DOI: 10.31038/AWHC.2019245
Abstract
The study investigated consumer responses to message about hair coloring, as one of the ongoing cartographies by Mind Genomics of the world of consumer beauty. Respondents evaluated short, systematically designed combinations of messages about hair coloring (vignettes), these vignettes talking about the rationale for coloring one’s hair, the feelings about changing one’s color, beliefs about the ‘downside’ of hair coloring, and a comparison of different methods for beautifying one’s hair (e.g., coloring versus cutting), respectively. The deconstruction of the vignettes into their components suggests an underlying core of at least three emergent mind-sets (Follow the prescription of others; Coloring is a personal expression; Focus on self-care). The paper presents the PVI, personal viewpoint identifier, to assign new people to one of these three mind-sets, for subsequent use in research or sales.
Introduction
Little of the published literature in ‘experimenting science; is devoted to questions of a ‘more broad nature.’ Most experiments deal with questions about a small sliver of human daily life. A search of the literature of beauty, especially the quotidian use of products and services for daily and ordinary purposes, quickly reveals that there is little in the way of archival scientific literature on study of beauty as a topic, except perhaps in the world of sociology and social ethnography. There is some data on beauty products and services, but not the plethora of information that would be expected, given the important role that beauty plays. The reality of ‘beauty’ as a topic is that it plays a major role in civilizations which have moved beyond the subsistence stage.
If one were to comment on today’s information about consumers and beauty, it would be almost impossible to assemble a world of scientific papers on beauty, and indeed nothing in comparison to the massive wealth of written material about beauty from the point of view of people and situations. The information, often purporting to be from scientific laboratories but presented in a high style, ‘glitzy’ fashion, would have us believe that we can be the masters of beauty, controlling it for our own uses.
A search through the literature of beauty suggests very little serious information regarding the way people think about beauty products and beauty services, especially hair, except for the most superficial information. We deal here with one topic, the psychological consideration of hair coloring, a topic which suffers a death of information, other than the insistent packages of home hair color, and occupying a lot of ‘trade real estate’ to feature the different colors. The reason for such dearth of information may lie in the fact that coloring one’s hair is not considered to be a topic of major scientific interest, nor in fact is it, when presented in such sterile terms. The reality is that the entire spectrum of behavior with respect to hair is typically considered from one of two rather distinct areas, neither of which deals with the deep psychology of cosmetics as one would have thought from the popular press:
- Hair specifically, then skin, as a substrate for the science of the product, usually studied by chemists. Hair products in general and hair coloring in particular, enjoy a reasonable number of papers in the world of chemistry, noticeably cosmetic chemistry, some of which appear in journals dealing with cosmetics from the point of view of science. The articles in this world deal with the science of the physical product, the performance, and the interaction with the substrate, namely the human body. The topics in journals dealing with these aspects of cosmetic chemistry can scarcely be distinguished in their manner of presentation from topics of in other chemistry journals; namely chemistry first, cosmetics second, and the human experience scarcely considered, if at all e.g., Trueb 2005 [1].
- Beauty as a subject of behavior, primarily social behavior. The objective of such study is ethnographic, looking at behaviors of normal people in society, as a reflection of the society and its mores. In other words, cosmetics as a topic in anthropology, namely the person’s search for (Bloch, 1993; Cash 1987; Graham & Jouhar, 1981) [2,3,4].
The World of Hair Coloring
Hair coloring in the salon has excited the interest of researchers, not just because of its fundamental behavior in the world of beauty but rather it is an aspect of behavior upon which people spend a fair amount of money. Talking care of one’s hair eventuates in psychological rewards. People receive certain things in return, certain values from the experience, which reveals a great deal about the respondent’s values as an individual, and society’s values [5,6]. In a period suffused with various ways to spend money on oneself, and demonstrate one’s values, the world of beauty represents one of the key areas wherein deep investigation is likely to deliver far more than one expects [7]. The value of cosmetics and cosmetic behavior as a lens’ into a person’s emotions and social mind has been a topic having a history of decades, far earlier than the emerging third decade of this century [4,8].
Hair coloring comprises aspects of self-preservation and health, focusing on oneself, and the pursuit of ‘wow,’ the influencing of other, and so forth. The topic of beauty in general, and hair care, including hair coloring, provides an extraordinary opportunity to understand the human mind, in an area where the outer world and the inner world collide, compensate for each other or simply endlessly dance around as the consumer, the individual, the outer-focused and the inner focused halves emerge and recede (Datamonitor, 2005; Euromonitor, 2005, 2013a, 2013b, 2014) [9–13].
The inner experience of beauty has been explored, but not as much as it should be, Any prolonged time exploring the internet will reveal that that the really ‘interesting, meaty stuff’ about one’s experience with beauty may have already been pre-empted those using such personal stories and accounts of experience to gain readers, provide excitement, and sell products, ‘hope in a jar’ [14].
The Emerging Science of Mind Genomics and Its Cartography of the Mind for the Experience of Beauty
Mind Genomics can be considered cartography of everyday experience, exploring and understanding the dimensions, the aspects of experience. In the case of beauty, and specifically hair coloring, Mind Genomics would explore the different topics in the experience. These are the category of questions to be answered, studies best answered by cosmetic science or sociology, or even studies of communication. But then there is the human element, the response of people to these systematically arranged ideas, the use of ‘experiment’ to identify how these elements of beauty ‘function.’ This study of function is done by taking the ‘what’, the questions and answers about the search for beauty, specifically about hair coloring, and asking the respondent to evaluate these different answers using a predefined criterion
As an aside, it is instructive to trace the antecedents of Mind Genomics back to two early ‘schools’ of experimental psychology. The early science of experimental psychology embodied two competing approaches, Structuralism versus Functionalism. Structuralists were interested in the basic dimensions of the mind, classifying perceptions, behaviors, and so forth into different groups. From that classification scheme they believed that the structures would show the nature of what they were studying. The logic was Aristotelian. Classify, organize, and one will learn. The other science of psychology, Functionalism, posited that is that it is the way things operate which inform us about what we are studied. Just knowing the different classifications of perceptions does not tell us how we perceive something (Boring, 1929) [15].
The beginnings of Mind Genomics come both from the experimental psychology of a century ago and from today’s unique confluence of experimental design, internet communication, need for speed, and the incessant push for faster, better, cheaper, and ultimately the push for ‘utterly effective.’ In other words, from the world of business, pushed back to the world of the scientist.
Mind Genomics began with the efforts of statisticians to understand the complexities of the world, but not from the hallowed methods of isolation and study, that gift of the enlightenment, and of the empirical Francis Bacon before the enlightenment. Isolation and study of single phenomena is fine, but in the world of beauty we deal with many variables interacting, swirling about, and creating patterns to be understood, but understood only in a general way. Experimental design simplifies that swirling complex cloud, showing relations between variables which interact to drive a response [16]. The development of Mind Genomics, continued with the pioneering work of Luce & Tukey [17], seeking to put their approach, conjoint measurement on a firm theoretic footing. The late Professor Paul Green of the Wharton School, University of Pennsylvania, and his associates over four decades, brought conjoint measurement into the world of business [18], which in the next evolution spawned Mind Genomics [19–22].
The ‘project’ of Mind Genomics, as explicated here, begins with an aspect of everyday life, proceeds to dissect that aspect into four questions which ‘tell a story,’ generate four answers to each question, and the present combinations of these answers in short, easy-to-read vignettes. Each vignette or combination comprises as many as four answers, or as few as two answers. No question ever contributes more than one answer to a single vignette. Over the course of 24 vignettes, each respondent is exposed to the same answer or message 5x, albeit in the context of other answers. The respondent rates the vignette on an attribute provides. The subsequent statistical analysis ensures that one can read the vignette, the respondent will see combinations of the element, albeit different combinations [23].
The Raw Materials
The raw material for the Mind Genomics study comprises a set of four questions, with each question generating four answers. Both the questions and the answers come from the researcher. There is no ‘fixed set of questions and answers.’ Rather, the questions are guides which tell a story. The answers are the communications, the messages.
The four questions in Table 1 focus on the externals of the coloring process, on what the respondent can be told by a professional. This Mind Genomics study deals with the process, and what is important about the process. It does NOT deal with feelings, except feelings affected by technology.
Table 1. The raw material, comprising the four questions, and the four answers to each question.
|
Question A: Why do you color your hair?
|
A1
|
Coloring hair hides the gray
|
A2
|
Coloring hair gives a person a chance to ‘change’ the look – for fun, temporarily
|
A3
|
Coloring hair is trendy today
|
A4
|
Coloring hair lets the person ‘show off’
|
|
Question B: How do you feel when you change the color?
|
B1
|
Coloring hair instills confidence
|
B2
|
It takes a little time to get accustomed to a new hair color
|
B3
|
To prevent a feeling of insecurity, the coloring has to be ‘just right’
|
B4
|
Coloring makes hair beautiful
|
|
Question C: Do you think the color damages your health, your scalp, or your hair?
|
C1
|
Hair dye can affect health
|
C2
|
Hair dye can damage hair
|
C3
|
Hair dye can damage scalp
|
C4
|
Hair dye damages nothing
|
|
Question D: What do you find more beneficial coloring, treatment or cutting?
|
D1
|
Treatment on hair should be done regularly
|
D2
|
Coloring hair should only be done every other haircut
|
D3
|
It’s important to get hair cut properly
|
D4
|
Hair should be natural… cut when needed, nothing else
|
Finally, the four questions in Table 1 and the 16 answers are set up in a Socratic fashion. That is, the questions are real questions, and the four answers are couched in sentences. The answers or elements suffice to stand by them, and can be mixed and matched in the vignettes, as we will see below. Researchers who do these Mind Genomics studies often volunteer their observation that the method forces them to think in a new way, one which is structured and defined by the question and answer structure. Furthermore, participation in many dozens of these studies over the past years suggests that the hardest part of the exercise comes when the researcher must formulate the four questions so that they ‘make sense’ and present the questions in the proper order to ‘tell a story’. In contrast the answers to the questions, the phrases which will become the building blocks of the vignettes, are far simpler to create. The answers are simple phrases that will be put together in a format, one atop the other, without the effort to make sense. That effort was already expended in the creation of the questions.
Combining the Answers or Elements into Easy-To-Read Vignettes or Test Concepts
If we were to stop at this point with the 16 answers to the four questions, we could subject the 16 answers to a set of rating scales, and feel that we have done adequate research, namely testing the raw materials. There might not be any thought of an experimental design in which we embed combinations of vignettes. The foregoing ‘one-at-a-time’ approach characterizes most of science. That process produces the image of the scientist focusing on one isolated aspect of reality, then studying it with sufficient passion and concentration until the aspect of reality yields ‘its secrets.’
The reality of experience is quite different, especially when we deal with the topic of beauty. Human experience comprises combinations of features, of ideas, of stimuli, as well as expectations, the individual’s history, and the specific nature of the combination. The traditional methods relying upon ‘isolation to understand’ simply cannot work. The researcher must deal with combinations of variables, and from the reaction to these combinations identify what works, and what doesn’t work
Mind Genomics works by using the technique of experimental design, prescribing the systematic combinations of the variables [16]. The combinations are set up so that we begin with a topic, ask four questions, and for each question provide four answers. This was already presented in Table 1. The experimental design specifies 24 combinations, with the property that each unique experimental design is a permutation of a basic, underlying ‘kernel’ design. This property is known as a permuted design [23]. The design ensures that the 16 answers are statistically independent of each other, allowing for regression analysis. The permutation means that no two respondents ever see the same combinations.
The combinations are generated, and put into a vignette, such as the vignette shown in Figure 1. The respondent who evaluates the 24 systematically generated combinations has no idea about an underlying design. The respondent may begin by trying to be ‘consistent,’ but the combinations end up putting a stop to that effort, and in turn the respondent simply assigns an answer in an intuitive way, following what Nobel Economist Daniel Kahneman called System 1 [24].
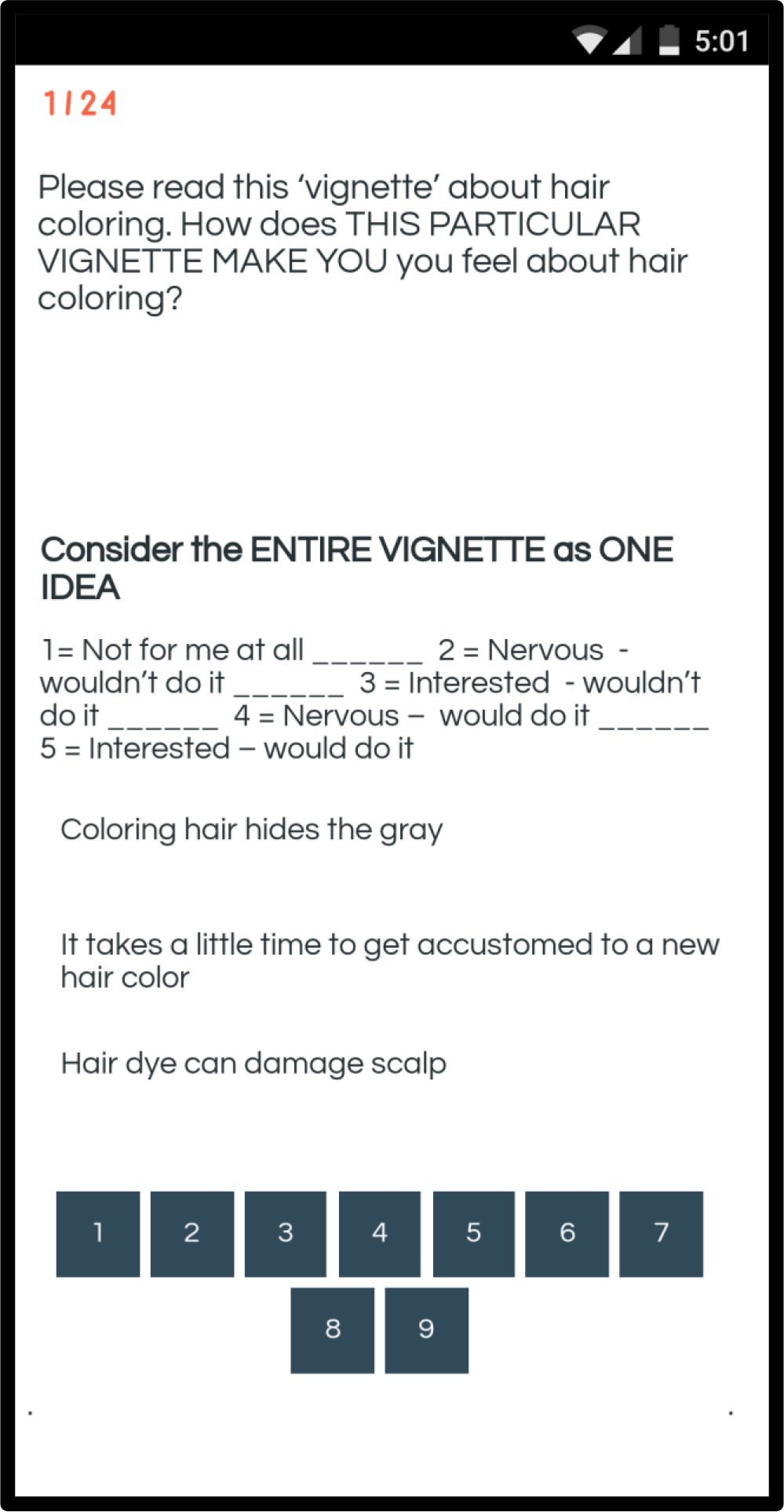
Figure 1. Example of a vignette prescribed by the experimental design and put into a test combination shown on either the screen of a smartphone (shown in Figure 1) or shown on the face of a tablet or PC.
The respondent evaluated each of the 24 vignettes on a single scale, shown in the middle of Figure 1 and in Figure 2. The five scale points deal with two aspects, first an emotional one (nervous versus interested) and second an action one (wouldn’t do it versus would do it). The two questions deal with hair coloring.
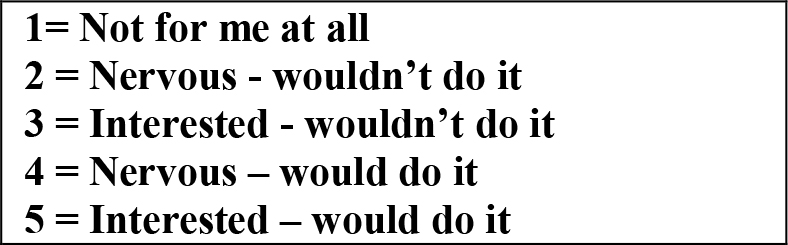
Figure 2. The labelled five-point scale, covering two aspects of the hair coloring experience, emotion and action, respectively.
Creating Binary Variables from the Five Scale Points in the Rating Scale
Mind Genomics studies provide a plethora of data. Each respondent evaluates 24 vignettes, doing so on a five-point scale. The single scale provides two measures; degree of feeling (nervous versus interested) and degree of intent (will not do versus will do.), respectively The Mind Genomics system also records the response time, defined as the number of seconds between the time that the vignette appears on the screen and the selection of the rating
We are fortunate to work with an underlying, ‘permuted’ experimental design, creating a unique set of 24 vignettes for each respondent, and in turn allowing us to discover the linkage between each element and both the 5-point rating and the response time. The algorithm [23] ensures the ability to understand the patterns in in a deep way.
Even before the application of modeling, we do a ‘surface analysis,’ looking at the average rating assigned by the respondents, for the 5-point scale, as well as for transformed aspects of the scale, such as ‘netting’ ‘nervous’ versus ‘enthusiastic.’ The logic of these derived variables will be presented below in the discussion of what the different variables are.
- Rating = Average rating of the labelled 5-point scale. The scale comprises two different underlying scale (feeling, action): 1= Not at all; 2=Nervous/Not Do; 3=Interested/Not Do; 4=Nervous/Do; 5=Interested/Do
- RTseconds – Response time in seconds, or in other words, how quickly the respondent makes his or her decision.
- R1NotAtAll, R2NervousNotDo, R3InterestedNotDo, R4 NervousDo, R5InterestedDo – the five rating scale points converted to binary. That is, when the rating is R1, for example, then the newly created variable, R1NotAtAll, is converted to 100, and the remaining four binary variables (e.g., R2NervousNotDo) are all converted to 0. In effect, only one of the five binary variables created from the one five-point scale can ever have the value 100. The remaining four binary variables created from that one five-point scale must have the value 0, at least for that vignette.
- Net Nervous = sum of both the two binary variables, R2NervousNotDo and R4NervousDo. This is a ‘netted’ variable. We look at the two responses which incorporated ‘nervous’ when either one is selected, we say that the respondent feels nervous We are not interested in whether the respondent will color hair, but only whether the respondent feels nervous.
- Net Do = the sum of two binary variables, R4NervousDo and R5NotNervousDo. The same logic applies. This time the newly created Net Do picks up the response of ‘Do’, whether the respondent feels nervous or not nervous.
Surface Analysis (Average Ratings) Comparing Groups
Table 2 presents the averages of these variables, by Total Panel, Gender, Age Group, Self-defined stage of hair coloring (from the third classification question), and finally the averages when the set of 24 vignettes was divided into four mutually exclusive, complementary groups of six vignettes each.
Table 2. Average ratings by groups of respondents, for the vignettes that they evaluated. The 5-point rating scale was divided into five distinct scale values (R1-R5). Four new variables were also created beyond those five, variables combining scale values of Nervous, of Interested, of No Do, and of Do, respectively. All averages of 40 or more are shown in shaded cells.
Averages
|
5-Point RATING
|
RT SECONDS
|
R1 NOT AT ALL
|
R2 NERVOUS NOT DO
|
|
R3 INTERES NOT DO
|
R4 NERVOUS DO
|
|
R5 INTERESTED DO
|
NERVOUS (NET)
|
INTERESTED (NET)
|
NOT DO (NET)
|
DO (NET)
|
Total
|
3.3
|
3.8
|
16
|
11
|
|
22
|
28
|
|
23
|
39
|
45
|
33
|
51
|
Female
|
3.6
|
4.0
|
6
|
12
|
|
21
|
32
|
|
29
|
44
|
50
|
33
|
61
|
Male
|
3.0
|
3.6
|
26
|
9
|
|
24
|
24
|
|
16
|
33
|
40
|
33
|
41
|
A16–24
|
3.1
|
3.3
|
19
|
15
|
|
21
|
30
|
|
15
|
44
|
37
|
36
|
45
|
A25–44
|
3.6
|
3.5
|
6
|
10
|
|
26
|
32
|
|
25
|
43
|
51
|
37
|
57
|
A45–60
|
3.5
|
3.8
|
10
|
11
|
|
22
|
29
|
|
28
|
41
|
50
|
33
|
57
|
A61+
|
2.4
|
4.7
|
45
|
9
|
|
17
|
18
|
|
12
|
27
|
29
|
26
|
30
|
Coloring Now
|
3.9
|
4.1
|
3
|
9
|
|
19
|
34
|
|
36
|
43
|
54
|
28
|
69
|
Thinking of it
|
3.7
|
3.7
|
3
|
10
|
|
28
|
35
|
|
24
|
45
|
52
|
38
|
59
|
Not Interested
|
2.3
|
3.7
|
44
|
13
|
|
20
|
15
|
|
8
|
28
|
28
|
33
|
24
|
Vignettes 01–06
|
3.3
|
5.8
|
19
|
9
|
|
20
|
29
|
|
23
|
38
|
43
|
29
|
52
|
Vignettes 07–12
|
3.3
|
3.6
|
16
|
12
|
|
23
|
28
|
|
22
|
40
|
45
|
35
|
50
|
Vignettes 13–18
|
3.3
|
3.1
|
16
|
11
|
|
23
|
30
|
|
20
|
41
|
43
|
34
|
50
|
Vignettes 19–24
|
3.4
|
2.8
|
15
|
11
|
|
24
|
26
|
|
25
|
36
|
49
|
34
|
51
|
Arrays of data such as those in Table 2 five a sense of the overall feelings of the respondent groups, as well as uncovering any specific issues or patterns with repeating the study, such as the faster response times for vignettes beyond the first six, or increased level of interest (e.g., the net variable “INTERESTED” is low among the younger respondents (age 16–24), but also low, and surprisingly so, among the older respondents (age 61+.)
We see general patterns from the averages in Table 2, such as the surprisingly resilience of the average rating across the four sets of six vignettes. We also see averages which make sense, such as the lower value for the rating for those respondents not thinking of coloring their hair (average rating = 2.3) versus those respondents already coloring their hair (average rating = 3.9.)
Within Table 2 lie a great deal of so-called ‘insights,’ data organized in such a way as to provide an idea of how people respond to the idea of hair coloring. These data reflect the ‘bread and butter’ information provided by conventional market research. There is a sense of knowing something about people, the notion of ‘insight.’ The reality, however, remains that we know far less than we could know about the respondents than we could know. We know their average responses but cannot yet ‘get into their mind.’ It is that ‘getting into the mind’ to which we now turn in the next sections.
Recoding: Structuring the Mind Genomics Data for Subsequent Analyses
The essence of Mind Genomics is the relation between the response and the specific messages or ‘answers’ to the questions. Mind Genomics system forces decision, but at the same time mirrors reality, by embedding the necessary information in vignettes, wherein the features or answers ‘fight’ with each other. The second aspects of Mind Genomics is the recognition that, for the most part, people are often unaware of ‘why’ they do certain things of an everyday nature. That is, people react quickly, and do not think about what they are doing for much of their behavior. This ‘System 1’ according to Nobel Laureate Daniel Kahneman, is intuitive, ‘at the gut level.’ The research must mirror this quickness [24]. When asked ‘WHY,’ most people can give a reason, but in everyday life the judgments are so rapid that the person is operating on ‘automatic.’
The vignettes were constructed according to an underlying experimental design, complete for each person, but different from person to person in the specific combinations. Mind Genomics then works at the level of the individual respondent, who evaluates the precise but unique set of combinations need to build a model or equation for that person. The metaphor is the MRI, magnetic resonance image, used in medicine to take various snapshots of the individual’s tissue, such as brain, combine these by computer, and create the full 3-dimensional picture of the brain, from which one can detect abnormalities, and so forth.
Each respondent evaluated 24 unique vignettes, created by the design. The result is a database comprising 24 vignette structures x 100 people (our respondents) or a rectangular matrix of 2400 rows, one row per respondent per vignette. In turn, the data begins with 18 columns, the first 16 columns corresponding to one column for each of the ‘elements’ or ‘answers’, our test stimuli, the 17th column for the rating and the 18th column for response time, RT. An additional set of nine columns was then created, five columns for the five responses, RT1 to RT6, and four columns for the newly created “NET” variables (Net Nervous, Net Interested, Net Not Do, Net Do), respectively
The matrix is designed for regression analysis. The independent variables, A1 to D4, are coded to tell the regression program about the status of the elements. When the variable has the value ‘1’ the value denotes the fact that the vignette contained that element or answer. The remaining four independent variables cells in that row will have the value 0 to denote the fact that the vignette did not feature answer or element.
Moving over to the 17th column we see the actual assigned rating which is 1, 2, 3, 4 or 5, depending upon the value chosen. The 18th column shows the response time to one decimal point.
Moving beyond the 18th column we see nine newly created variables. The first five correspond to R1-R5, respectively. When a variable is selected by the respondent, e.g., R2, that newly created variable is assigned the value 100, and the remaining newly created variables (R1, R3, R4, and R5) are assigned the value 0. Finally, the four ‘NET” variables are created by the appropriate addition.
As a matter of course, we add a very small random number to each cell, so that the cell is not 0 or 100, but sum small number a bit larger than 0 or a bit large than 100, respectively. The rationale for adding the small random number is that it prevents the underlying regression program from crashing, were the respondent to never have used 5 or always to have used 5. In the latter situations, there would be no variation in the dependent variable, and in turn the regression analysis could not work. Adding the very small random number barely affects the parameters emerging from OLS (ordinary least-squares) regression model, but avoids the possible crash were the ratings to be all 0 or 100 at the start of the regression.
The data matrix has now been put into a format that the statistical analysis program can ‘process.’ It is an inconvenient but exceptionally widespread, virtually universal reality that much of the effort in the analysis of data to discover patterns is not so much the actual statistical processing, but rather the thinking about how to represent the data in a way that make the data amenable. The restructuring of the data for the regression analysis is as much part of the analysis as are the computations. Indeed, we may say that the up-front thinking IS the analysis, the rest, the computations, simply being the drone work, the busy work. By the time we conceptualize the system as a set of 1’s and 0’s we can be said to have analyzed the data, although not yet to have computed the parameters.
Relating the Key Evaluative Criterion (R5 Interested/Do) To the 16 Answers
We apply OLS (ordinary least-squares) regression to our data. There are research purists who will aver that OLS Regression is not the best approach with data which is ‘binary’ both in the independent variables (the 0/1 representation of the 16 answers as predictors), and dependent variable (0 if the rating is not 5, and 100 if the rating is 5.) Author HRM has consistently use the OLS regression to make the results easy to understand as we see below.
For each dependent variable, and each group of respondents, the OLS regression makes one ‘pass’ through the data. Table 3 shows the results. The table begins with the title of the dependent variable, and the binary expansion. The dependent variable is 5. The data come from the 2400 vignettes evaluated by the total panel. As noted above, the rating of 5 was converted to 100. The complementary ratings of 1–4 were converted to 0.
Table 3. Results from modeling the contribution of the 16 answers to the binary transformed rating of ‘Rating 5’ (Interested and will do.)
|
Dependent variable = binary expansion focusing on R5; INTERESTED and WILL DO
|
Total
|
|
Additive constant
|
25
|
A2
|
Coloring hair gives a person a chance to ‘change’ the look – for fun, temporarily
|
6
|
A1
|
Coloring hair hides the gray
|
5
|
D1
|
Treatment on hair should be done regularly
|
5
|
B4
|
Coloring makes hair beautiful
|
4
|
D3
|
It’s important to get hair cut properly
|
4
|
B2
|
It takes a little time to get accustomed to a new hair color
|
3
|
D4
|
Hair should be natural… cut when needed, nothing else
|
2
|
D2
|
Coloring hair should only be done every other haircut
|
1
|
A3
|
Coloring hair is trendy today
|
0
|
A4
|
Coloring hair lets the person ‘show off’
|
-1
|
B1
|
Coloring hair instills confidence
|
-1
|
B3
|
To prevent a feeling of insecurity, the coloring has to be ‘just right’
|
-2
|
C4
|
Hair dye damages nothing
|
-2
|
C2
|
Hair dye can damage hair
|
-11
|
C1
|
Hair dye can affect health
|
-12
|
C3
|
Hair dye can damage scalp
|
-13
|
The regression was done on the entire set of 2400 observations, the so-called ‘Total Panel.’ We proceed to the Additive Constant, which is the estimated percent of responses of exactly ‘5’ in the absence of any elements. The additive constant is a purely estimated parameter, since all vignettes by design comprised 2–4 answers or elements. Nonetheless, we compute the regression with the additive constant.
We treat the additive constant as a baseline, the measure of tendency to be interested in hair coloring and ready to color one’s hair without any additional information. In other words, we assume an underlying tendency to respond ‘Interested and Will Do’ for a given topic, just based upon the name of the topic, but without any other specifics. The OLS regression takes this tendency into account, showing it as the ‘additive constant.’ The additive constant for our study of 100 respondents and hair coloring achieves a value of 25 when the dependent variable is ‘R5,’ after R5 is converted into a binary value which takes on the value 0 (R5<>5) or takes on the value 100 (R5=5).
The low additive constant of 25 means in ‘technical talk’ that in only 25% of the time may we expect to see a rating of 5 in a vignette without elements. Respondents are simply not interested in the notion of hair coloring as a topic. They do color their hair, but they would not say ‘interested and will do’ as a basic matter of course. It is the elements, the answers, which must drive the response.
Table 3 also shows us that for the most part the coefficients are low, the highest coefficients achieved by A2 and A2 (changing the look; hiding the gray.) These low coefficients should alert us to the possibility that either people are not particularly interested in the notion of hair coloring, even at the level of specifics, or more likely, there are different mind-sets which cancel each other.
The negatives push people away from the positive of interested/will do. We don’t know whether these are truly negative. They are simply non-positive. We simply know from our recoding that they are not positive, not 5. The negatives are the answers which talk about the issues and problems. We should not be surprised; the problems are the issues of dye, injuring hair, health, scalp, respectively. Even saying that there is no damage is a negative, perhaps because no damage, no untoward accident, is not a positive.
Respondent Data Are Reliable – Evidence From Looking At The Starting Versus The Ending Test Vignettes
The criticism is often raised that respondents cannot actually do the task. Despite the emergence of clear patterns, there are purists who believe that the five-minute experiment with 24 vignettes is simply too fatiguing, and that what one sees is the analysis of rather meaningless data, assigned by respondents who are tired, bored, and angry.
To assess reliability, we divide the vignettes into those appearing in positions 1–6, 7–12, 13–18 and 19–24. We do not look at the respondents, but simply create four databases, and do the regression modeling for each of the four sets of position. We do so by OLS, ordinary least-squares regression, and force the equation through the origin by not having an additive constant, a slight departure from the OLS regression for the ratings, but one which allows us the ability to compare coefficients without the interference of the additive constant. OLS returns with estimates of the 16 coefficients. Figure 3 shows the scatterplot, the ordinate showing the coefficients emerging when we look only at the set of ratings obtained from responses to vignettes 19–24, the last six vignettes tested. The abscissa shows the coefficients emerging when we look only at the set of ratings obtained from responses to vignettes 01–06, the first six vignettes tested. They are quite similar, overall, albeit with some natural noise to destroy the otherwise very high correlation.
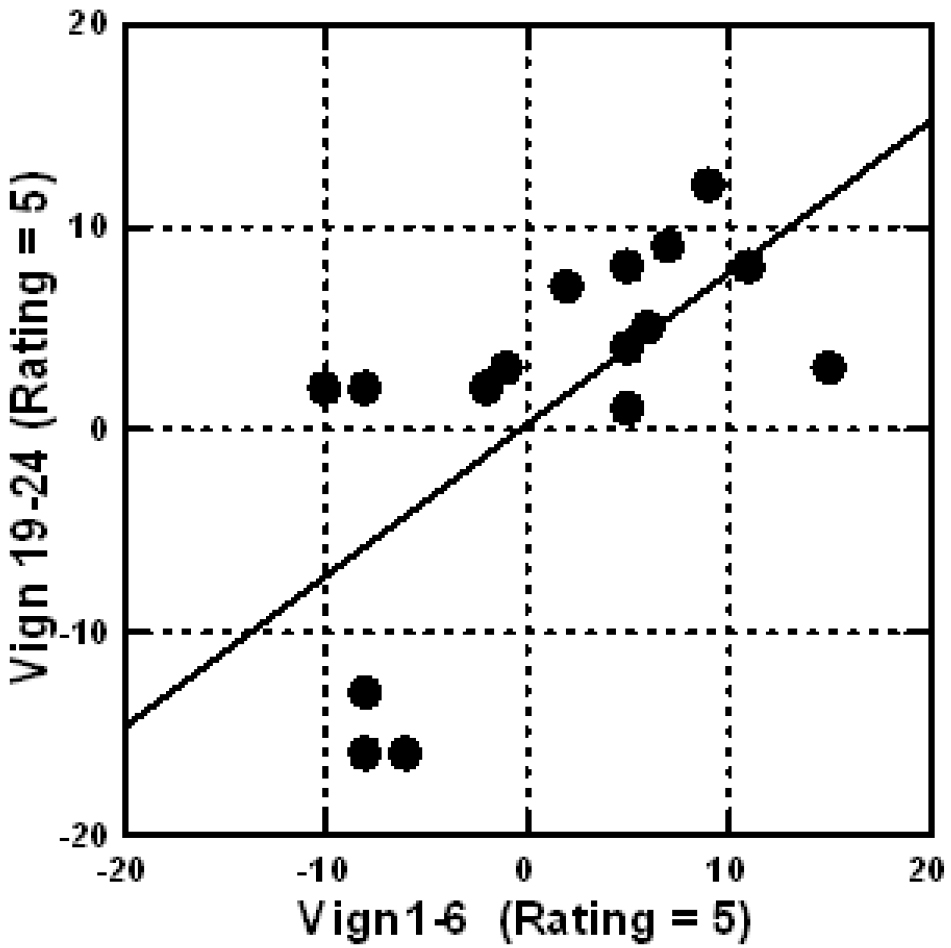
Figure 3. Scatterplot showing the 16 coefficients estimated from vignettes at the first part of the experiment (vignettes 01–06) versus the vignettes from the last part of the experiments (vignettes 19–24), The 16 coefficients were estimated after the binary transformation of Rating 5 (interested/will do).
A parenthetical note is appropriate here: The negative reactions to the Mind Genomics effort is most often heard from professionals who participate in a study, only to return with a host of negatives ranging from ‘I didn’t know what I was doing, I just guessed’ to ‘The graphics are so 20th century, and fail to make the experience engaging, in turn failing to make the experience even valid. The pattern of coefficients presented here refutes the accusations, almost always from professionals, almost never from ‘real people.’
Differences in the Performance of Elements among Complementary Subgroups
The poor showing of most elements or answers in Table 3, presenting the Total Panel’ may surprise the reader, since hair coloring is a very popular topic. The surprise only comes when we realize that for virtually everything, there are at least two or more points of view, stances when ‘human judge.’ Our quotidian existence is replete with aphorism driving home the individuality of judgment, the tacit recognition that people differ. It’s not only the recognition that they exist but accepting and enshrining those differences as a mainstay of an enlightened point of view.
The dramatic nature of the group difference can be seen in Table 4. The table shows many more elements performing better, at least at the level of statistical significance. The elements show those elements which perform well in at least one group. The operational definition of ‘perform well’ is a coefficient greater than 7.51, which is both statistically significant and corresponds to elements which have been observed to represent ‘effective’ in the outside world of daily experience. Table 4 suggests that looking at key subgroups increases the likelihood of at least one element performing strongly.
Table 4. Coefficients from the model for complementary subgroups. The dependent variable is the binary transformation of Rating5 (Interested / Will Do).
|
|
Gender
|
Age
|
Current status re hair coloring
|
|
Dependent variable = binary transformation of Rating R5
(Interested, Will Do).
|
Male
|
Female
|
A16–24x
|
A25–44x
|
A45–60x
|
A61Plus
|
Coloring Now
|
Thinking about it
|
Not Interested
|
|
CONSTANT
|
22
|
28
|
12
|
31
|
28
|
13
|
38
|
24
|
15
|
A1
|
Coloring hair hides the gray
|
3
|
8
|
4
|
3
|
7
|
7
|
7
|
8
|
1
|
A2
|
Coloring hair gives a person a chance to ‘change’ the look – for fun, temporarily
|
7
|
6
|
16
|
8
|
3
|
3
|
3
|
12
|
4
|
A3
|
Coloring hair is trendy today
|
0
|
1
|
10
|
3
|
-1
|
-5
|
-1
|
1
|
0
|
B2
|
It takes a little time to get accustomed to a new hair color
|
-1
|
7
|
-12
|
8
|
3
|
-2
|
3
|
5
|
1
|
C4
|
Hair dye damages nothing
|
-2
|
-3
|
8
|
-7
|
-2
|
1
|
-3
|
4
|
-8
|
D1
|
Treatment on hair should be done regularly
|
4
|
6
|
4
|
1
|
9
|
6
|
9
|
5
|
1
|
D2
|
Coloring hair should only be done every other haircut
|
1
|
2
|
9
|
1
|
1
|
0
|
3
|
-1
|
1
|
D3
|
It’s important to get hair cut properly
|
5
|
4
|
0
|
5
|
4
|
8
|
5
|
3
|
3
|
D4
|
Hair should be natural… cut when needed, nothing else
|
3
|
1
|
2
|
0
|
0
|
9
|
1
|
2
|
2
|
The data in Table 4 suggest that many of the elements simply ‘do not work.’ They only show a slight- increase in the coefficients. Two elements, however, performed well, with coefficients about 10 in at least one subgroup.
Coloring hair gives a person a chance to ‘change’ the look – for fun, temporarily
Coloring hair is trendy today
Creating Mind-Sets as a Way to Dive Deeply Into the Cosmetic Mind
Mind Genomics provides a tool by which one can create divisions, groups, among respondents based on how the people think, not who they are, not what they believe. Traditional research with customers often labels this approach psychographic segmentation, in which one divides people by what they think (general attitudes; Wells, 2011 [25]) versus dividing people by WHO THEY ARE OR WHAT THEY DO. The technique used is clustering, a well-established approach in statistics to explore data with the hope of finding groups of similar ‘objects’, similarity based upon the pattern of properties of those objects [26].
Mind Genomics carries psychographic segmentation one step further, beyond attitudes and beliefs, and into the response pattern to messages crafted to be specific for a topic, and thus precisely appropriate for that topic. A typical psychographic segmentation involving hair coloring would incorporate the entire gamut of cosmetics, and beauty-seeking behavior. The goal in Mind Genomics is to work at the very ‘micro-level,’ with language most closely associated with the topic. Thus, the data emerging from the clustering or segmentation may be said to be laser-focused on the topic of hair coloring, and perhaps even more focused on the reasons for coloring hair versus not coloring hair. Discoveries from the clustering and segmentation are thus both limited but often extremely novel, often ready to turn into both scientific insights and business actions.
The clustering performed on the data for this study looked at the respondents based upon the coefficients emerging from the regression analysis, wherein the dependent variable is R5 (Interested / Will Do). Each respondent generated an individual model. The 16 coefficients were used as the basis for clustering the respondents twice, first into two mind-sets or clusters, second into three mind-sets or clusters. The two-segment solution could not be interpreted. A cluster or mind-set comprised different elements which could not be the basis of a simple ‘description.’ In contrast, dividing the respondents into three clusters or segments made it simple to assign names. We simply looked at the elements which scored the highest in each cluster. Table 5 presents the three mind-sets.
Table 5. Three segments for hair coloring emerging from segmenting the respondents by the pattern of coefficients for R5, Interested/Do.
|
|
MS1
|
MS2
|
MS3
|
|
Dependent variable = binary transformation of Rating R5 (Interested, Will Do).
|
Follow prescription
|
Coloring is a personal expression
|
Focus on self-care
|
|
Additive constant
|
27
|
21
|
25
|
|
Mind-Set 1 – Follow the prescriptions of others
|
|
|
|
D1
|
Treatment on hair should be done regularly
|
8
|
4
|
4
|
D4
|
Hair should be natural… cut when needed, nothing else
|
7
|
-3
|
2
|
|
Mind-Set 2 – Coloring is self-expression
|
|
|
|
A2
|
Coloring hair gives a person a chance to ‘change’ the look – for fun, temporarily
|
6
|
13
|
-1
|
A1
|
Coloring hair hides the gray
|
4
|
10
|
2
|
A3
|
Coloring hair is trendy today
|
-1
|
7
|
-6
|
|
Mind-Set 3 – Self Care
|
|
|
|
D3
|
It’s important to get hair cut properly
|
4
|
1
|
8
|
|
Does not strongly appeal to any mind-set
|
|
|
|
B4
|
Coloring makes hair beautiful
|
4
|
3
|
4
|
D2
|
Coloring hair should only be done every other haircut
|
2
|
0
|
3
|
B2
|
It takes a little time to get accustomed to a new hair color
|
6
|
2
|
1
|
A4
|
Coloring hair lets the person ‘show off’
|
-4
|
3
|
-3
|
C4
|
Hair dye damages nothing
|
-4
|
2
|
-3
|
B1
|
Coloring hair instills confidence
|
1
|
3
|
-5
|
B3
|
To prevent a feeling of insecurity, the coloring has to be ‘just right’
|
0
|
0
|
-6
|
C2
|
Hair dye can damage hair
|
-16
|
-5
|
-11
|
C1
|
Hair dye can affect health
|
-17
|
-5
|
-12
|
C3
|
Hair dye can damage scalp
|
-16
|
-4
|
-18
|
Finding Respondents in the Population – the PVI (Personal Viewpoint Identifier)
The premise of Mind Genomics is that the mind-sets exist but need not be correlated with WHO the respondents are, or even what, in general, the respondents BELIEVE. (see Table 6). Yet, these mind-sets have a very important role to play, both for knowledge and for application. When one works with these mind-sets, it becomes possible to explore more deeply the roots and foundations, if any, undergirding one’s membership in a mind-set.
Table 6. Cross tabulation of mind-set membership by self-profiled group membership. Numbers in the body of the table represent numbers of respondents out of the total group of 100 respondents.
|
MS1 – Follow prescription
|
MS2 – Coloring a personal expression
|
MS3 – Focus on self-care
|
Total
|
Total
|
37
|
33
|
30
|
100
|
Mind-Sets by Gender
|
|
|
|
|
Male
|
16
|
14
|
20
|
50
|
Female
|
21
|
19
|
10
|
50
|
Mind-Sets by Age
|
|
|
|
|
A16–24
|
4
|
2
|
4
|
10
|
A25–39
|
15
|
10
|
8
|
33
|
A40–55
|
9
|
11
|
11
|
31
|
A56+
|
9
|
10
|
7
|
26
|
Mind-Sets by Hair coloring behavior
|
|
|
|
|
Coloring Now
|
10
|
15
|
7
|
32
|
Thinking About It
|
14
|
9
|
12
|
35
|
Not Interested
|
5
|
7
|
11
|
23
|
No Answer
|
8
|
2
|
0
|
10
|
If, as continuing research suggests, there are no general co-variations of membership in a mind-set, especially age and gender, as well perhaps in one’s behavior in the category (question 3), then the next thing is to create a tool by which to assign new people to one of three mind-sets. Author Gere has created the PVI, the personal viewpoint identifier, using as a base the pattern of responses to different and jet differentiating questions. Figure 4 shows the six questions constituting the PVI. The pattern of answers to the six questions are used to assign a new person to one of the three mindsets, with the feedback shown in Figure 5.
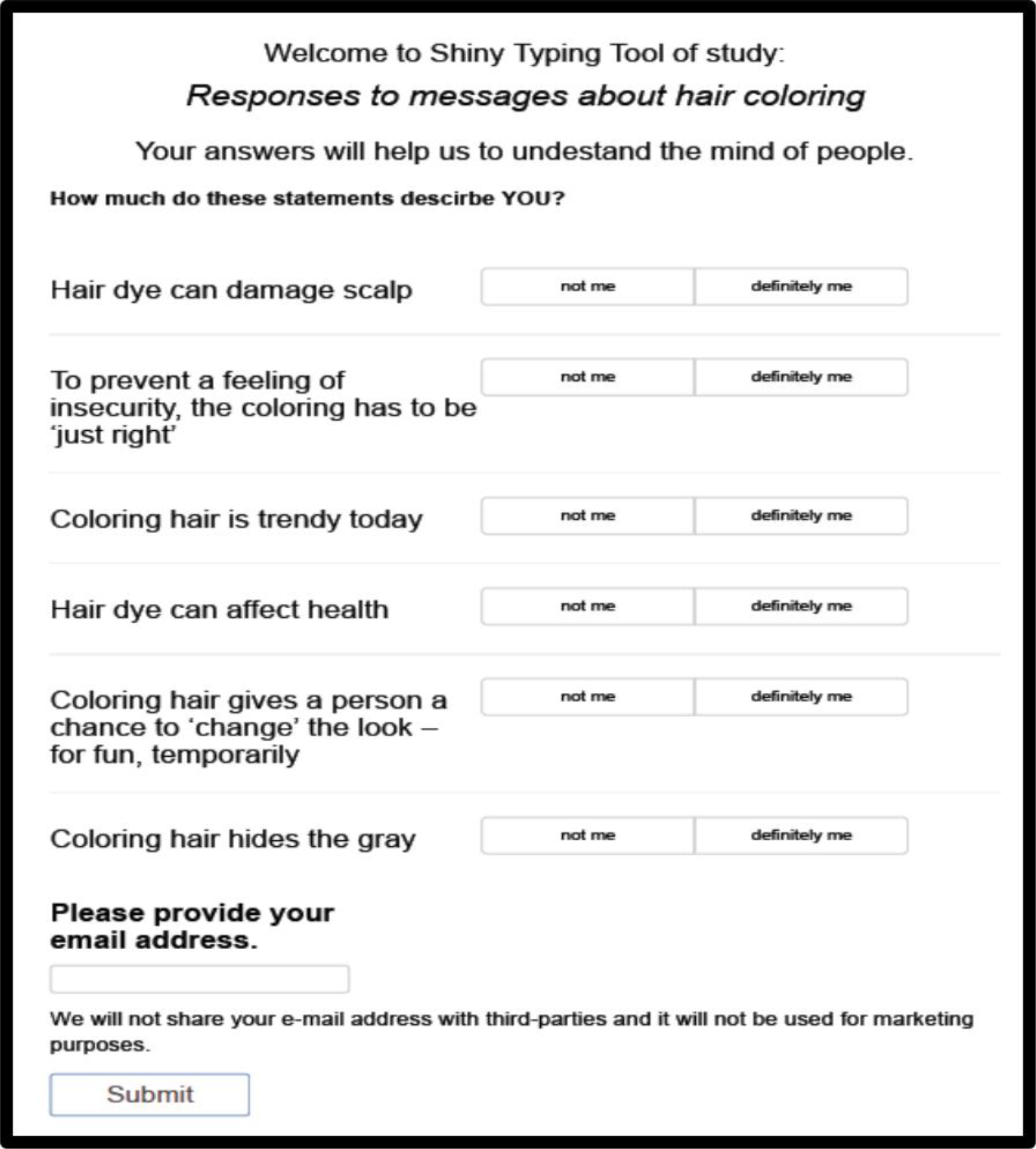
Figure 4. The PVI (personal viewpoint identifier) created from the hair coloring study to assign new people to one of the three mind-sets.
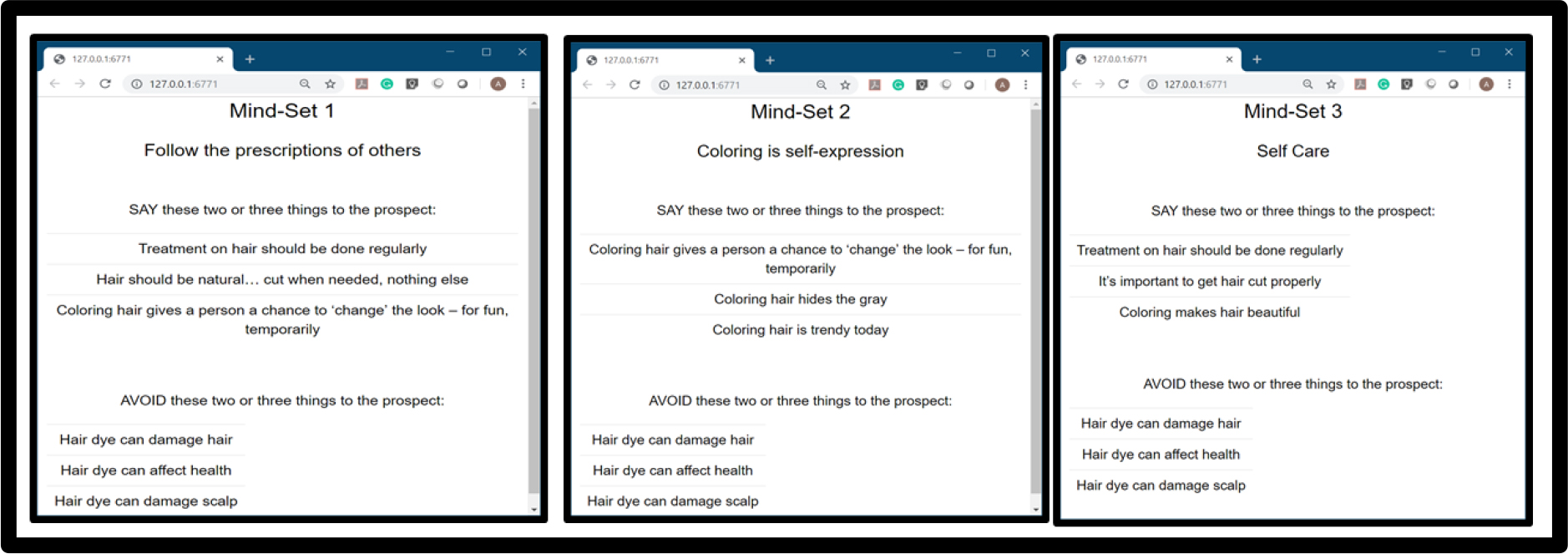
Figure 5. Feedback screens from the PVI. The feedback can go to the respondent, the hair salon, or to guide the messaging by merchants who advertise, either at point of sale in stores or on the Internet in e-commerce.
The PVI as shown in Figures 4 and 5 enable the use of the knowledge for either business applications or for continuing social research. One need only deploy the PVI at a salon or on the web, in order to understand the mind of the person, and relate mind-set membership for hair coloring to different forms of knowledge such as WHO and WHAT the person is an does, in the three mind-sets. Or the researcher can move more deeply into understanding how mind-set covaries with shopping behaviors.
At the level of application, one need only realize the business power of knowing the mind-set of a person with respect to hair coloring. Such knowledge, perhaps obtained quickly on the internet or in person, can be used to drive marketing efforts. The PVI enables the sales messages to be those which are similar in content and tonality to the messages which would appeal to the mind-set to which the prospective customer appears to belong.
Response Time
Our final topic concerns the deconstruction of the messages into those which engage, based upon long response times, versus those messages which do not appear to engage, based upon short response time. It is important to note that response time IS NOT ACCEPTANCE. Rather, response time is an empirical measure of the expected number of seconds (to the nearest tenth) that one appears to ‘read’ and thus process the element.
It is impossible to measure the response time to the individual answers, but it is very straightforward to measure the response time to the vignette and then use OLS regression to deconstruct the response time into the contribution of the individual elements or answers. The regression model is simple, similar to the model used for R5, except that the dependent variable is the response time, and the model has no additive constant. That is, the ingoing assumption is that in the absence of phrases, no one reads, and therefore there is no processing time.
Tables 7A and 7B show the estimated response times to the different elements or answers, this time by total panel, by gender, age, thoughts about coloring hair, mind-sets, and finally starting versus ending vignettes. To make the table easier to read, those response times of 1.5 seconds or longer are shown in shade and in bold face. One can look across the table or downward, looking across a person, to discover what engages the respondent.
Mind Genomics works with ‘cognitively meaningful’ stimuli. That is, the elements have real meaning in the world, and thus our analysis to find a strong performer and interpret why, is made much easier. We are struck with a few observations, mainly qualitative ones when we look at the patterns of shaded cells, the intersection of an element or answer (row) and a subgroup (column),
- We begin with the fact that the nature of the information is the same, messages about hair color. The information differs both in the morphology (length of the answer in words and letters), and in meaning (what the answer conveys.)
- There appears to be a greater similarity of response times within a column (same group), rather than within a row (same answer or message.) This is a qualitative observation only. The implication for subsequent research is that the response time may be hinting at differences in the way people process nature.
- Some questions, such as Question A ‘Why do you color your hair?’ show relatively short response times associated with their answers. In contrast, other questions, such as Question D ‘What do you find more beneficial; coloring, treatment or cutting?’ show long response times associated with their answers. The differences, again, are qualitative, and should be considered against the background of dramatic variation both in the response times of different groups, and the response times to different answers.
Discussion and Conclusion
Relevance of Mind Genomics Knowledge to Understanding People in Society: The experiment on attitudes or mind-sets about hair coloring suggests that science need not be relegated to large-scale studies, the norm today in the hard sciences, but increasingly so in the psychological and social sciences. Today’s attitudes towards science stress the deadly combination of doing research in an acceptable way to the academic community, often picking topics to validate or disprove small points in a larger theory, while working with surveys which fail to give a sense of the immediacy of the experience.
By couching the test stimuli in the language of the everyday, by making studies possible with as few as 50 respondents, and by allowing a research project to take perhaps no more than a few hours, Mind Genomics presents the scientific and business community with a new tool, one to understand people in society. One may think of Mind Genomics as a combination of quantitative ethnography (albeit ethnography of the mind’s interaction with the world), and a Technical Aid to Creative Thought, a term coined by Harvard computer professor, Anthony Gervin Oettinger, more than 55 years ago,
Applications of the Mind-Sets: Once the mind-sets are revealed, the reactions are quite predictable. The first reaction is a delighted wonderment. The reaction cannot be controlled nor suppressed, at least for long. There is an innate, almost child-like delight in the discovery of something new. The second reaction, however, is perplexity. The individual or group of individuals encountering the mind-sets for the first time begins to wonder ‘what do we do with this information.’ The mind-sets are too compelling to be ignored in the way many other ‘factoids’ emerging from an experiment are ignored.
Table 7A. Response time as a function of element, showing complementary subgroups of WHO respondents ARE.
|
|
|
Gender
|
Age
|
|
Response Time – Total panel and ages
|
Total
|
Male
|
Female
|
A16–24
|
A25–44x
|
A55–60x
|
A61+
|
|
Question A: Why do you color your hair?
|
|
|
|
|
|
|
|
A1
|
Coloring hair hides the gray
|
0.6
|
0.8
|
0.4
|
0.7
|
0.3
|
0.6
|
1.3
|
A2
|
Coloring hair gives a person a chance to ‘change’ the look – for fun, temporarily
|
0.8
|
0.7
|
0.9
|
0.3
|
0.6
|
1.1
|
1.0
|
A3
|
Coloring hair is trendy today
|
0.7
|
0.7
|
0.6
|
-0.2
|
0.4
|
0.8
|
1.1
|
A4
|
Coloring hair lets the person ‘show off’
|
1.2
|
1.2
|
1.1
|
1.3
|
1.1
|
1.2
|
1.4
|
|
Question B: How do you feel when you change the color?
|
|
|
|
|
|
|
|
B1
|
Coloring hair instills confidence
|
1.1
|
1.0
|
1.2
|
2.0
|
1.1
|
1.1
|
0.8
|
B2
|
It takes a little time to get accustomed to a new hair color
|
1.4
|
1.4
|
1.3
|
1.2
|
1.2
|
1.6
|
1.2
|
B3
|
To prevent a feeling of insecurity, the coloring has to be ‘just right’
|
1.5
|
1.4
|
1.6
|
1.7
|
1.1
|
1.7
|
1.9
|
B4
|
Coloring makes hair beautiful
|
1.2
|
1.3
|
1.1
|
2.1
|
1.0
|
1.4
|
1.2
|
|
Question C: Do you think the color damages your health, your scalp, or your hair?
|
|
|
|
|
|
|
|
C1
|
Hair dye can affect health
|
1.1
|
1.0
|
1.3
|
1.7
|
1.1
|
0.9
|
1.2
|
C2
|
Hair dye can damage hair
|
1.2
|
0.9
|
1.5
|
1.5
|
1.4
|
0.9
|
1.3
|
C3
|
Hair dye can damage scalp
|
1.1
|
0.9
|
1.3
|
1.5
|
1.0
|
1.0
|
1.3
|
C4
|
Hair dye damages nothing
|
1.1
|
1.0
|
1.2
|
1.4
|
0.9
|
1.1
|
1.3
|
|
Question D: What do you find more beneficial: coloring, treatment or cutting?
|
|
|
|
|
|
|
|
D1
|
Treatment on hair should be done regularly
|
1.0
|
1.0
|
1.0
|
-0.1
|
1.1
|
1.0
|
1.4
|
D2
|
Coloring hair should only be done every other haircut
|
1.1
|
1.0
|
1.3
|
-0.7
|
1.1
|
1.4
|
1.5
|
D3
|
It’s important to get hair cut properly
|
1.2
|
1.1
|
1.4
|
0.7
|
1.3
|
1.1
|
1.6
|
D4
|
Hair should be natural… cut when needed, nothing else
|
1.5
|
1.4
|
1.6
|
0.4
|
1.9
|
1.0
|
2.2
|
The applications of the mind-sets range from understanding to sales, from science to application. The key to application is recognizing that people are different in the way they think about the same topic, knowing the specific ways that they think for a topic (the mind-set segmentation), and then having a tool to assign a new person to a mind-sets (the above-mentioned PVI, personal viewpoint identifier.)
The applications abound:
- Specific knowledge: create an entire science of a topic of the everyday (e.g., the science of the beauty experience),
- Co-variation of mind-sets with external behaviors: understand the nature of how people in different mind-sets of the same topic behave in their actual choices,
- Persuade: Assign a new person (sales prospect) to a mind-type in a short interaction, and presenting that person with the appropriate sales material
Prospects for databases and understanding interactions between WHO, WHAT, and the Mind
The ability to create a database about a specific topic with as few as 50–100 respondents, and then create the PVI (personal viewpoint identifier) means that it becomes possible to profoundly understand a small and clearly defined topic of experience, and then expand that topic through the PVI. The analogy is inexpensively created color science, and a colorimeter to measure the color for millions upon millions of objects.
The same thinking can be applied to Mind Genomics. We can take the topic of beauty care, divide it into 20 or even more topics, dimensionalize each topic (four questions, four answers per question), and run the study with 100 people. The emergent mind-sets can then be captured for new people using the PVI. With 20 studies, we have a grand PVI of 20 topics, each topic comprising 6 questions. It is only a matter of motivating a respondent to participate, answering the 120-question PVI, perhaps over a period of two or three sessions. The data, along with the respondents’ age, gender, and other details of a standard self-profiling questionnaire, provides the necessary information to ‘mind-type’ the world.
The analysis then proceeds in a very simple fashion. The PVI exercise has generated the profile of a person’s mind with respect to beauty care. We need only relate the ‘sequenced profile’ of a person’s 20 mind-sets in beauty care to other measures of the person, whether these be WHO the person is, or WHAT the person does.
Table 7B. Response time as a function of element, showing complementary subgroups what the respondents think about hair coloring, different mind-sets, and a comparison response time at the start of the experiment (vignettes 1–6) and at the end of the experiment (vignettes 19–24).
|
|
Q3: Coloring hair behavior
|
Mind-Sets
|
Order of testing
|
|
|
Coloring my hair now
|
Thinking about it
|
Not interested
|
Follow the prescriptions
of others
|
Coloring –
A personal expression
|
Focus on self-care
|
Vignettes 01–06
|
Vignettes 19–24
|
|
Question A: Why do you color your hair?
|
|
|
|
|
|
|
|
|
A1
|
Coloring hair hides the gray
|
0.2
|
0.7
|
0.9
|
0.5
|
0.7
|
0.7
|
1.0
|
0.3
|
A2
|
Coloring hair gives a person a chance to ‘change’ the look – for fun, temporarily
|
1.2
|
0.9
|
0.5
|
1.1
|
0.8
|
0.6
|
1.3
|
0.6
|
A3
|
Coloring hair is trendy today
|
0.8
|
0.6
|
0.5
|
0.6
|
0.7
|
0.7
|
1.4
|
0.4
|
A4
|
Coloring hair lets the person ‘show off’
|
1.4
|
1.3
|
0.9
|
1.1
|
1.3
|
1.2
|
2.3
|
0.9
|
|
Question B: How do you feel when you change the color?
|
|
|
|
|
|
|
|
|
B1
|
Coloring hair instills confidence
|
1.1
|
1.2
|
0.9
|
1.3
|
1.0
|
1.0
|
1.5
|
1.0
|
B2
|
It takes a little time to get accustomed to a new hair color
|
1.4
|
1.1
|
1.6
|
1.6
|
1.3
|
1.1
|
2.3
|
1.2
|
B3
|
To prevent a feeling of insecurity, the coloring has to be ‘just right’
|
1.8
|
1.1
|
1.6
|
1.6
|
1.5
|
1.3
|
2.0
|
1.2
|
B4
|
Coloring makes hair beautiful
|
1.2
|
1.0
|
1.4
|
1.4
|
1.2
|
1.0
|
1.9
|
1.0
|
|
Question C: Do you think the color damages your health, your scalp, or your hair?
|
|
|
|
|
|
|
|
|
C1
|
Hair dye can affect health
|
1.4
|
1.0
|
1.0
|
1.4
|
1.0
|
1.0
|
1.8
|
0.8
|
C2
|
Hair dye can damage hair
|
1.3
|
1.3
|
1.0
|
1.4
|
1.3
|
0.9
|
2.0
|
0.6
|
C3
|
Hair dye can damage scalp
|
1.2
|
1.2
|
0.9
|
1.2
|
1.3
|
0.7
|
1.8
|
0.0
|
C4
|
Hair dye damages nothing
|
1.3
|
0.8
|
1.1
|
1.2
|
1.1
|
1.0
|
1.5
|
1.1
|
|
Question D: What do you find more beneficial coloring, treatment or cutting?
|
|
|
|
|
|
|
|
|
D1
|
Treatment on hair should be done regularly
|
1.0
|
0.9
|
1.1
|
0.9
|
0.9
|
1.2
|
0.8
|
1.2
|
D2
|
Coloring hair should only be done every other haircut
|
1.3
|
1.1
|
1.0
|
1.2
|
0.8
|
1.3
|
1.4
|
0.9
|
D3
|
It’s important to get hair cut properly
|
1.3
|
1.2
|
1.3
|
1.1
|
1.1
|
1.6
|
1.6
|
1.0
|
D4
|
Hair should be natural… cut when needed, nothing else
|
1.3
|
1.8
|
1.3
|
1.9
|
0.7
|
1.8
|
2.1
|
1.0
|
Acknowledgement
Attila Gere thanks the support of Premium Postdoctoral Research Program of the Hungarian Academy of Sciences.
References
- Trueb RM (2005) Understanding Hair Biology European Hair Research Society, 11th Meeting, Zurich, July 2005: Abstracts and Selected Reviews.
- Bloch PH (1993) Involvement with adornment as leisure behavior: an exploratory study. Journal of Leisure Research 25: 245–262.
- Cash TF (1987) The psychology of cosmetics: A review of the scientific literature. Social and Behavioral Sciences Documents 17: MS No2800.
- Graham JA, Jouhar AJ (1981) The effects of cosmetics on person perception. International Journal of Cosmetic Science 3: 199–210.
- Moore L (2005) The Personal Beauty Care Market Outlook: NPD and Consumer Trends in Haircare and Skincare. Business Insights.
- Zhao X (2014) Salon hair coloring in the United States: A consumer perceived value analysis of Gen Y consumers. International Journal of Arts and Commerce 3: 105–115.
- Mitchell VW (2003) Generation Y female consumer decision-making styles. International Journal of Retail & Distribution Management 31: 95–106.
- Souiden N, Diagne M (2009) Canadian and French men’s consumption of cosmetics: a comparison of their attitudes and motivations. Journal of Consumer Marketing 26: 97 – 109.
- Datamonitor (2004) Haircare in the United States. Datamonitor USA, Reference Code: 0072–2242 Retrieved September 2, 2005 from http://www.datamonitor.com
- Euromonitor (2014) The Divergent Worlds of Hair Care: Global Strategies for Growth Euromonitor. Passport February, Retrieved April 16, 2014 from http://www.euromonitor.com
- Euromonitor (2005) The World Market for Cosmetics and Toiletries. Euromonitor International Retrieved October 1, 2005 from http://libproxy.uncg.edu:2798/Reports.aspx Euromonitor
- Euromonitor (2013a) Rethinking Beauty: Exploring New Growth Models Euromonitor Passport October, Retrieved May 12th, 2014 from http://www.euromonitor.com
- Euromonitor (2013b) Hair Care in China. Euromonitor Passport April, Retrieved May 12th, 2014 from http://www.euromonitor.com Euromonitor (2013c) Hair Care in the US Euromonitor Retrieved May 12th, 2014 from http://www.euromonitor.com
- Bloch PH, Richins ML (1992) You look “Marvelous”: the pursuit of beauty and the marketing concept Psychology and Marketing 9: 3–15.
- Boring EG (1929) A history of experimental psychology Prentice-Hall.
- Box GE, Hunter WG, Hunter JS (1978) Statistics for experimenters, New York, John Wiley
- Luce RD, Tukey JW (1964) Simultaneous conjoint measurement: A new type of fundamental measurement. Journal of mathematical psychology 1: 1–27.
- Green PE, Srinivasan V (1990) Conjoint analysis in marketing: new developments with implications for research and practice. The journal of marketing 54: 3–19.
- Moskowitz HR (2012) ‘Mind genomics’: The experimental inductive science of the ordinary, and its application to aspects of food and feeding. Physiology & Behavior 107: 606–613.
- Moskowitz HR and Gofman A (2007) Selling blue elephants: How to make great products that people want before they even know they want them. Pearson Education.
- Moskowitz HR, Gofman A, Beckley J and Ashman H (2006) Founding a new science: Mind genomics. Journal of sensory studies 21: 266–307.
- Moskowitz HR, Gofman A, Itty B, Katz R, Manchaiah M, Ma Z (2001) Rapid, inexpensive, actionable concept generation and optimization: the use and promise of self-authoring conjoint analysis for the food service industry. Food Service Technology 1: 149–167.
- Gofman A, Moskowitz H (2010) Isomorphic permuted experimental designs and their application in conjoint analysis. Journal of Sensory Studies 25: 127–145.
- Kahneman D, Egan P (2011) Thinking, fast and slow. New York: Farrar, Straus and Giroux.
- Wells WD (2011) Life style and Psychographics, Chapter 13: Life Style and Psychographics: Definitions, Uses, and Problems. Marketing Classics Press.
- Dubes R, Jain AK (1980) Clustering methodologies in exploratory data analysis. Advances in Computers 19: 113–238.