.476640922
|
3.18E-10
|
3.19E-08
|
13.45176623
|
PTPRN2
|
1.013187332
|
9.817821348
|
9.100558946
|
7.54E-10
|
5.75E-08
|
12.59855544
|
ZBTB18
|
-1.012739735
|
9.549857266
|
-6.564108062
|
4.11E-07
|
5.59E-06
|
6.352323976
|
SERINC3
|
1.012252699
|
9.515098643
|
5.004169961
|
2.76E-05
|
0.000164243
|
2.194671288
|
EPHA4
|
1.012059148
|
7.692051937
|
8.221126207
|
6.11E-09
|
2.48E-07
|
10.52738762
|
EXPH5
|
-1.011374294
|
9.267532038
|
-6.395702776
|
6.42E-07
|
7.99E-06
|
5.910831112
|
SLC14A1
|
1.010232523
|
8.216419273
|
4.925155609
|
3.42E-05
|
0.00019565
|
1.982449863
|
AMPH
|
-1.008900528
|
7.734124024
|
-3.684116541
|
0.000975864
|
0.003135087
|
-1.281735767
|
HLA-J
|
1.00871551
|
10.19609554
|
8.597452642
|
2.46E-09
|
1.28E-07
|
11.42665058
|
PRMT5
|
1.007933207
|
9.428092233
|
7.939886786
|
1.22E-08
|
4.11E-07
|
9.842889558
|
PON2
|
1.007800835
|
10.09079366
|
3.81949425
|
0.000682209
|
0.002328987
|
-0.936662463
|
FBXW2
|
1.007654936
|
7.868340028
|
7.861953607
|
1.48E-08
|
4.63E-07
|
9.651359007
|
STX18
|
1.006898222
|
10.37920307
|
10.5061697
|
3.28E-11
|
6.42E-09
|
15.68836623
|
MRPL9
|
1.00575951
|
8.56785435
|
10.39823871
|
4.14E-11
|
7.62E-09
|
15.4606155
|
NCOA1
|
1.005421619
|
9.05825609
|
6.916782293
|
1.63E-07
|
2.76E-06
|
7.267865336
|
EIF3D
|
1.004960463
|
10.76774901
|
9.588105769
|
2.47E-10
|
2.61E-08
|
13.70089836
|
ZNF45
|
1.004939996
|
5.556368284
|
6.771883066
|
2.38E-07
|
3.67E-06
|
6.893262879
|
RBL2
|
1.004173461
|
7.21346767
|
8.632505051
|
2.27E-09
|
1.21E-07
|
11.50942809
|
PTP4A2
|
1.003941839
|
7.140081341
|
8.279182405
|
5.30E-09
|
2.24E-07
|
10.66737061
|
CXADR
|
1.003893604
|
9.64625798
|
3.484927272
|
0.001642901
|
0.004854007
|
-1.781577202
|
TTC37
|
1.001622392
|
7.444769781
|
5.465907256
|
7.83E-06
|
5.91E-05
|
3.435710333
|
ATP6V0E2
|
-1.001198901
|
9.83254098
|
-7.451280242
|
4.14E-08
|
9.82E-07
|
8.629154547
|
UROD
|
1.001043527
|
9.325183612
|
16.03116183
|
1.28E-15
|
9.51E-12
|
25.49653811
|
THSD7A
|
1.001019085
|
9.626288581
|
3.889413906
|
0.00056643
|
0.001995535
|
-0.756941013
|
NFIL3
|
-1.000765264
|
8.473403841
|
-5.219220674
|
1.53E-05
|
0.000101131
|
2.77276385
|
POLR2A
|
-1.000463151
|
8.643383429
|
-6.408934128
|
6.20E-07
|
7.77E-06
|
5.945612006
|